Efficient Coded Multi-Party Computation at Edge Networks
IEEE TRANSACTIONS ON INFORMATION FORENSICS AND SECURITY(2024)
摘要
Multi-party computation (MPC) is promising for designing privacy-preserving machine learning algorithms at edge networks. An emerging approach is coded-MPC (CMPC), which advocates the use of coded computation to improve the performance of MPC in terms of the required number of workers involved in computations. The current approach for designing CMPC algorithms is to merely combine efficient coded computation constructions with MPC. We show that this approach fails short of being efficient; e.g., entangled polynomial codes are not necessarily better than PolyDot codes in MPC setting, while they are always better for coded computation. Motivated by this observation, we propose a new construction; Adaptive Gap Entangled (AGE) polynomial codes for MPC. We show through analysis and simulations that MPC with AGE codes always perform better than existing CMPC algorithms in terms of the required number of workers as well as computation, storage, and communication overhead.
更多查看译文
关键词
Codes,Servers,Privacy,Machine learning algorithms,Computational efficiency,Computational modeling,Data privacy,Adaptive gap entangled polynomial codes,multi-party computation,coded computation,edge computing,privacy
AI 理解论文
溯源树
样例
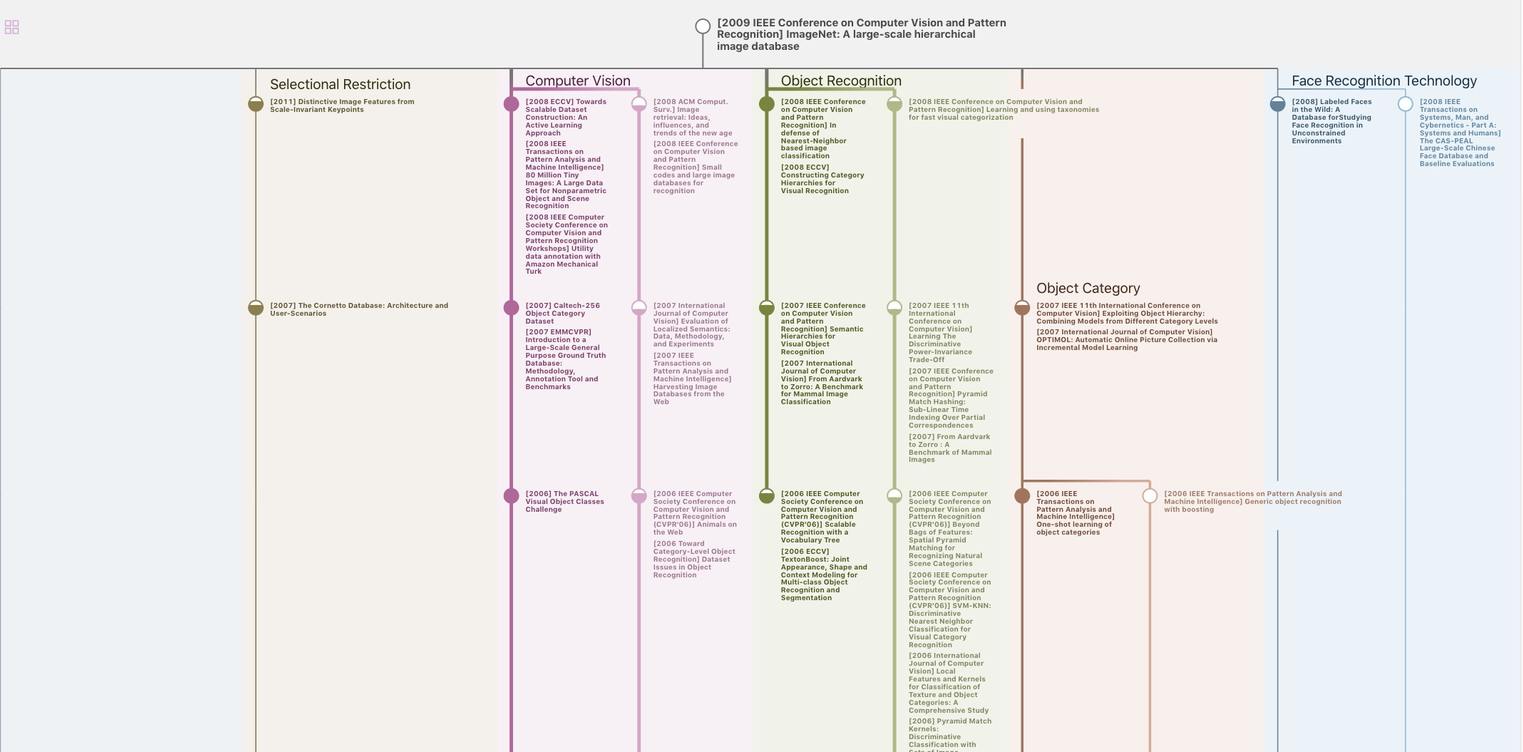
生成溯源树,研究论文发展脉络
Chat Paper
正在生成论文摘要