Federated Learning Framework for Dynamic Power Management in RAN Data Plane Systems
2023 IEEE Wireless Communications and Networking Conference (WCNC)(2023)
摘要
Radio Access Network (RAN) data plane systems in 5G are expected to support multiple user traffic flows demanding high throughput. Base Station (BS) units like Distributed Unit (DU), and Radio Unit (RU) are heavy consumers of power. Network traffic varies over time and peak traffic is rarely seen. These traffic variations provide an opportunity for power saving to reduce operational expenditure (OpEx) during low-traffic periods. In commercial operator deployment, multiple BS units are connected to a Central Management Entity (CME). In this paper, we propose a dynamic power management method to proactively adjust the operating CPU frequency of BS according to the predicted core load. The proposed solution considers deriving a mathematical formula for predicting core load using the computing horsepower of CME with machine learning techniques. To avoid prediction model localization to a single BS and to provide better generalization, Federated Learning (FL) based mathematical formula derivation is proposed. To achieve this, multiple BSs with demographic similarities are grouped. Mathematical formulas for each BS are aggregated and finetuned at the controller using Federated Learning to arrive at a formula to be used by BSs to predict core load. Experiments are performed on real network traces with multiple data flows across different Radio Bearers in New Radio (NR) Radio Link Control (RLC) at RAN Distributed Unit (DU). Results show that the proposed solution reduces processor power consumption by 12%, potentially saving millions of USD annually for commercial deployments.
更多查看译文
AI 理解论文
溯源树
样例
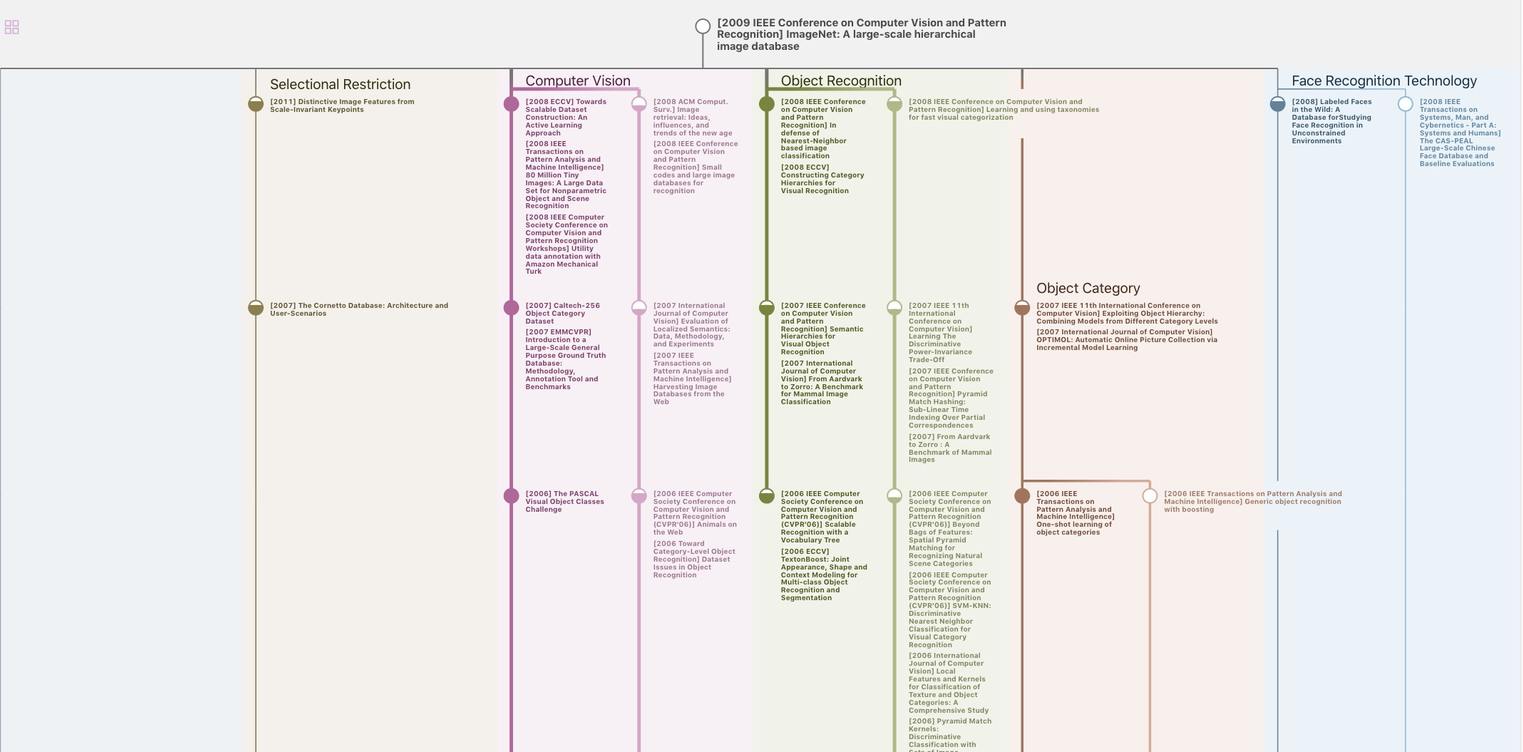
生成溯源树,研究论文发展脉络
Chat Paper
正在生成论文摘要