Symmetric Bayesian Personalized Ranking With Softmax Weight
IEEE TRANSACTIONS ON SYSTEMS MAN CYBERNETICS-SYSTEMS(2023)
摘要
Preference learning, especially pairwise preference learning, is an efficient method for modeling implicit feedback in item recommendation. However, it is insufficient and not always valid to work for a basic pairwise preference model, which assumes that users prefer interacted (i.e., bought or viewed) items to un-interacted (i.e., not bought or not viewed) items. Recently, the state-of-the-art approaches have emerged as two separate but powerful methods, namely, pairwise preferences over item-sets and asymmetric pairwise preference models, respectively, to address limitations of the basic models. In spite of the success achieved by these methods, the assumption that the horizontal pairwise preference is with respect to two items does not always hold in asymmetric pairwise preference. Hence, it is appealing to integrate them into a uniform approach. In this article, we propose a novel symmetric pairwise preference assumption. We use a weighted average through a softmax function and define the overall preferences that can better discover users' preference patterns. With the new assumption and the weighted average method, we propose a novel recommendation algorithm to improve the recommendation quality. Extensive empirical studies show that our new algorithms can significantly outperform several state-of-the-art and baseline methods over a number of public datasets.
更多查看译文
关键词
Implicit feedback,item recommendations,softmax weight,symmetric pairwise preference assumption
AI 理解论文
溯源树
样例
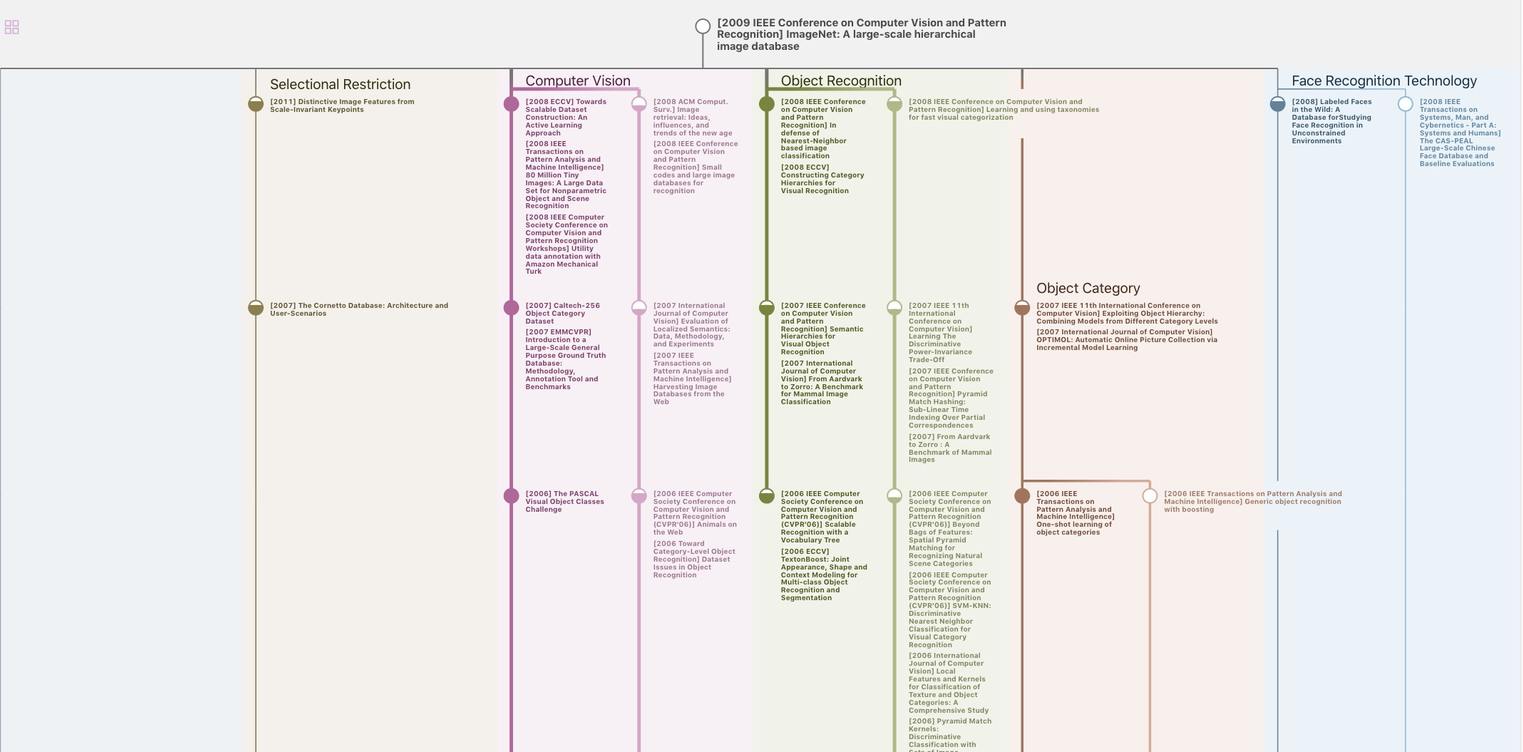
生成溯源树,研究论文发展脉络
Chat Paper
正在生成论文摘要