GAT-EGRU: A Deep Learning Prediction Model for PM2.5 Coupled with Empirical Modal Decomposition Algorithm
JOURNAL OF SYSTEMS SCIENCE AND SYSTEMS ENGINEERING(2023)
Abstract
With the rapid development of the economy and industry and the improvement of pollution monitoring, how to accurately predict PM2.5 has become an issue of concern to the government and society. In the field of PM2.5 pollution forecasting, a series of results have emerged so far. However, in the existing research field of PM2.5 prediction, most studies tend to predict short-term temporal series. Existing studies tend to ignore the temporal and spatial characteristics of PM2.5 transport, which leads to its poor performance in long-term prediction. In this paper, by optimizing previous PM2.5 deep learning prediction models, we propose a model GAT-EGRU. First, we add a spatial modular Graph Attention Network (GAT) and couple an Empirical Modal Decomposition algorithm (EMD), considering the temporal and spatial properties of PM2.5. Then, we use Gated Recurrent Unit (GRU) to filter spatio-temporal features for iterative rolling PM2.5 prediction. The experimental results show that the GAT-EGRU model has more advantages in predicting PM2.5 concentrations, especially for long time steps. This proves that the GAT-EGRU model outperforms other models for PM2.5 forecasting. After that, we verify the effectiveness of each module by distillation experiments. The experimental results show that each model module has an essential role in the final PM2.5 prediction results. The new model improves the ability to predict PM2.5 after a long time accurately and can be used as a practical tool for predicting PM2.5 concentrations.
MoreTranslated text
Key words
Air pollution forecasting,deep learning,spatial-temporal prediction,empirical modal decomposition
AI Read Science
Must-Reading Tree
Example
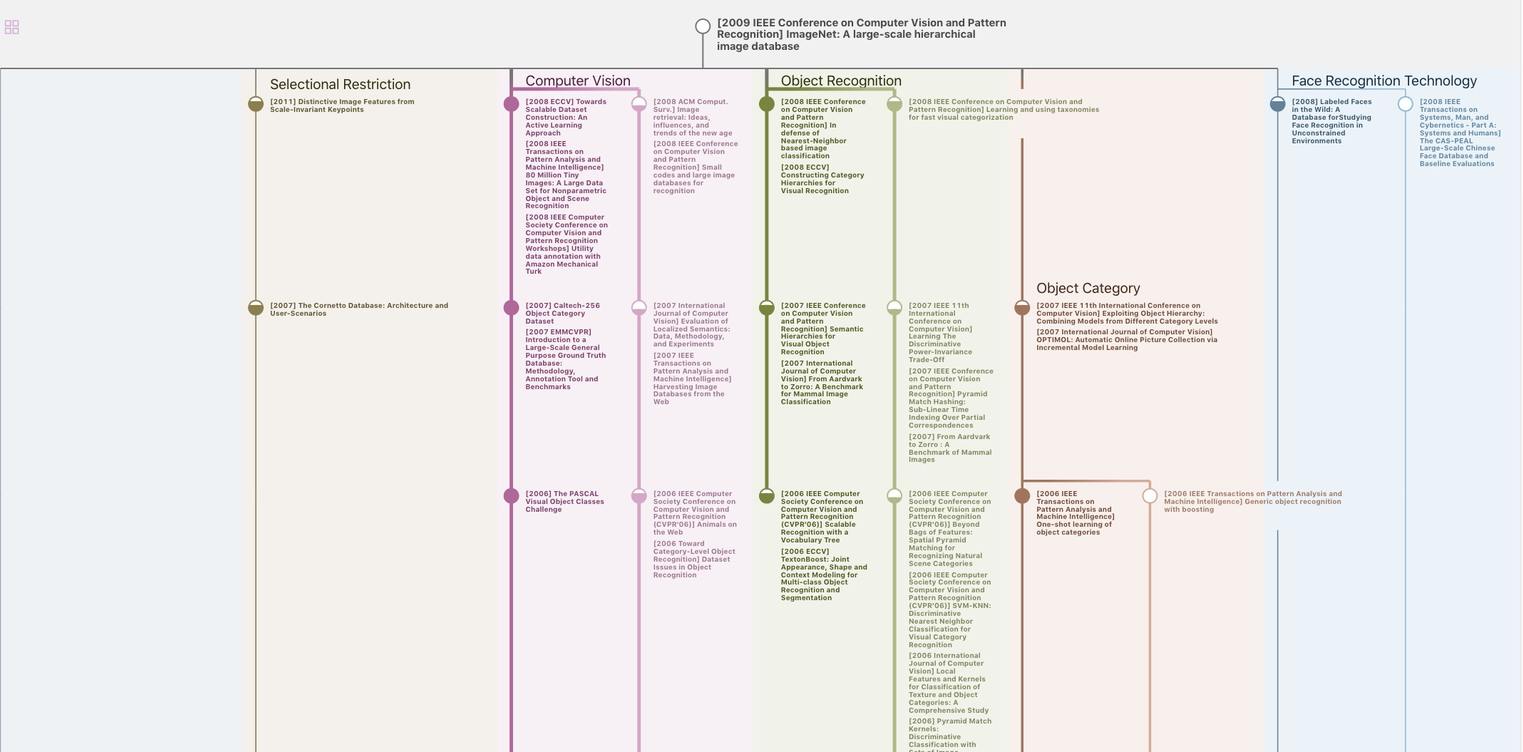
Generate MRT to find the research sequence of this paper
Chat Paper
Summary is being generated by the instructions you defined