Comparative study of deep learning models on the images of biopsy specimens for diagnosis of lung cancer treatment
JOURNAL OF RADIATION RESEARCH AND APPLIED SCIENCES(2023)
摘要
Objective: To compare the application value of different traditional deep learning models in diagnosing and classifying lung cancer. Methods: According to the biopsy samples of our hospital from January 2018 to November 2022, 37 patients treated in this department were selected as the study subjects. Nonsmall and small cell lung cancer biopsy specimens were obtained and stained. Two experienced pathologists diagnosed the biopsy specimens. Multiple in-depth learning methods were used to distinguish between cancer and noncancer biopsies. In this study, we compared the application value of traditional deep learning models in lung cancer diagnosis and Classification. Results: The study tested several popular CNN architectures based on image block classification: AlexNet, VGG, ResNet, and SqueezeNet, comparing two types of training schemes: training from scratch and fine-tuning the entire pretrained network. The AUC of the deep learning model is more reasonable (0. 8808-0. 9121). Except for Resnet-50, the AUC of the training is higher than that of the fine-tuning of the whole network. Conclusion: Deep learning analysis can accelerate the detection speed of whole section images (WSI) and maintain a similar detection rate with pathologists.
更多查看译文
关键词
deep learning models,lung cancer,biopsy specimens
AI 理解论文
溯源树
样例
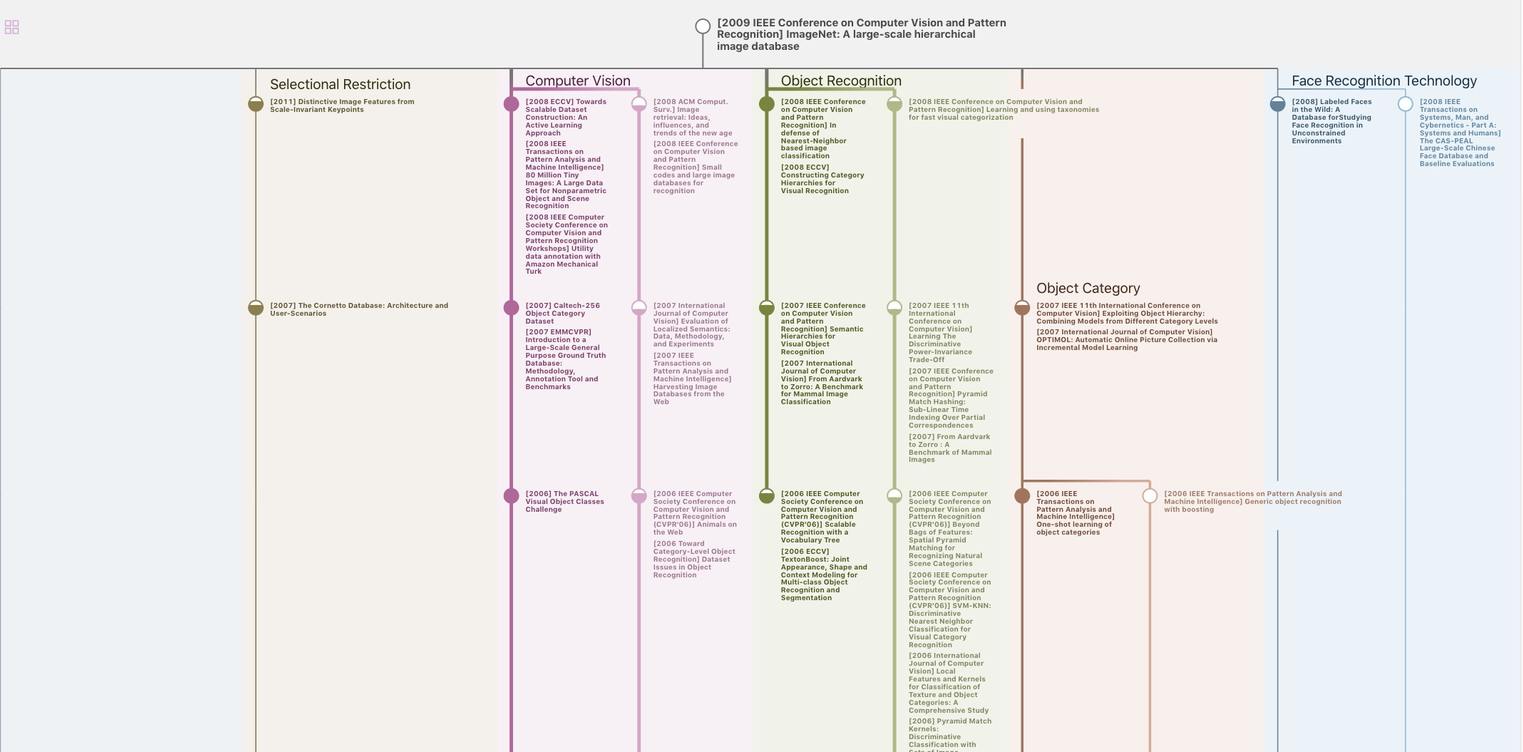
生成溯源树,研究论文发展脉络
Chat Paper
正在生成论文摘要