Characteristics of interannual variability in space-based XCO2 global observations
Atmospheric Chemistry and Physics(2023)
摘要
Atmospheric carbon dioxide (CO2) accounts for the largest radiative forcing among anthropogenic greenhouse gases. There is, therefore, a pressing need to understand the rate at which CO2 accumulates in the atmosphere, including the interannual variations (IAVs) in this rate. IAV in the CO2 growth rate is a small signal relative to the long-term trend and the mean annual cycle of atmospheric CO2, and IAV is tied to climatic variations that may provide insights into long-term carbon–climate feedbacks. Observations from the Orbiting Carbon Observatory-2 (OCO-2) mission offer a new opportunity to refine our understanding of atmospheric CO2 IAV since the satellite can measure over remote terrestrial regions and the open ocean, where traditional in situ CO2 monitoring is difficult, providing better spatial coverage compared to ground-based monitoring techniques. In this study, we analyze the IAV of column-averaged dry-air CO2 mole fraction (XCO2) from OCO-2 between September 2014 and June 2021. The amplitude of the IAV, which is calculated as the standard deviation of the time series, is up to 1.2 ppm over the continents and around 0.4 ppm over the open ocean. Across all latitudes, the OCO-2-detected XCO2 IAV shows a clear relationship with El Niño–Southern Oscillation (ENSO)-driven variations that originate in the tropics and are transported poleward. Similar, but smoother, zonal patterns of OCO-2 XCO2 IAV time series compared to ground-based in situ observations and with column observations from the Total Carbon Column Observing Network (TCCON) and the Greenhouse Gases Observing Satellite (GOSAT) show that OCO-2 observations can be used reliably to estimate IAV. Furthermore, the extensive spatial coverage of the OCO-2 satellite data leads to smoother IAV time series than those from other datasets, suggesting that OCO-2 provides new capabilities for revealing small IAV signals despite sources of noise and error that are inherent to remote-sensing datasets.
更多查看译文
关键词
global observations,interannual variability,xco<sub>2</sub>,space-based
AI 理解论文
溯源树
样例
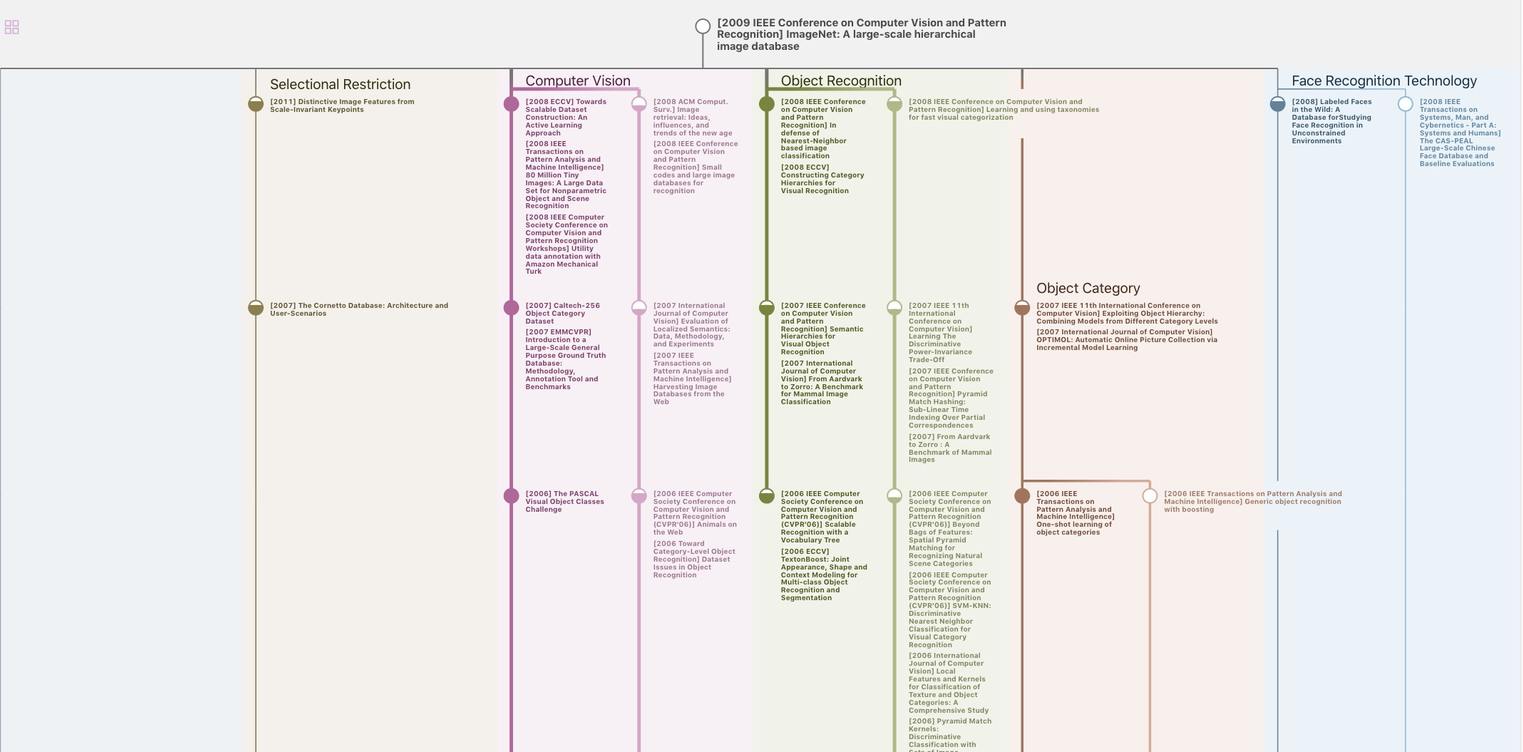
生成溯源树,研究论文发展脉络
Chat Paper
正在生成论文摘要