An Empirical Study on the Robustness of the Segment Anything Model (SAM)
CoRR(2023)
摘要
The Segment Anything Model (SAM) is a foundation model for general image segmentation. Although it exhibits impressive performance predominantly on natural images, understanding its robustness against various image perturbations and domains is critical for real-world applications where such challenges frequently arise. In this study we conduct a comprehensive robustness investigation of SAM under diverse real-world conditions. Our experiments encompass a wide range of image perturbations. Our experimental results demonstrate that SAM's performance generally declines under perturbed images, with varying degrees of vulnerability across different perturbations. By customizing prompting techniques and leveraging domain knowledge based on the unique characteristics of each dataset, the model's resilience to these perturbations can be enhanced, addressing dataset-specific challenges. This work sheds light on the limitations and strengths of SAM in real-world applications, promoting the development of more robust and versatile image segmentation solutions.
更多查看译文
关键词
segment anything model,robustness,empirical study
AI 理解论文
溯源树
样例
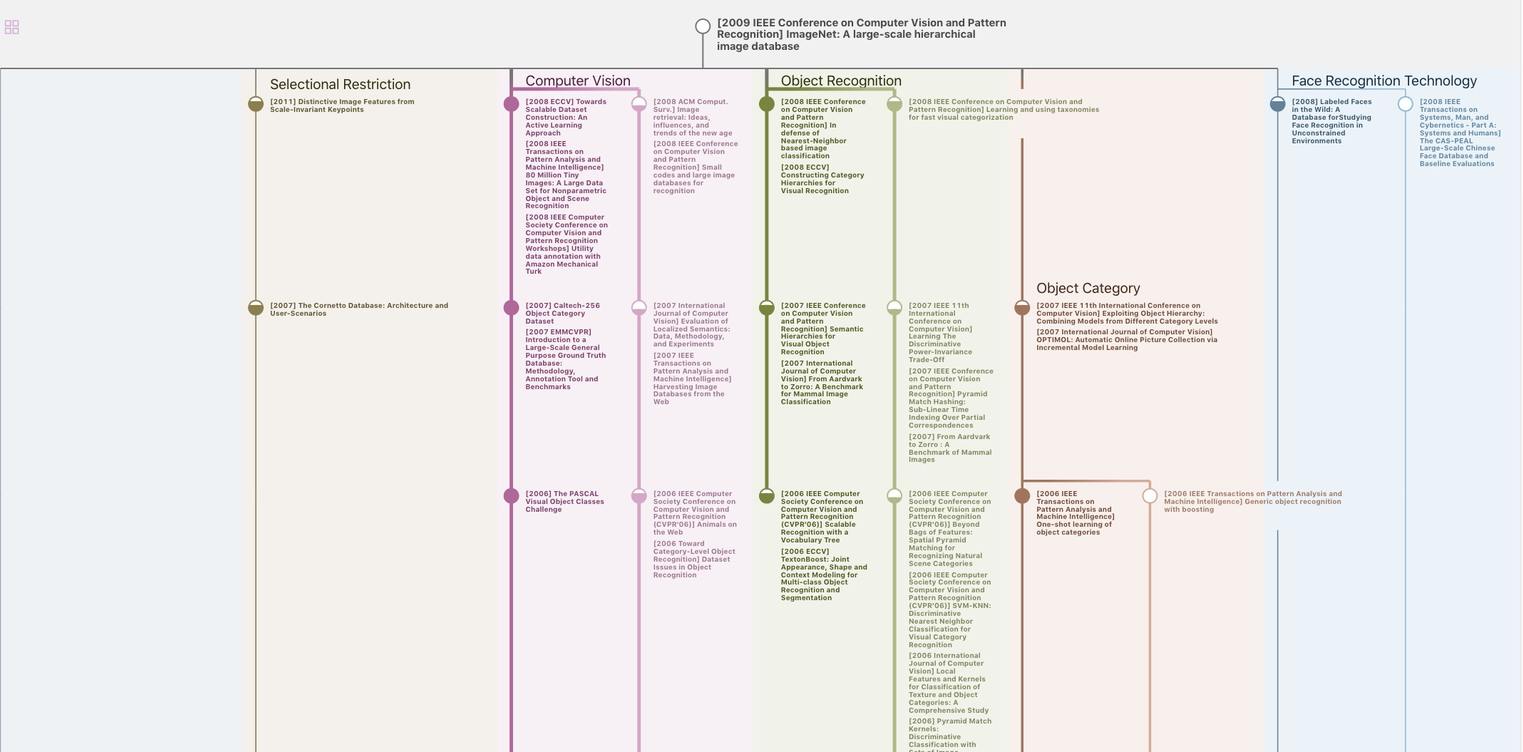
生成溯源树,研究论文发展脉络
Chat Paper
正在生成论文摘要