A Patch Information Supplement Transformer for Person Re-Identification
Electronics(2023)
摘要
Extracting fine-grained features from person images has proven crucial in person re-identification (re-ID). Although the research of convolutional neural networks (CNN) has been very successful in person re-ID, due to the small receptive field and downsampling operation, the existing CNNs cannot solve the problem of information loss. The multi-head attention modules in transformer can solve the above problems well. However, since dicing operations destroy the spatial correlation between patches, transformer still loses some local features. in this paper, we propose the scheme of the patch information supplement transformer (PIT) to extract fine-grained features in the dicing stage. Patch pyramid network (PPN) is introduced to solve the problem of local information loss. This is accomplished by dividing the image into different scales through the dicing operation and adding them together from top to bottom according to the pyramid structure. In addition, we insert a learnable identity information-embedding module (IDE) to reduce the feature bias of clothing and camera perspective. Experiments verify the superiority and effectiveness of PIT compared to state-of-the-art methods.
更多查看译文
关键词
person re-identification,fine-grained features,transformer,patch pyramid network,identity information embedding module
AI 理解论文
溯源树
样例
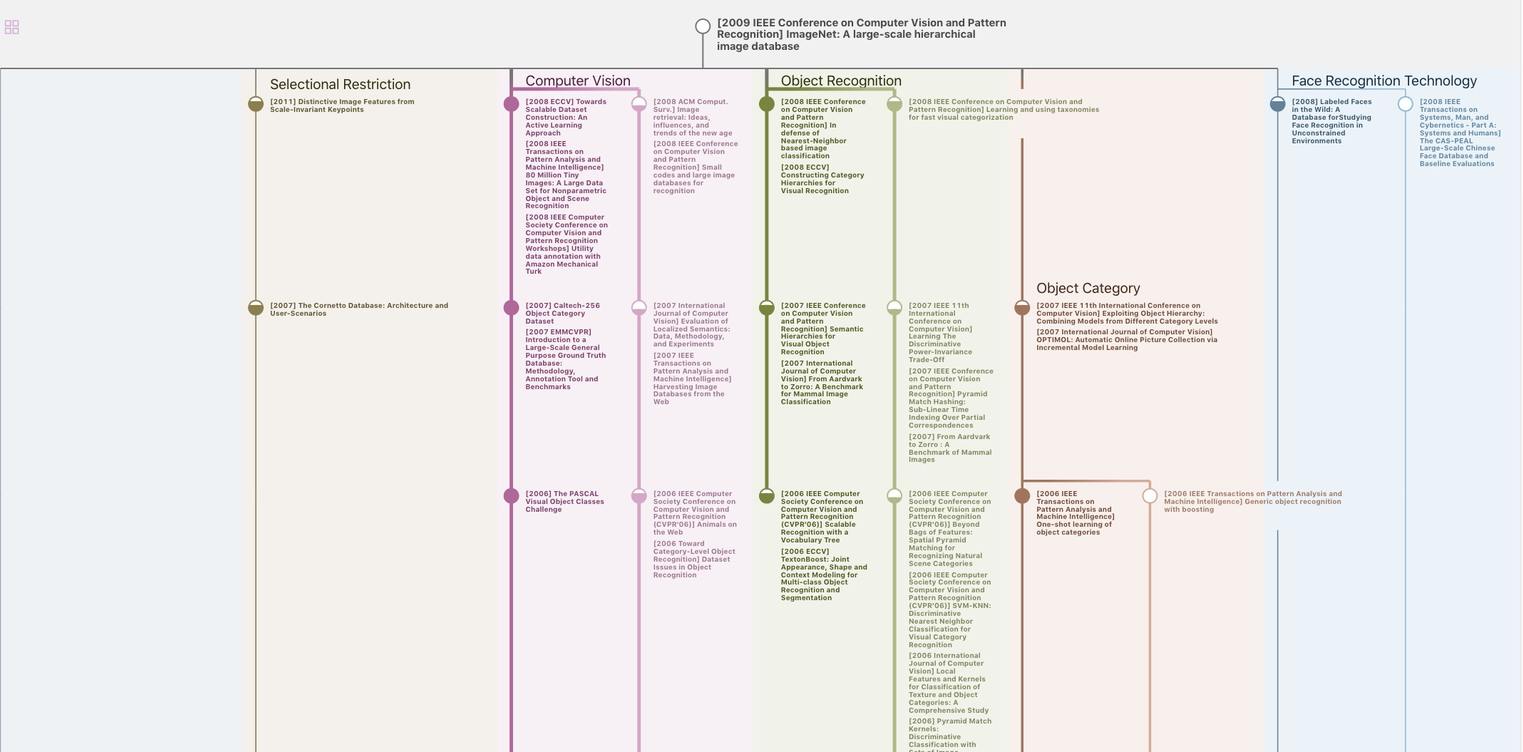
生成溯源树,研究论文发展脉络
Chat Paper
正在生成论文摘要