A Feasible Method for Solving an SDP Relaxation of the Quadratic Knapsack Problem
MATHEMATICS OF OPERATIONS RESEARCH(2024)
摘要
In this paper, we consider a semidefinite programming (SDP) relaxation of the quadratic knapsack problem. After applying low-rank factorization, we get a nonconvex problem, whose feasible region is an algebraic variety with certain good geometric properties, which we analyze. We derive a rank condition under which these two formulations are equivalent. This rank condition is much weaker than the classical rank condition if the coefficient matrix has certain special structures. We also prove that, under an appropriate rank condition, the nonconvex problem has no spurious local minima without assuming linearly independent constraint qualification. We design a feasible method that can escape from nonoptimal nonregular points. Numerical experiments are conducted to verify the high efficiency and robustness of our algorithm as compared with other solvers. In particular, our algorithm is able to solve a one-million-dimensional sparse SDP problem accurately in about 20 minutes on a modest computer.
更多查看译文
关键词
quadratic knapsack problem,Burer and Monteiro method,low-rank SDP,Riemannian optimization
AI 理解论文
溯源树
样例
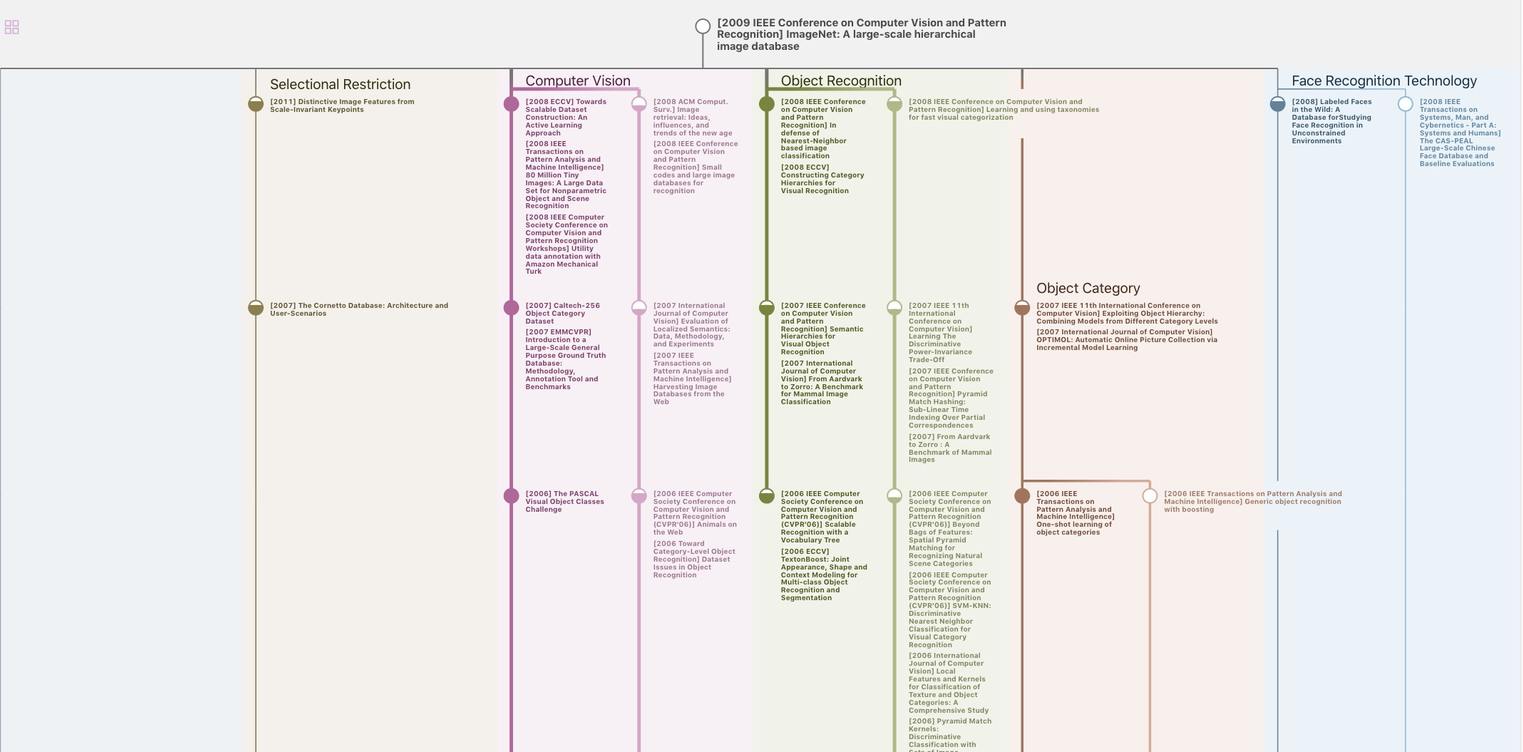
生成溯源树,研究论文发展脉络
Chat Paper
正在生成论文摘要