Lasso-based variable selection methods in text regression: the case of short texts
ASTA-ADVANCES IN STATISTICAL ANALYSIS(2024)
摘要
Communication through websites is often characterised by short texts, made of few words, such as image captions or tweets. This paper explores the class of supervised learning methods for the analysis of short texts, as an alternative to unsupervised methods, widely employed to infer topics from structured texts. The aim is to assess the effectiveness of text data in social sciences, when they are used as explanatory variables in regression models. To this purpose, we compare different variable selection procedures when text regression models are fitted to real, short, text data. We discuss the results obtained by several variants of lasso, screening-based methods and randomisation-based models, such as sure independence screening and stability selection, in terms of number and importance of selected variables, assessed through goodness-of-fit measures, inclusion frequency and model class reliance. Latent Dirichlet allocation results are also considered as a term of comparison. Our perspective is primarily empirical and our starting point is the analysis of two real case studies, though bootstrap replications of each dataset are considered. The first case study aims at explaining price variations based on the information contained in the description of items on sale on e-commerce platforms. The second regards open questions in surveys on satisfaction ratings. The case studies are different in nature and representative of different kinds of short texts, as, in one case, a concise descriptive text is considered, whereas, in the other case, the text expresses an opinion.
更多查看译文
关键词
Text mining,Lasso,Variable screening,Stability selection,Latent Dirichlet allocation
AI 理解论文
溯源树
样例
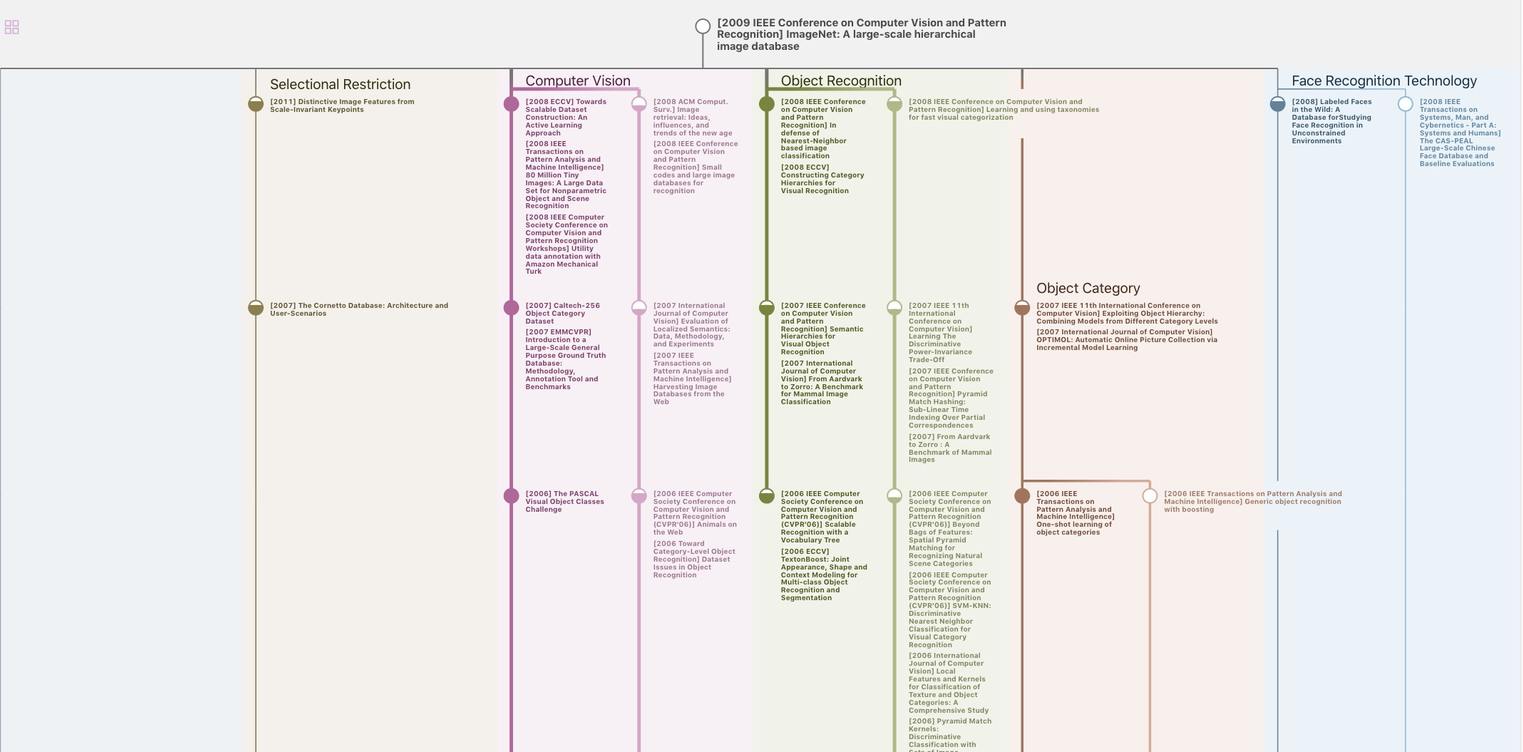
生成溯源树,研究论文发展脉络
Chat Paper
正在生成论文摘要