Extended ADMM for general penalized quantile regression with linear constraints in big data
COMMUNICATIONS IN STATISTICS-SIMULATION AND COMPUTATION(2023)
摘要
Quantile regression offers a powerful means of understanding the comprehensive relationship between response variables and predictors. By formulating prior domain knowledge and assumptions as constraints on parameters, the estimation efficiency can be enhanced. This paper studies some methods based on multi-block ADMM (Alternating Direction Method of Multipliers) to fit general penalized quantile regression models with linear constraints on regression coefficients. Different formulations for handling linear constraints and general penalties are explored and compared. Among these formulations, the most efficient one is identified, which provides an explicit expression for each parameter during iterations and eliminates the nested-loop in existing algorithms. Furthermore, this work addresses the challenges posed by big data by developing a parallel ADMM algorithm suitable for distributed data storage. The algorithm's convergence and a robust stopping criterion are established. To demonstrate the excellent performance of the proposed algorithms, extensive numerical experiments and a real data example are presented. These empirical validations showcase the effectiveness of the methods in handling complex datasets. The details of theoretical proofs and different algorithm variations are provided in the Appendix.
更多查看译文
关键词
ADMM,Big data,General penalty,Linear constraints,Quantile regression
AI 理解论文
溯源树
样例
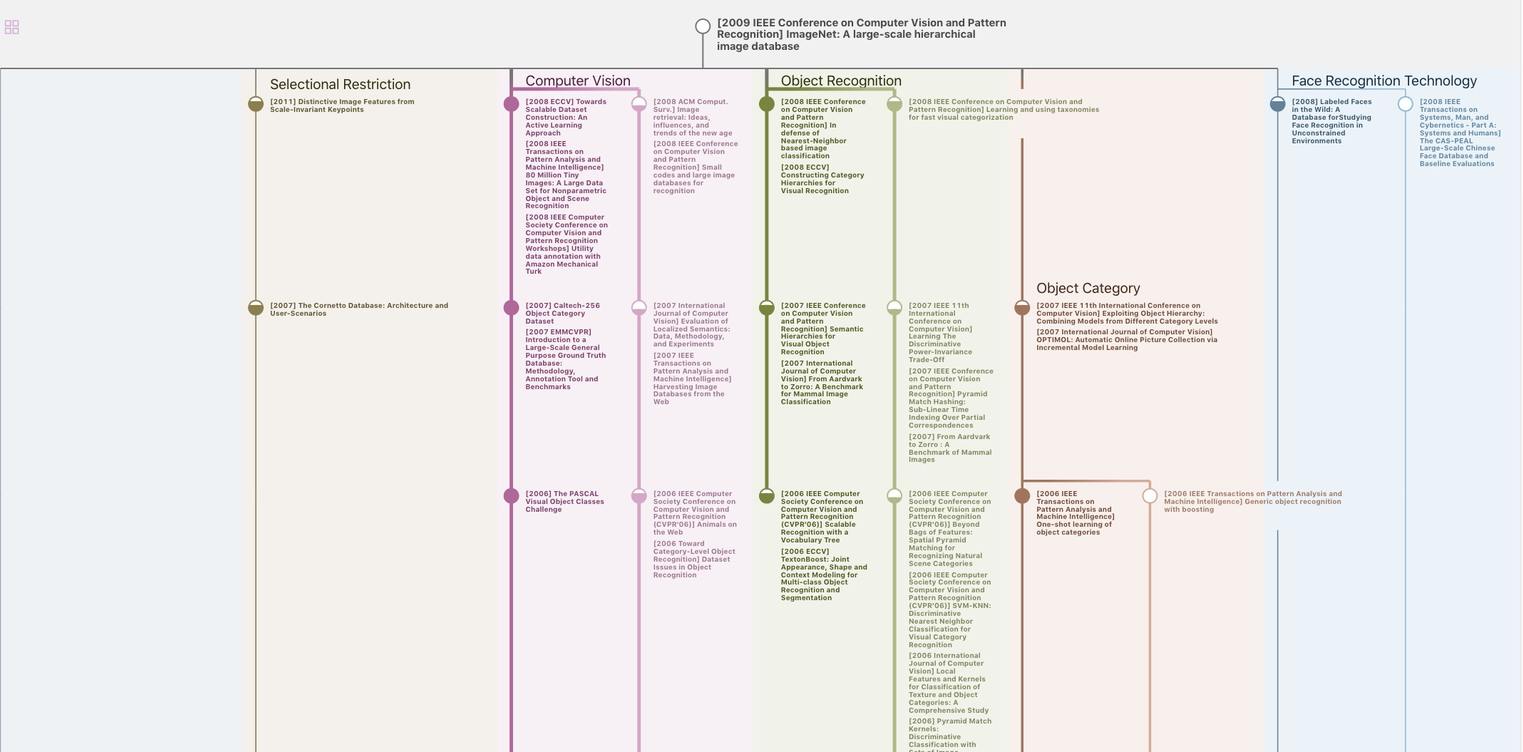
生成溯源树,研究论文发展脉络
Chat Paper
正在生成论文摘要