Level-set-based multiplicative intrinsic component optimization for brain tissue segmentation in T1-W and T2-W modality MRI
EXPERT SYSTEMS WITH APPLICATIONS(2023)
摘要
Brain tissue segmentation is fundamental to structure extraction and quantitative analysis, thereby plays a critical role in lesion detection and aid diagnosis. However, intensity inhomogeneity caused by bias field presents a considerable challenge in accurate segmentation. Multiplicative intrinsic component optimization is one of the most widely used models for brain tissue segmentation. Nevertheless, limited by clustering properties and no penalty term, the accuracy decreases rapidly with the increased number of tissues and the enhancement of noise. To seek better approaches to these issues, a level-set-based method with constraint term is proposed in this paper. First, the cerebrospinal fluid atlas representing pre-segmented tissue and the white matter atlas utilized in the constraint term are obtained from the difference image composed of T1-and T2-weighted magnetic resonance images. Then, the T1-weighted image is modeled as multiplicative components, namely bias field and true image. A novel membership function driven by level set method is presented for the true image. Finally, the contour evolution for tissue segmentation and the component optimization for bias field correction are simultaneously performed during an energy minimization. Experiments on the BrainWeb dataset and clinical brain images have validated the segmentation ability of the proposed method. Comparisons with some state-of-the-art approaches have demonstrated the superiority of our method in terms of accuracy and robustness.
更多查看译文
关键词
Magnetic resonance imaging,Brain tissue segmentation,Intensity inhomogeneity,Bias field,Multiplicative intrinsic component,Level set method
AI 理解论文
溯源树
样例
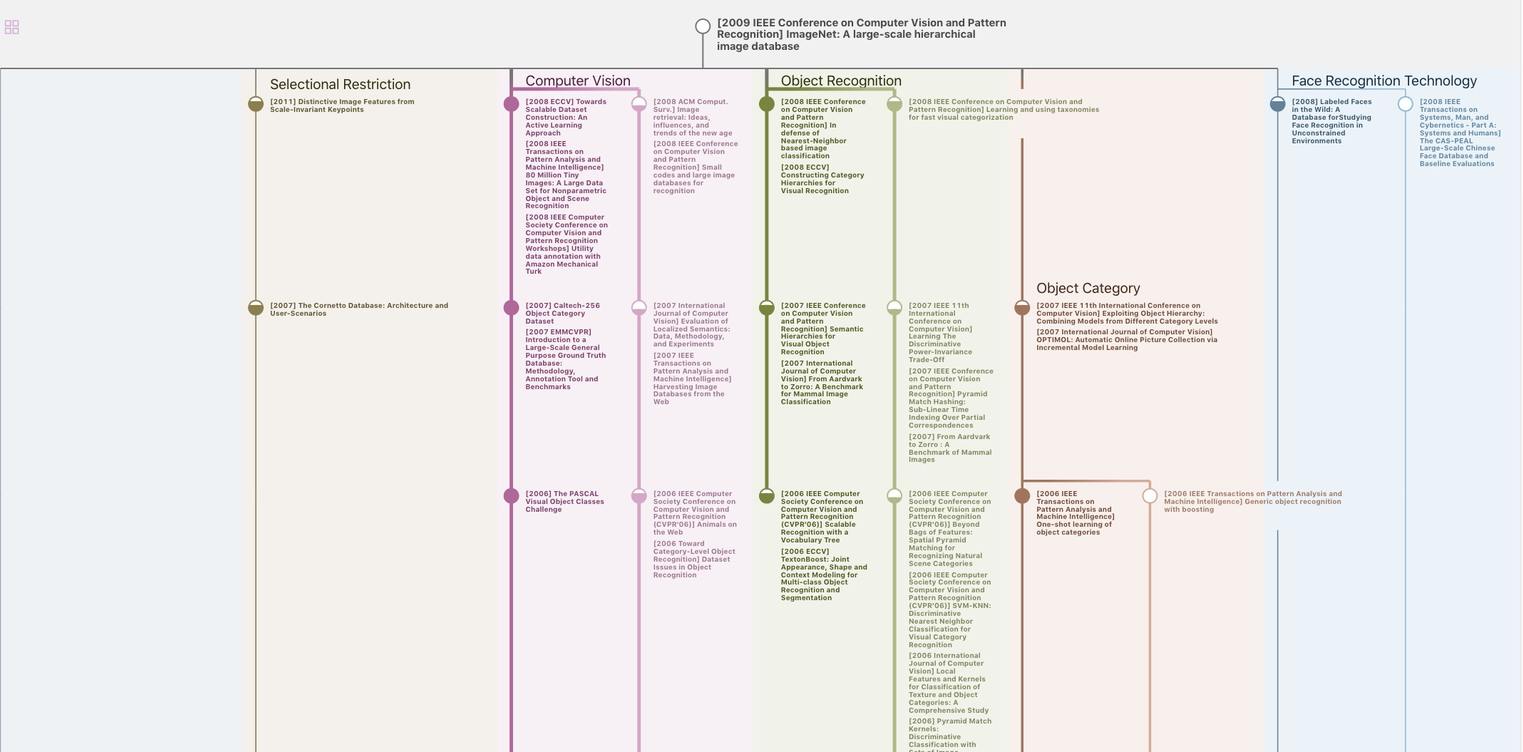
生成溯源树,研究论文发展脉络
Chat Paper
正在生成论文摘要