Damage detection on steel-reinforced concrete produced by corrosion via YOLOv3: A detailed guide
FRONTIERS IN BUILT ENVIRONMENT(2023)
Abstract
Damage assessment applied to reinforced concrete elements is one of the main activities of infrastructure maintenance tasks. Among these elements, the problem of corrosion in reinforced concrete is particularly critical and requires careful consideration. Annually, governments invest a large amount of economic resources in this activity. However, most methodologies for damage assessment rely on visual inspection, which may be subjectively interpreted, producing inconsistent results and requiring a considerable amount of time and resources. This study evaluates the performance of real-time object detection using You Only Look Once, version 3, for detecting corrosion damage in concrete structures. The architecture of YOLOv3 is based on a complex, but efficient, convolutional neural network fed by a dataset proposed and labeled by the authors. Two training stages were established to improve the model precision, using transfer learning with medium- and high-resolution training images. The test results show satisfactory concrete-corrosion detection through validation photographs and videos demonstrating the capabilities of explainable artificial intelligence and its applications in civil engineering.
MoreTranslated text
Key words
artificial intelligence, civil engineering, corrosion damage detection, machine learning, YOLOv3
AI Read Science
Must-Reading Tree
Example
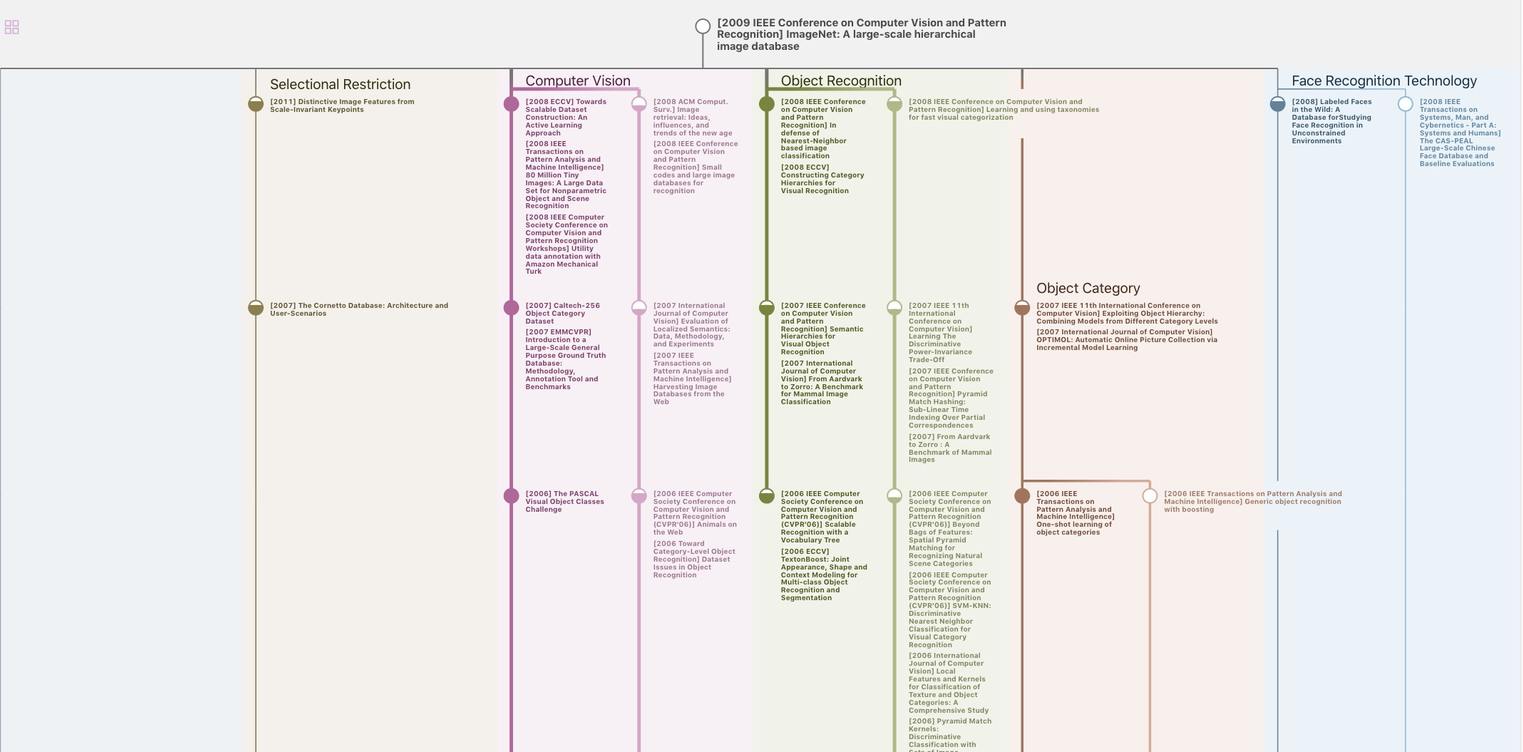
Generate MRT to find the research sequence of this paper
Chat Paper
Summary is being generated by the instructions you defined