SMONE: A Session-based Recommendation Model Based on Neighbor Sessions with Similar Probabilistic Intentions
ACM Transactions on Knowledge Discovery from Data(2023)
摘要
A session-based recommendation system (SRS) tries to predict the next possible choice of anonymous users. In recent years, graph neural network (GNN) models have been successfully applied to SRSs and have achieved great success. Using GNN models in SRSs, each session graph is processed successively to obtain the embedding of the node (i.e, each action on an item), which is then imported into the prediction module to generate recommendation results. However, solely depending on the session graph to obtain the node embeddings is not sufficient because each session only involves a few items. Therefore, neighbor sessions have been used to extend the session graph to learn more informative node representations. In this paper, we introduce a S ession-based recommendation MO del based on N eighbor sessions with similar probabilistic int E ntions(SMONE). SMONE models the intentions behind sessions in a probabilistic way and retrieves the neighbor sessions with similar intentions. After the neighbor sessions are found, the target session and its neighbor sessions are modeled as a hypyergraph to learn the contextualized embeddings, which are combined with item embeddings through GNN to produce the final item recommendations. Experiments on real-world datasets prove the effectiveness and superiority of SMONE.
更多查看译文
关键词
Session-based recommender systems, neighbor sessions, probabilistic intentions
AI 理解论文
溯源树
样例
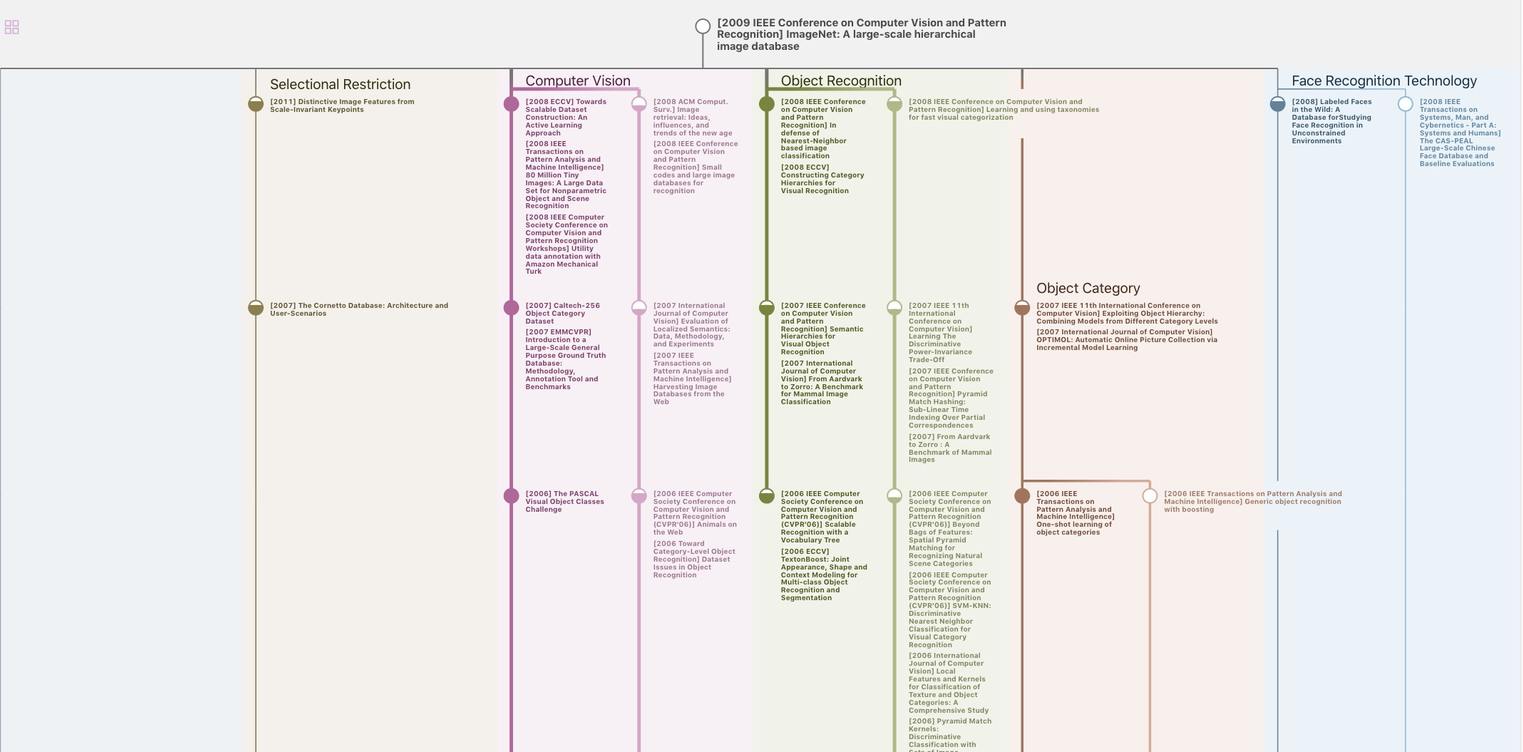
生成溯源树,研究论文发展脉络
Chat Paper
正在生成论文摘要