Global-and-Local sampling for efficient hybrid task self-supervised learning
KNOWLEDGE-BASED SYSTEMS(2023)
摘要
Siamese-architecture-based self-supervised learning usually suffers from relatively high computational consumption and unsatisfactory performance because of its slow convergence and poor feature extraction capability. To alleviate these issues, we propose a self-supervised method, called SSL2, that is both efficient and effective. Specifically, a global and local sampling method is proposed to increase the number of samples while maintaining connections between semantic features. More significantly, SSL2 maintains low computational complexity and facilitates the establishment of mapping relationships between global comprehensive and local detailed features compared with other methods. In addition, an information retainer projection head (IRPH) is proposed to further balance the information between detailed inconsistency and semantic consistency. Finally, hybrid tasks are embedded into SSL2 to optimize the model so that it can effectively leverage the data provided by global and local sampling. Extensive qualitative and quantitative evaluations of various types of benchmarks illustrate that SSL2 outperforms existing self-supervised frameworks in commonly used computer vision tasks. Specifically, SSL2 achieved satisfactory performance with linear classification on ImageNet, outperforming MoCo-v2 by 2.2% with fewer calculations, and it also achieved competitive results compared with other state-of-the-art methods. (c) 2023 Elsevier B.V. All rights reserved.
更多查看译文
关键词
learning,global-and-local,self-supervised
AI 理解论文
溯源树
样例
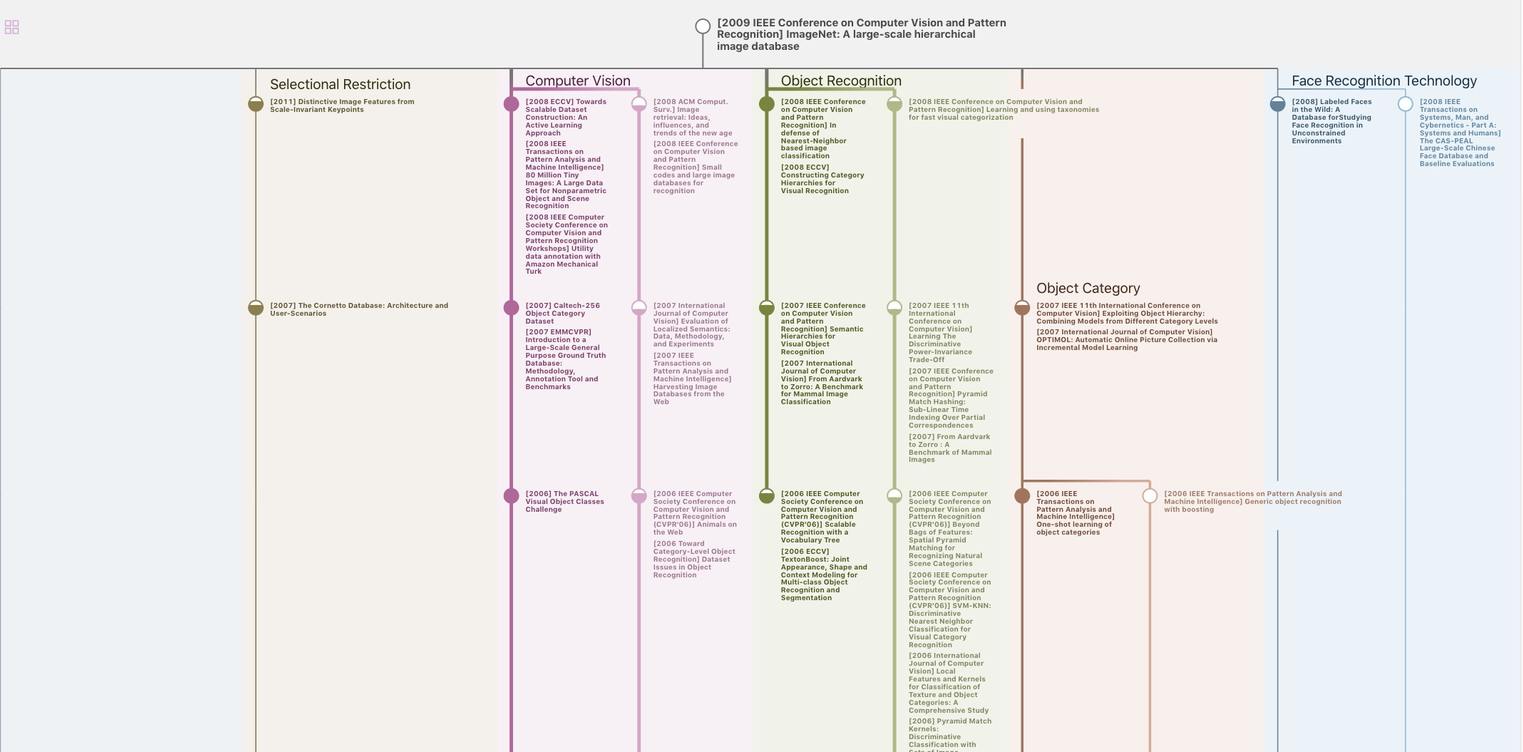
生成溯源树,研究论文发展脉络
Chat Paper
正在生成论文摘要