Time Series Forecasting using LSTM and ARIMA
INTERNATIONAL JOURNAL OF ADVANCED COMPUTER SCIENCE AND APPLICATIONS(2023)
摘要
The series analysis is the process of evaluating sequential data to extract meaningful statistics. In the current era, organizations rely greatly on data analysis to solve and predict possible answers to a specific problem. These predictions help greatly in decision-making. In time series problems, the data is used to train the different machine and deep learning models. The models train on provided data displays particular outcomes. These outcomes anticipate possible solutions. In this paper, the two most effective Python models LSTM (Long Short Term Memory Loss) and ARIMA (Autoregressive integrated moving average) are used. These are the two most recommended models while dealing with Time series forecasting. The selected dataset is from Mulkia Gulf Real Estate available at MarketWatch. The main objective of this research paper is to study and compare the results of the two models used and determine which one is the best-suited model for that particular type of prediction. However, these are widely used models but the focus point of this research is determining the performance variance between these two models. LSTM became famous in 1997 as a training model that can remember patterns based on previous data while ARIMA is famous for forecasting variables of interest using a linear combination of previous values of the variable. The findings state that ARIMA is better for time series forecasting than LSTM based on the mean average of the basic evaluation parameters.
更多查看译文
关键词
lstm,arima
AI 理解论文
溯源树
样例
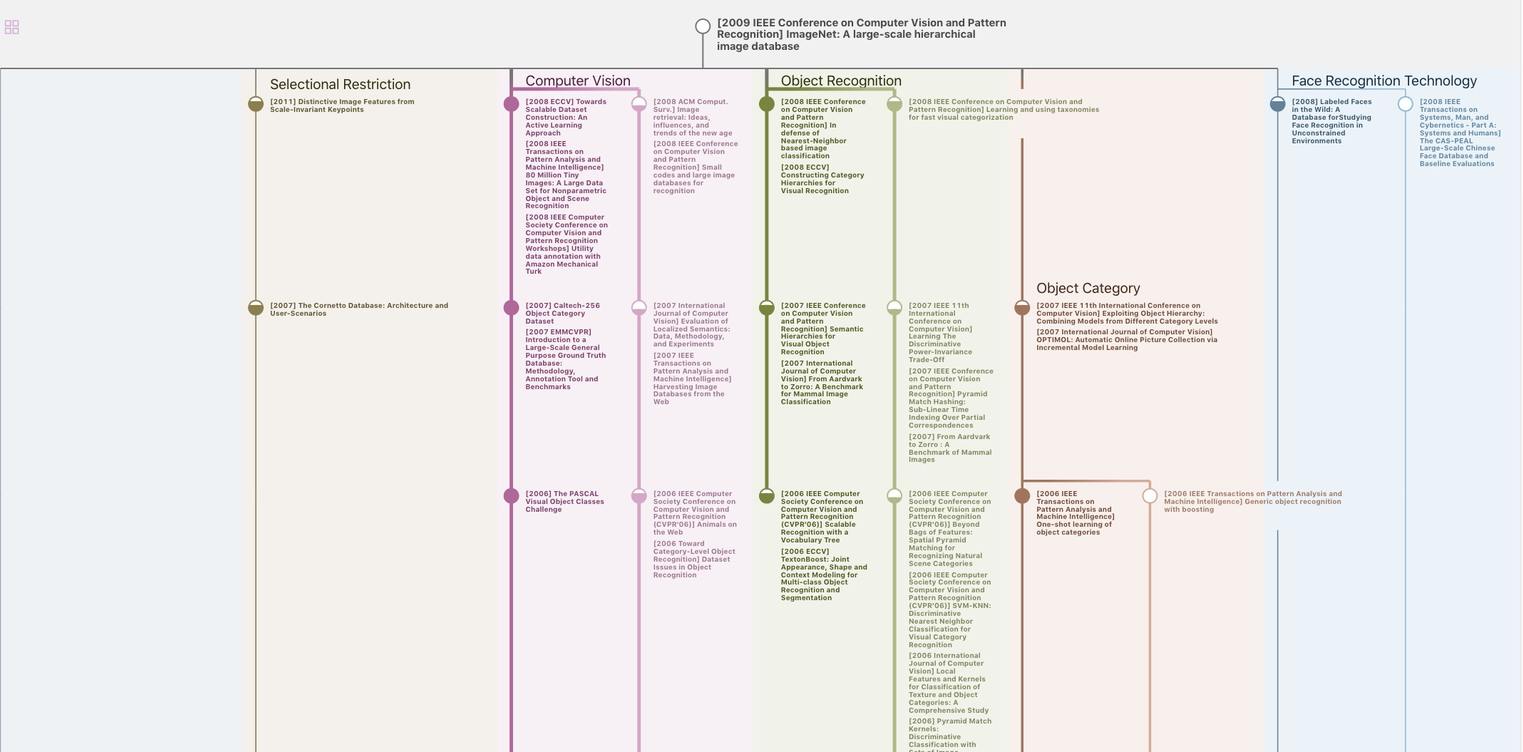
生成溯源树,研究论文发展脉络
Chat Paper
正在生成论文摘要