Depression detection via conversation turn classification
Multimedia Tools and Applications(2023)
摘要
The fast pace of modern life caused people to experience more pressure from their surrounding environments. As a result, depression has emerged as one of the most common diseases. To detect depression, psychiatrists need to interview patients to understand their mental health condition. However, it may not fully reflect the condition of the patient since some people cannot adequately express their feelings. As an alternative, researchers work on automated depression detection. Our work aims to improve the detection capability by extracting knowledge from psychiatric consultation. Previous studies classified the questions and answers in the interview into categories unrelated directly to depression. In this paper, we implement a novel categorization based on the Patient Health Questionnaire-8 (PHQ-8). The PHQ-8 is commonly used as a screening tool to identify the severity of depression, making it more reliable for detecting depression. Transcribed data is processed using contextual word embedding, BERT, to learn semantic information. In the final step, a transformer encoder is used to get a higher-level representation of turns. Using categorization based on PHQ-8 and BERT as word embedding, we propose a novel deep learning framework with two-level encoding for detecting depression. In the training step, we use Distress Analysis Interview Corpus (DAIC) dataset, a transcript of the interview data. The model is evaluated with different adjustments to achieve the best performance. Various experiments are designed to show the effectiveness of our method for detecting depression from the psychiatric consultation dataset. As a result, our model performs better than others using the same dataset, achieving 0.76 F1-score.
更多查看译文
关键词
Depression detection,Psychiatric consultations,Turn features,Deep learning
AI 理解论文
溯源树
样例
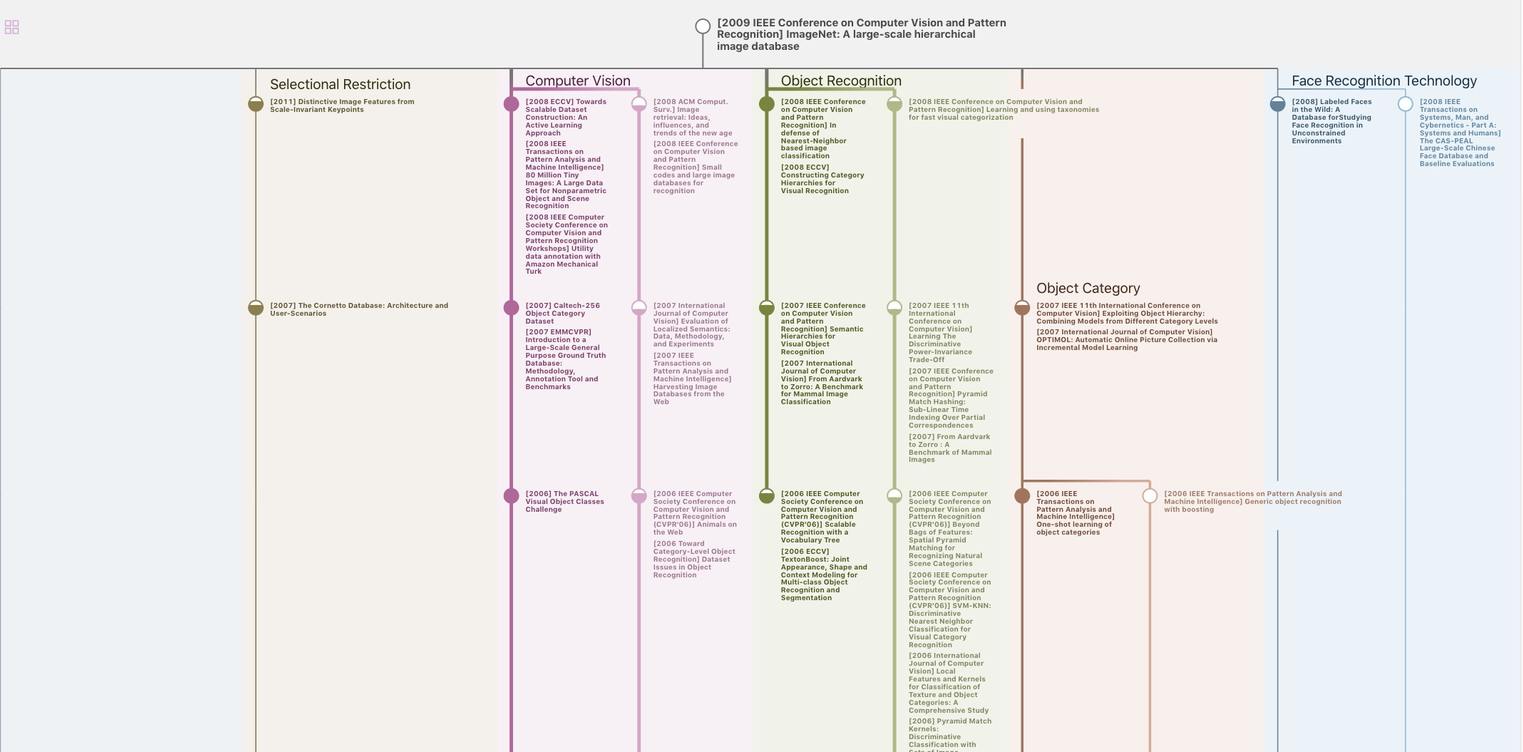
生成溯源树,研究论文发展脉络
Chat Paper
正在生成论文摘要