Urban network-wide traffic volume estimation under sparse deployment of detectors
TRANSPORTMETRICA A-TRANSPORT SCIENCE(2024)
摘要
Sensing network-wide traffic information is fundamental for the sustainable development of urban planning and traffic management. However, owing to the limited budgets or device maintenance costs, detector deployment is usually sparse. Obtaining full-scale network volume using detectors is neither effective nor practical. Existing works primarily focus on improving the estimation accuracy using multi-correlation of networks and ignore the underlying challenges, particularly for these entire undetected road segments in sparse detector deployment scenarios. Here our study proposes a tailored transfer learning framework called the transfer learning-based least square support vector regression (TL-LSSVR) model. Network-wide volume can be estimated by fusing active detectors (taxi GPS data) and fixed passive detectors (license plate recognition data). Numerical experiments are carried out on a real-world road network in Nanjing, China. It is demonstrated that our approach achieves high performance even under sparse deployment of detectors and outperforms other baselines significantly.
更多查看译文
关键词
Network-wide volume estimation,Sparse detectors deployment,Transfer learning,Data fusion,Similarity analysis
AI 理解论文
溯源树
样例
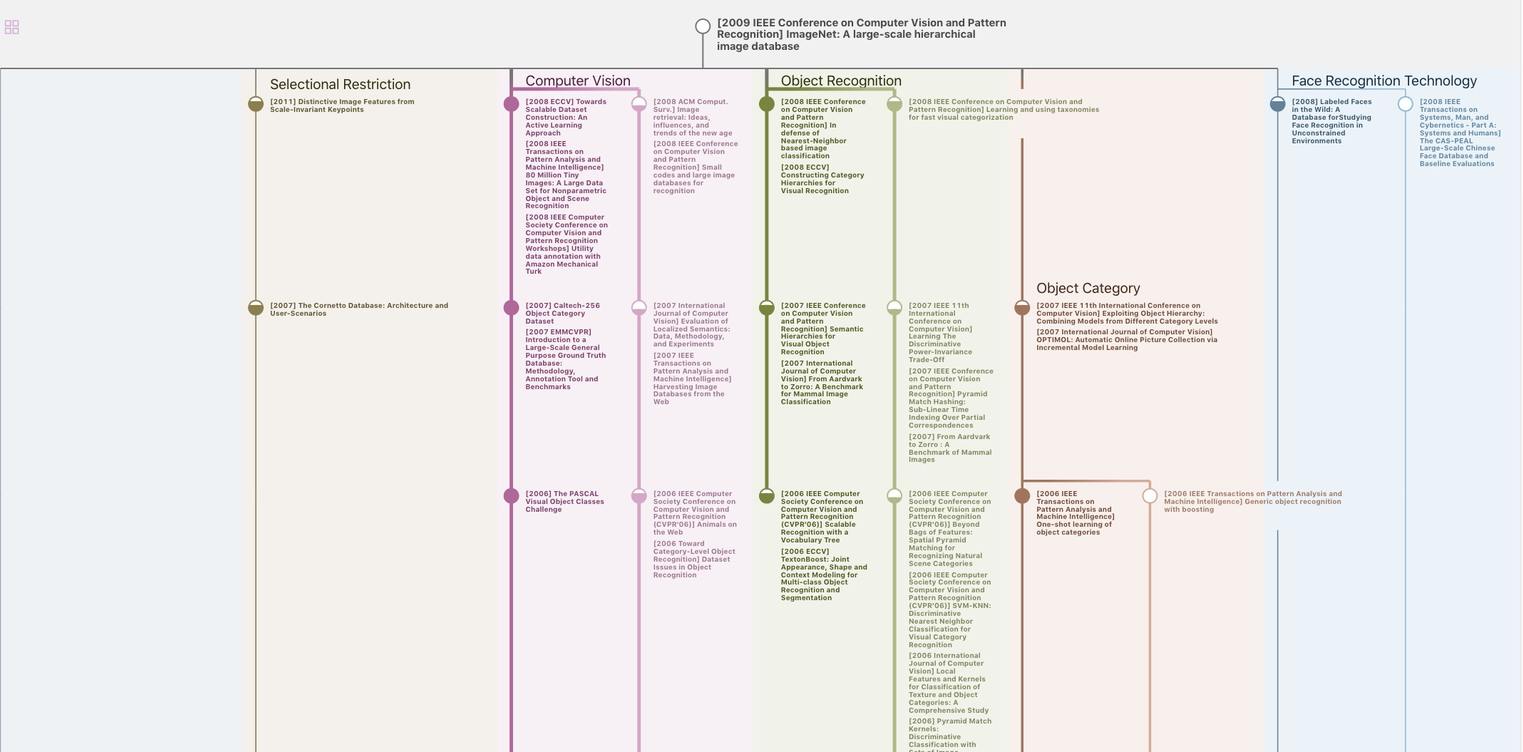
生成溯源树,研究论文发展脉络
Chat Paper
正在生成论文摘要