Prediction of Disc Cutter Replacement of Tunnel Boring Machine Using Denoising Auto Encoder
Flexible Automation and Intelligent Manufacturing: The Human-Data-Technology Nexus(2023)
摘要
While a tunnel boring machine (TBM) is working, rocks are crushed into pieces by disc cutters which often fail during construction. To replace disabled cutters timely, the condition of the cutters needs to be checked regularly. However, this is time-consuming and uneconomical. In this paper, a denoising auto encoder (DAE) model is proposed to judge whether TBM disc cutters need to be replaced. First of all, the field data related to dis cutter status are selected as inputs. Then, the cutter conditions can be learned automatically base on a DAE model. Test result on a water transport tunnel shows that the proposed model can obtain an average accuracy of 99.7% and an average F1 score of 99.4% on field data prediction. Compared with other machine learning and deep learning models, proposed method reduces the need of manual data denoising and feature extraction.
更多查看译文
关键词
Tunnel boring machine, Disc cutter, Deep Learning, Denoising auto encoder
AI 理解论文
溯源树
样例
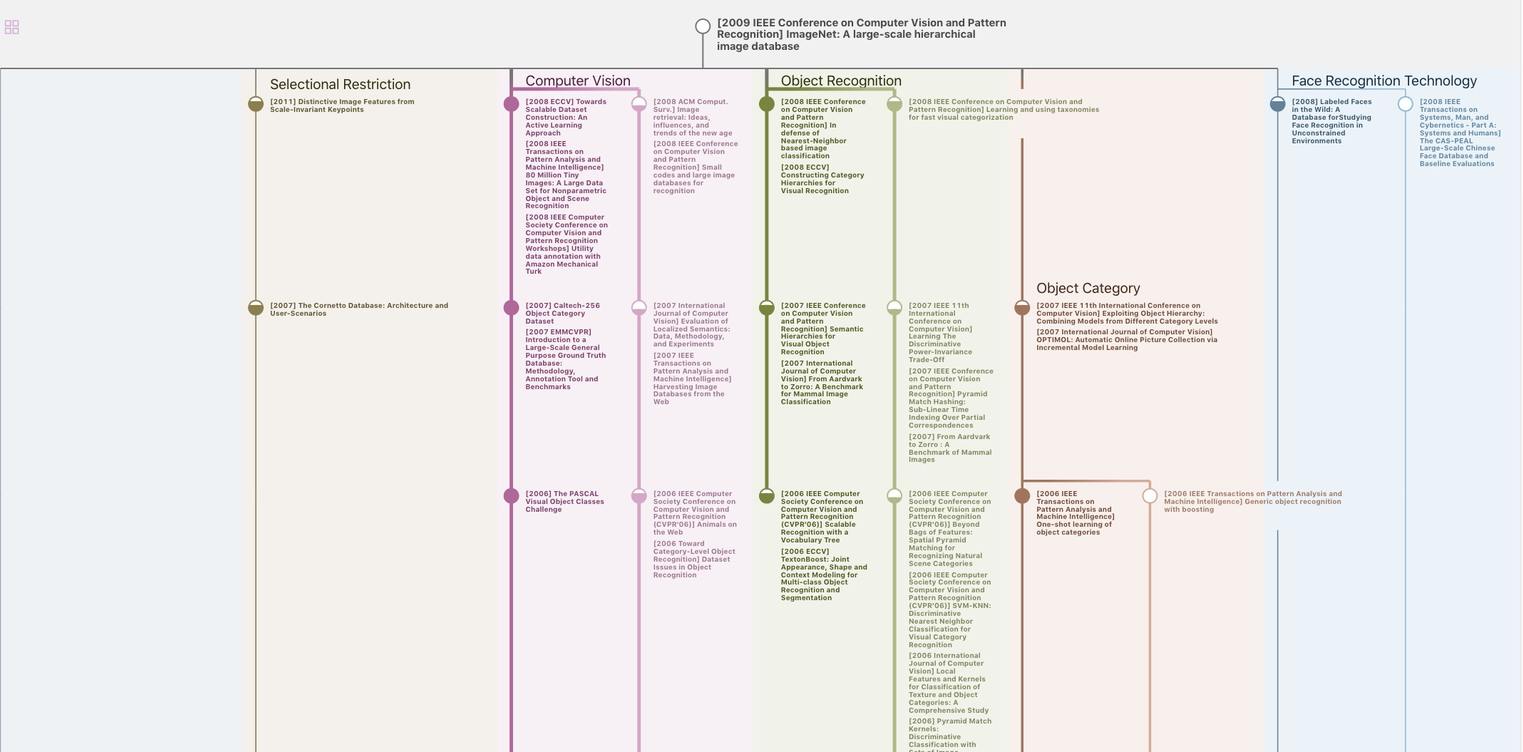
生成溯源树,研究论文发展脉络
Chat Paper
正在生成论文摘要