Towards Theoretical Understanding of Data-Driven Policy Refinement
CoRR(2023)
摘要
This paper presents an approach for data-driven policy refinement in reinforcement learning, specifically designed for safety-critical applications. Our methodology leverages the strengths of data-driven optimization and reinforcement learning to enhance policy safety and optimality through iterative refinement. Our principal contribution lies in the mathematical formulation of this data-driven policy refinement concept. This framework systematically improves reinforcement learning policies by learning from counterexamples surfaced during data-driven verification. Furthermore, we present a series of theorems elucidating key theoretical properties of our approach, including convergence, robustness bounds, generalization error, and resilience to model mismatch. These results not only validate the effectiveness of our methodology but also contribute to a deeper understanding of its behavior in different environments and scenarios.
更多查看译文
关键词
policy,theoretical understanding,data-driven
AI 理解论文
溯源树
样例
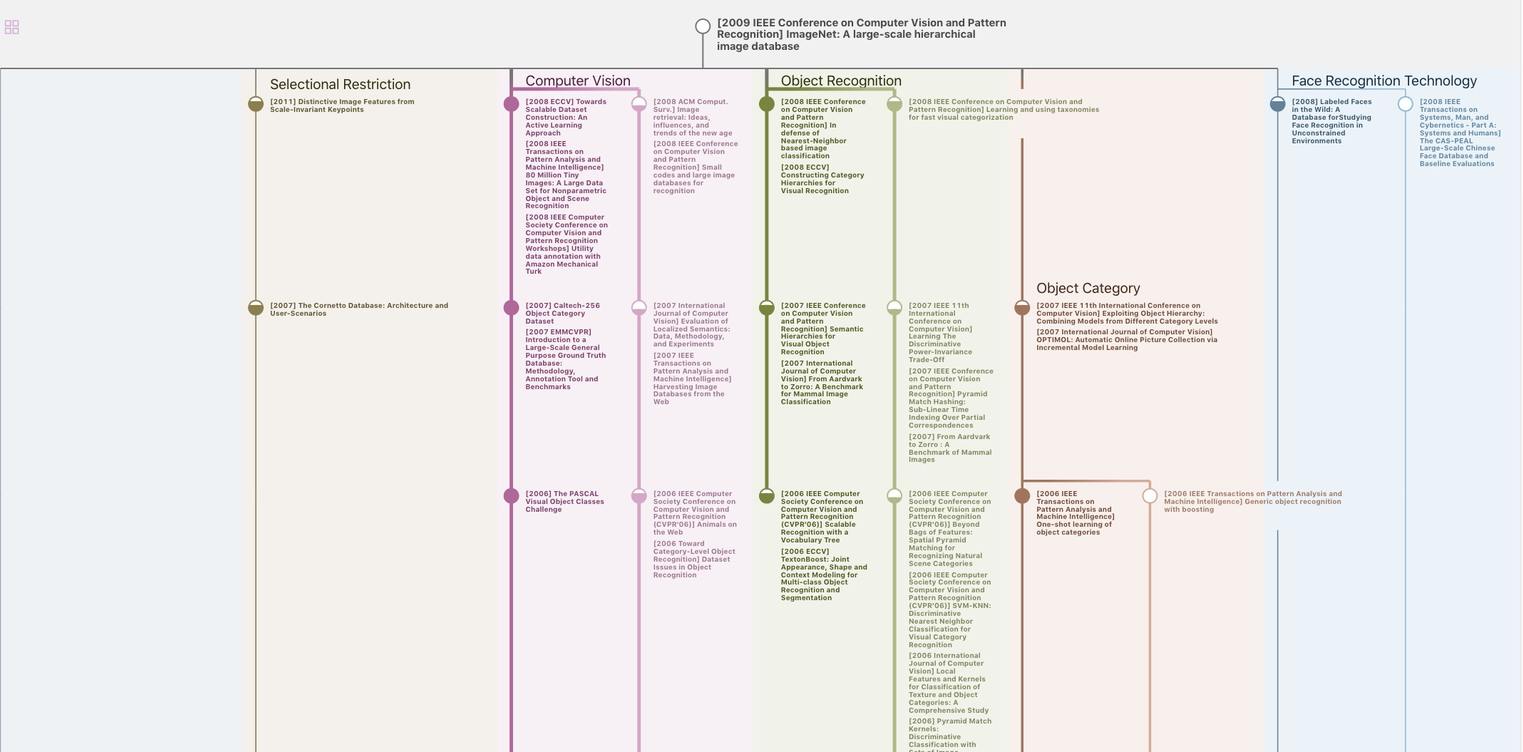
生成溯源树,研究论文发展脉络
Chat Paper
正在生成论文摘要