Learning to Retrieve User Behaviors for Click-through Rate Estimation
ACM Transactions on Information Systems(2023)
摘要
Click-through rate (CTR) estimation plays a crucial role in modern online personalization services. It is essential to capture users’ drifting interests by modeling sequential user behaviors to build an accurate CTR estimation model. However, as the users accumulate a large amount of behavioral data on the online platforms, the current CTR models have to truncate user behavior sequences and utilize the most recent behaviors, which leads to a problem that sequential patterns such as periodicity or long-term dependency are not contained in the recent behaviors but in far back history. However, it is non-trivial to model the entire user sequence by directly using it for two reasons. Firstly, the very long input sequences will make online inference time and system load infeasible. Secondly, the very long sequences contain much noise, thus making it difficult for CTR models to capture useful patterns effectively. To tackle this issue, we consider it from the input data perspective instead of designing more sophisticated yet complex models. As the entire user behavior sequence contains much noise, it is unnecessary to input the entire sequence. Instead, we could just retrieve only a small part of it as the input to the CTR model. In this article, we propose the U ser B ehavior R etrieval (UBR) framework which aims at learning to retrieve the most informative user behaviors according to each CTR estimation request. Retrieving only a small set of behaviors could alleviate the two problems of utilizing very long sequences (i.e., inference efficiency and noisy input). The distinguishing property of UBR is that it supports arbitrary and learnable retrieval functions instead of utilizing a fixed pre-defined function, which is different from the current retrieval-based methods. Offline evaluations on three large-scale real-world datasets demonstrate the superiority and efficacy of the UBR framework. We further deploy UBR at the Huawei App Store, where it achieves 6.6% of eCPM gain in the online A/B test and now serves the main traffic in the Huawei App Store advertising scenario.
更多查看译文
关键词
retrieve user behaviors,rate estimation,click-through
AI 理解论文
溯源树
样例
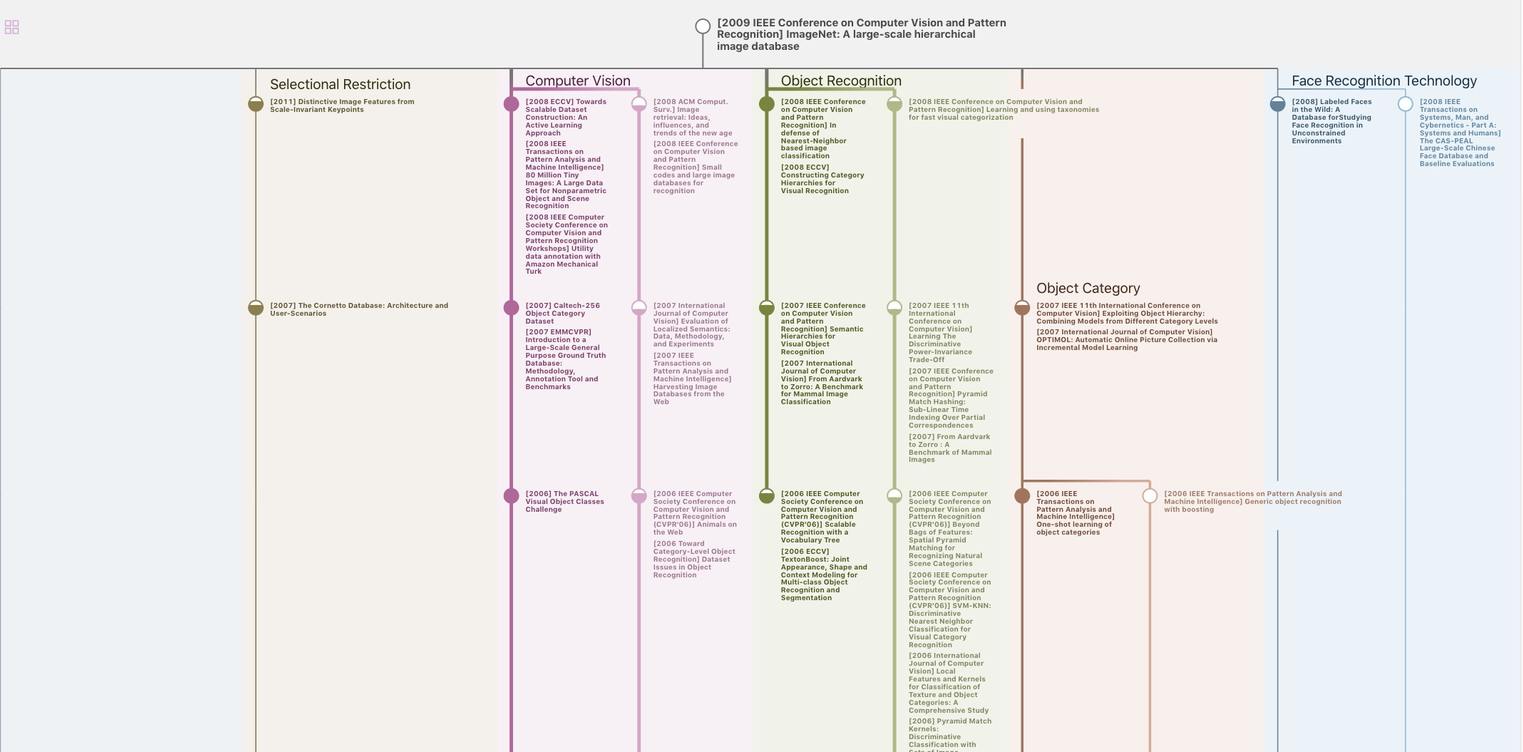
生成溯源树,研究论文发展脉络
Chat Paper
正在生成论文摘要