Hardware implementation of 1D-CNN architecture for ECG arrhythmia classification
BIOMEDICAL SIGNAL PROCESSING AND CONTROL(2023)
摘要
Electrocardiography (ECG) has been used as a diagnostic tool for various heart diseases. It is most effective in detecting myocardial infarction and fatal arrhythmias. This work proposes a safety-critical hardware system for early arrhythmia diagnoses, particularly Atrial Fibrillation. This diagnosis is fairly accurate and unbiased. It is attempted using 1D Convolutional Neural Network (CNN) architecture analysis with the physionet/computing in cardiology challenge database considering the trade-off between accuracy and computational complexity. The state-of-the-art methods do not provide such analysis for CNN hardware implementation. Two software CNN structures are introduced in this work. The foremost Supreme CNN Architecture (SCA) gives an accuracy of 99.17%, which is 7.04% more than existing 1D-CNN architectures for Atrial Fibrillation (AF) classification. Further, it has 18.93%, 12.3%, and 15.99% more precision, recall, and F1 score compared to the state-of -the-art method. It is helpful for software-based arrhythmia classification. The second proposed architecture is the Software-Selected CNN Architecture (SSCA), having lower computational complexity and providing 98.95% accuracy for AF classification. It is used for hardware realization through various optimization techniques considering a reasonable trade-off between accuracy and computational complexity. The proposed multiplier-less Hardware CNN Architecture (HCA) achieves 97.34% Atrial fibrillation classification accuracy for arrhythmia detection. Further, it consumes only 628 mW power on ZYNQ Ultrascale (ZCU106) FPGA, which is 66.95% lesser than the existing 1D-CNN hardware.
更多查看译文
关键词
ECG, Arrhythmia, Atrial Fibrillation, CNN, Hardware architecture, FPGA
AI 理解论文
溯源树
样例
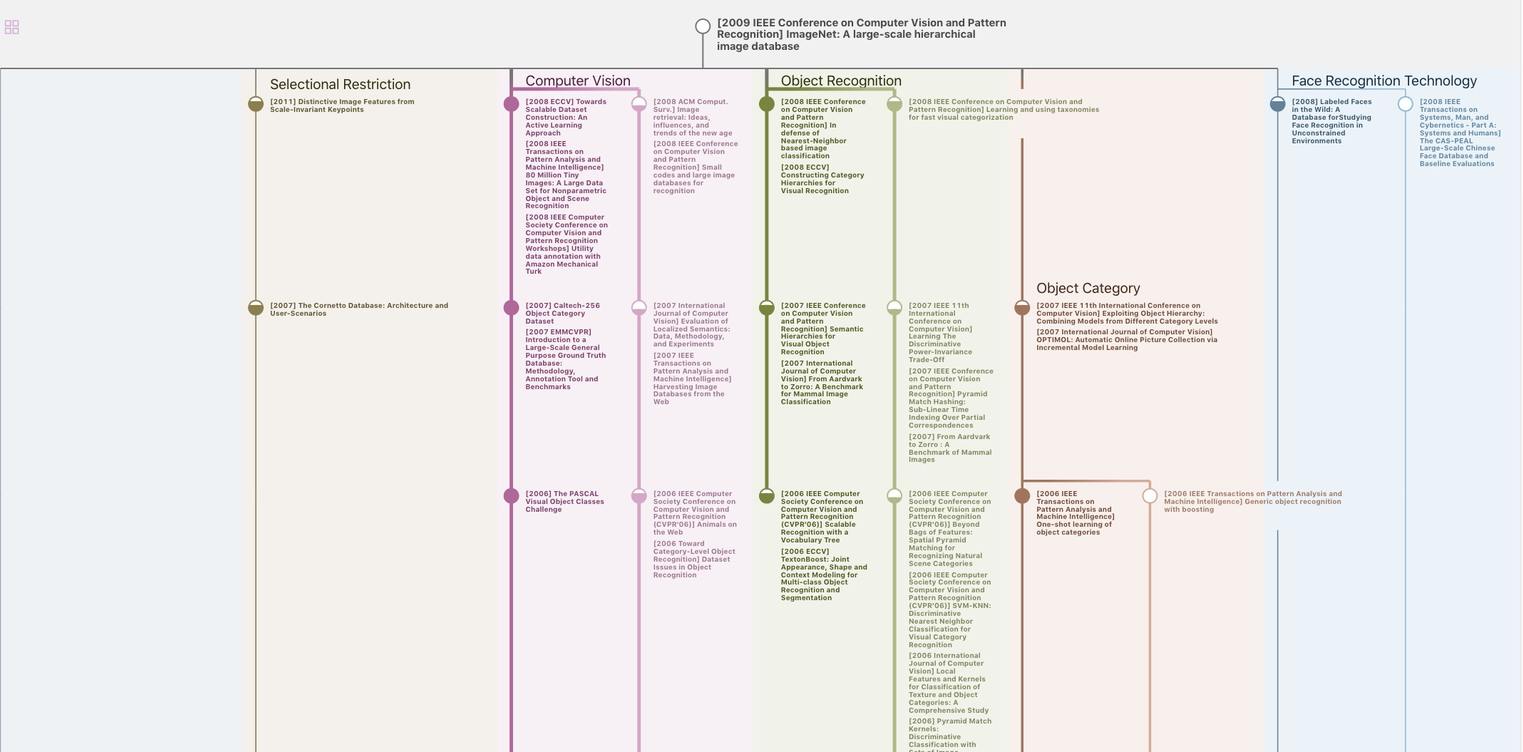
生成溯源树,研究论文发展脉络
Chat Paper
正在生成论文摘要