JULive3D: a live image acquisition protocol for real-time 3D face recognition
Multimedia Tools and Applications(2024)
摘要
The capturing and development of a real time 3D face recognition system is always a big challenge and attracts huge popularity nowadays. This is because, in most security systems employing face recognition, it is always crucial to capture live images and recognize them. This should save time and cost as well, and incredibly is acceptable across all areas of security concerns. This paper deals with the development of an entirely new 3D face recognition system which was developed in Jadavpur University with six subjects captured fully in real time. The main problem which we have tried to address in the present work is that, how various issues like camera calibration, alignment of the camera, distance of the camera from the subject affects the facial recognition rate. We will also analyze how facial registration helps to increase the recognition rates which have been imposed by the above factors. The problem is relevant, because, it’s always a challenge to capture and correctly predict a real time system. The necessary problems needed for setting up a real time system is always a matter to be investigated by the researchers in face recognition domain. The main overview of our present proposed method is to capture the subjects in real-time taking into consideration all the calibration issues like camera alignment, distance of camera from the subject, and several other factors. We have also discussed how these factors affect the recognition rate. Once captured, we have tried to justify the varying recognition rate of the subjects due to the changes in calibration, alignment and other issues. Now, in order to improve the performances of the subjects, we have proposed a new 3D face registration algorithm termed as FaRegAvFM8, which was tested on the subjects from our database acquired in real-time, as well as on Frav3D and GavabDB databases. Our system attained a recognition rate of 95.83% after registration on frontal subjects using Haar wavelet as the feature extraction method, which depicts the robustness of the present system. Not only that, we have improved our recognition rate up to 96% using the Deep Convolutional Neural Network (DCNN).
更多查看译文
关键词
Kinect,Registration,Recognition,Calibration
AI 理解论文
溯源树
样例
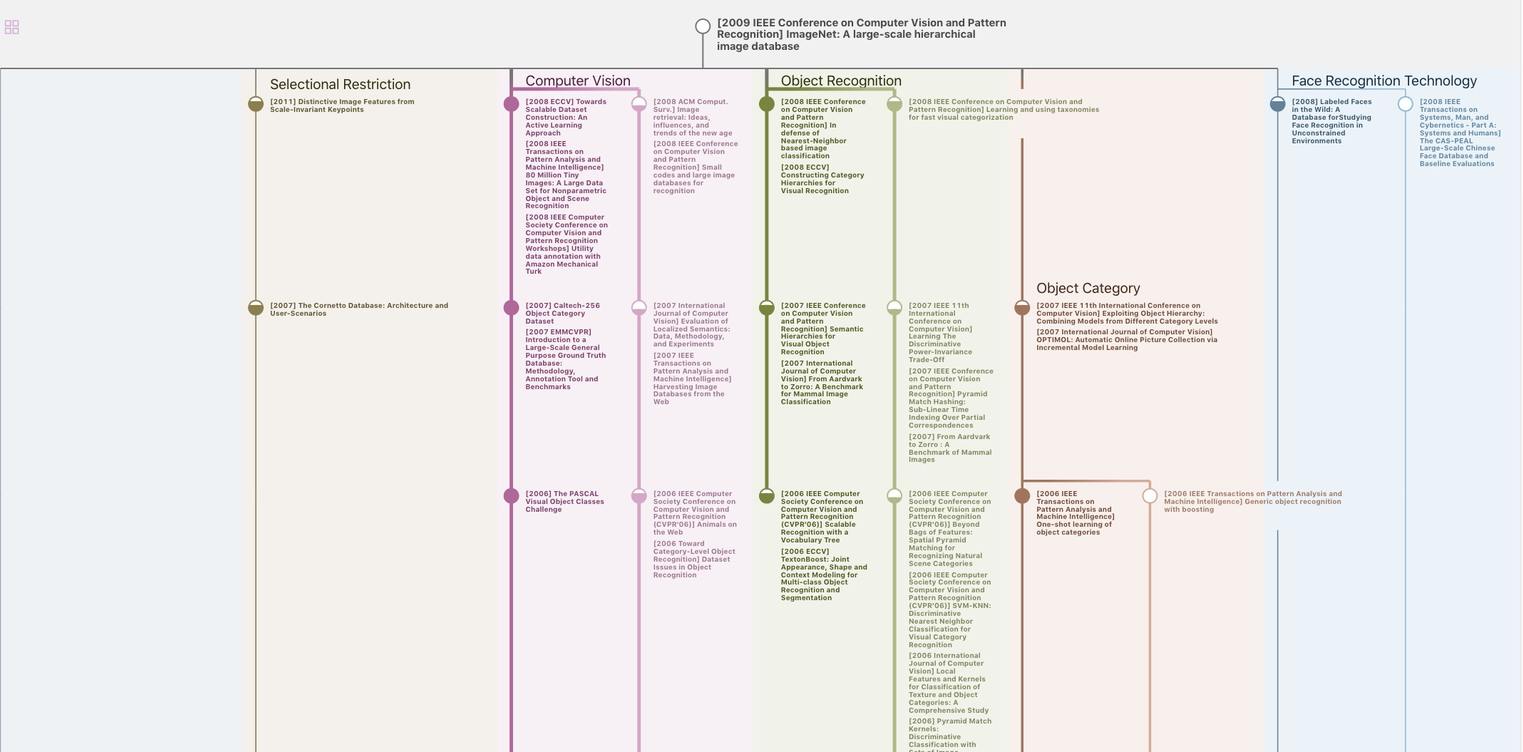
生成溯源树,研究论文发展脉络
Chat Paper
正在生成论文摘要