Super-resolution of brain MRI images based on denoising diffusion probabilistic model
BIOMEDICAL SIGNAL PROCESSING AND CONTROL(2023)
Abstract
Super-resolution of brain magnetic resonance imaging (MRI) generates high resolution brain images as opposed to low-resolution ones, thus providing more detailed anatomical information for diagnosis of neurodegenerative diseases. Although denoising diffusion probabilistic model (DDPM) has displayed remarkable performance in super-resolution of face and natural images, its application to producing high-resolution brain MRI images has not been explored. This study proposed a new deep-learning super-resolution framework for brain MRI images based on DDPM, via incorporating self-attention mechanism into DDPM. The main improvements are as follows: (a) Only one input channel is preserved. (b) The number of baseline channels is reduced from 64 to 32, to improve training speed. (c) Self-attention mechanism is added to 32 x 32, 16 x 16, and 8 x 8 resolution layers rather than only to 16 x 16 layer. (d) Feature-wise affine transformation is added to residual block. Experimental results on open T1-and diffusion-weighted brain MRI datasets show that our DDPM model outperformed the state-of-the-art super-resolution methods. In terms of learned perceptual image patch similarity (LPIPS) metric, the proposed DDPM model achieved the least distortion of generated super-resolution brain MRI images. This framework can also be conveniently used to reconstruct high-resolution MRI images of other body parts such as spinal and knee in future.
MoreTranslated text
Key words
brain mri images,diffusion,super-resolution
AI Read Science
Must-Reading Tree
Example
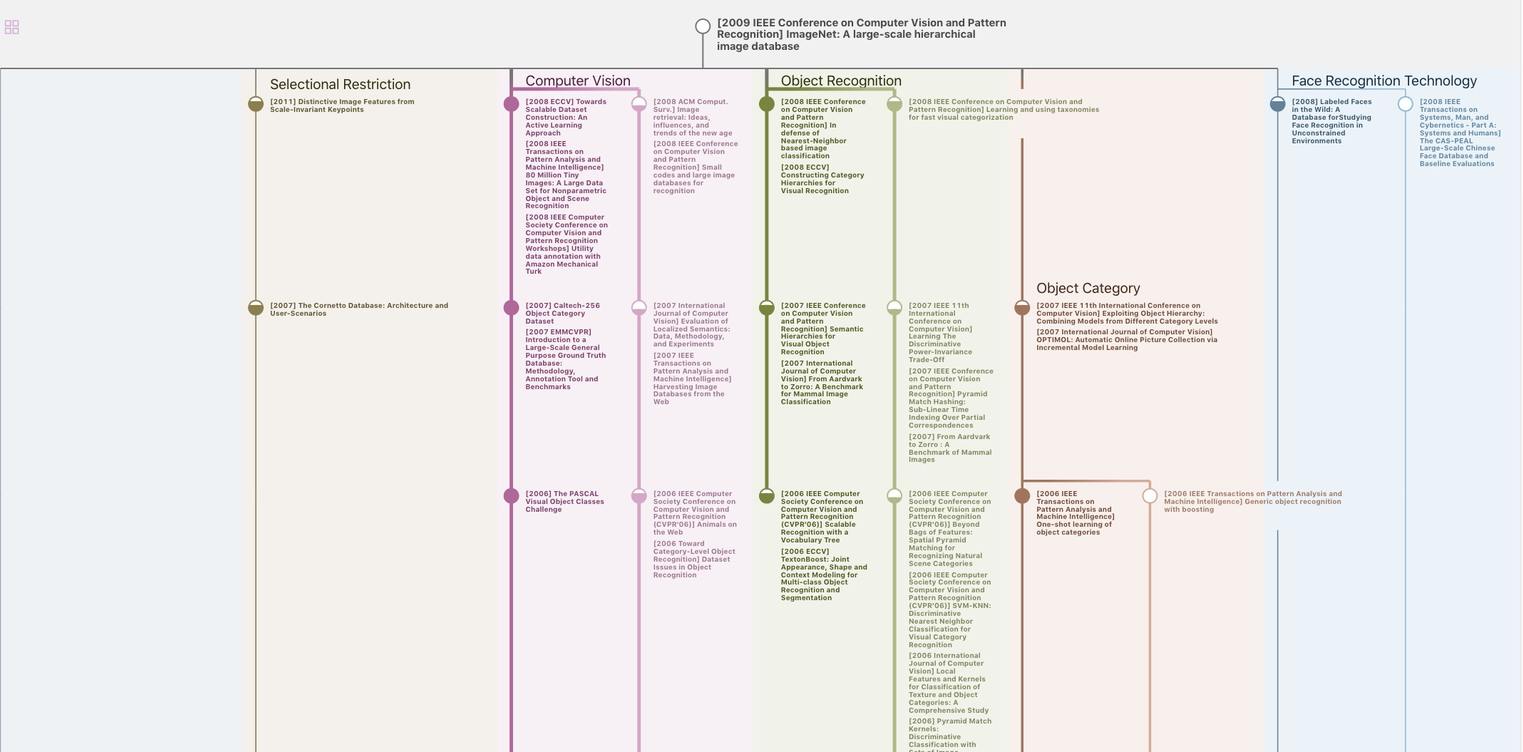
Generate MRT to find the research sequence of this paper
Chat Paper
Summary is being generated by the instructions you defined