Multi-step Time Series Forecasting on the Temperature of Lithium-Ion Batteries
Journal of energy storage(2023)
摘要
Lithium-ion battery temperature monitoring contributes to the higher performance of lithium batteries and reduces the risk of thermal runaway. Since the battery temperature can be approximated as a time series, this work reports a new model named convolutional transformer (Convtrans) for multi-step time series forecasting, which obtains pleasing results. To evaluate our model, we present a cross-sectional comparison of the model with the other three mainstream algorithms in multi-step time series forecasting and a vertical comparison with single-step time forecasting. On one hand, Convtrans has the minimum root mean square error with the highest prediction accuracy and can also predict the trend and shape of temperature curves compared with the other three mainstream algorithms, which means that it has the best results in multi-step time series forecasting. On the other hand, compared with single-step time series forecasting, Convtrans predicts 24 times temperature data while doesn't sacrifice much accuracy even though it costs 6 times running time. Furthermore, in the case of predicting more points, it also maintains good accuracy and can perfectly predict trends of the temperature. In all, we prove the superiority of multi-step time series forecasting over a long period. Therefore, multi-step time series forecasting based on Convtrans can sever as the battery temperature prognostic technology providing timely warnings to assist battery thermal management.
更多查看译文
关键词
Convolutional transformer,Multi-step time series forecasting,Battery thermal management
AI 理解论文
溯源树
样例
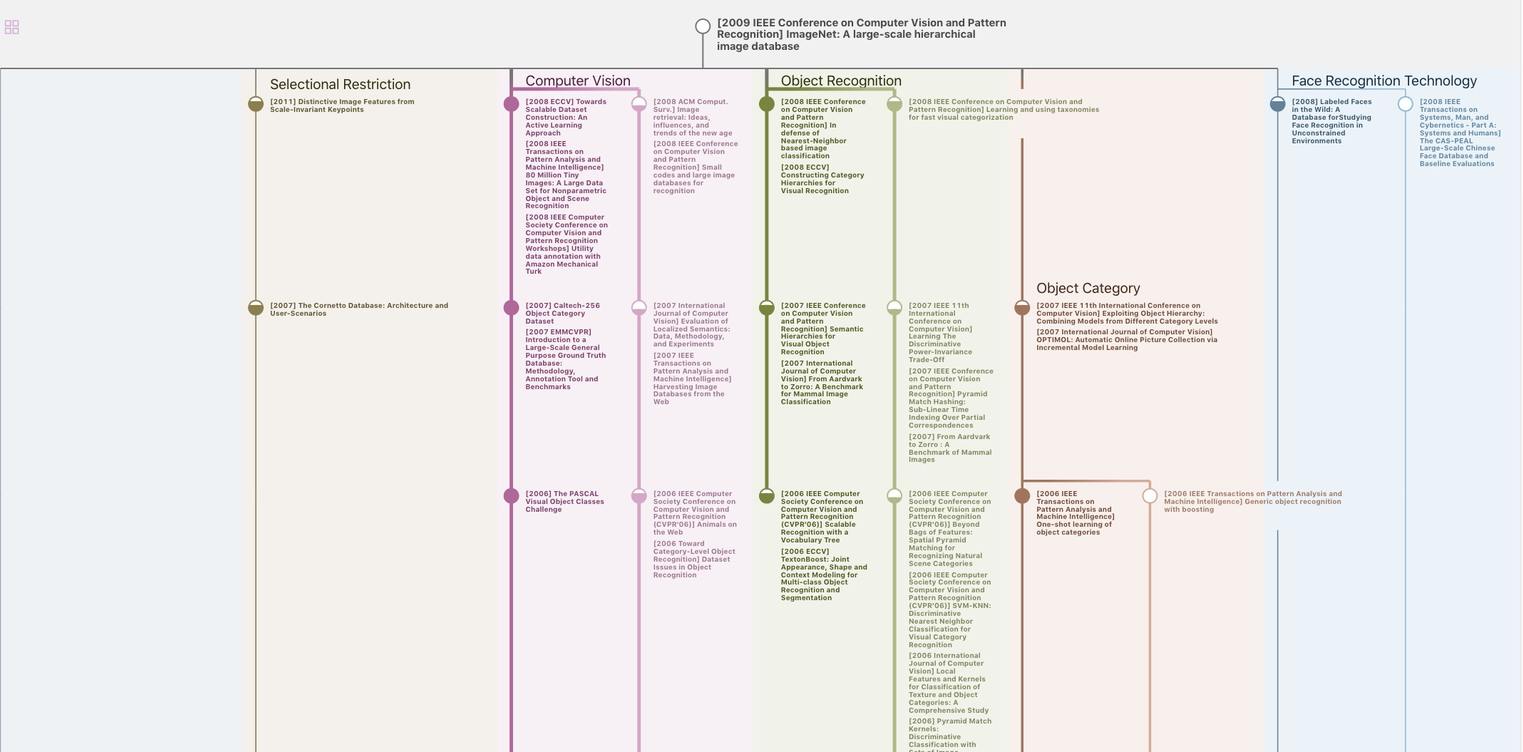
生成溯源树,研究论文发展脉络
Chat Paper
正在生成论文摘要