A deep neural network for general scattering matrix
NANOPHOTONICS(2023)
Abstract
The scattering matrix is the mathematical representation of the scattering characteristics of any scatterer. Nevertheless, except for scatterers with high symmetry like spheres or cylinders, the scattering matrix does not have any analytical forms and thus can only be calculated numerically, which requires heavy computation. Here, we have developed a well-trained deep neural network (DNN) that can calculate the scattering matrix of scatterers without symmetry at a speed thousands of times faster than that of finite element solvers. Interestingly, the scattering matrix obtained from the DNN inherently satisfies the fundamental physical principles, including energy conservation, time reversal and reciprocity. Moreover, inverse design based on the DNN is made possible by applying the gradient descent algorithm. Finally, we demonstrate an application of the DNN, which is to design scatterers with desired scattering properties under special conditions. Our work proposes a convenient solution of deep learning for scattering problems.
MoreTranslated text
Key words
deep neural network,inverse problem,scattering matrix
AI Read Science
Must-Reading Tree
Example
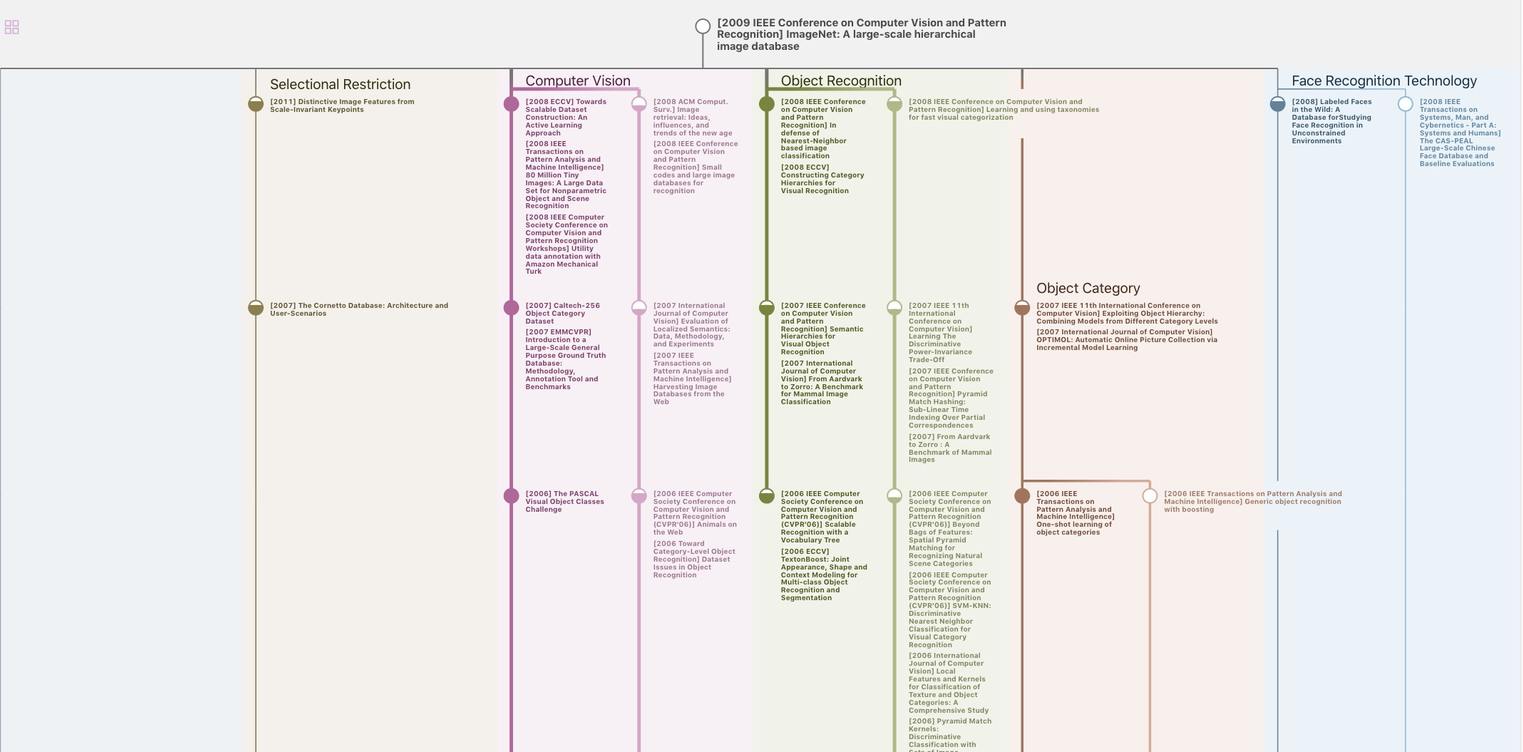
Generate MRT to find the research sequence of this paper
Chat Paper
Summary is being generated by the instructions you defined