Two-stage label distribution learning with label-independent prediction based on label-specific features
KNOWLEDGE-BASED SYSTEMS(2023)
摘要
Label distribution learning (LDL) explicitly models label ambiguity based on the degree to which each label describes an instance. Existing LDL algorithms typically consider all features shared by all class labels. However, irrelevant or redundant information in the feature space can degrade the performance of the model. In this study, a novel two-stage LDL algorithm with label-independent prediction based on label-specific features (TSLIP) is proposed. The first stage combines sparse feature selection and instance correlation constraints. The original high-dimensional input feature space is mapped to a derived low-dimensional label-independent prediction space. The mapping operation not only supports the extraction of label-specific features but also brings the derived space closer to the label distribution space. The second stage employs the Pearson coefficient to explore the label correlation to enhance the performance of the model. Subsequently, the label-independent predictions are normalized to obtain the final label distribution. Extensive experiments are conducted on twelve real-world datasets. The results of the seven measures demonstrate the superiority of our algorithm compared with several state-of-the-art methods.(c) 2023 Elsevier B.V. All rights reserved.
更多查看译文
关键词
Label correlations, Label distribution learning, Label-independent prediction, Label-specific features
AI 理解论文
溯源树
样例
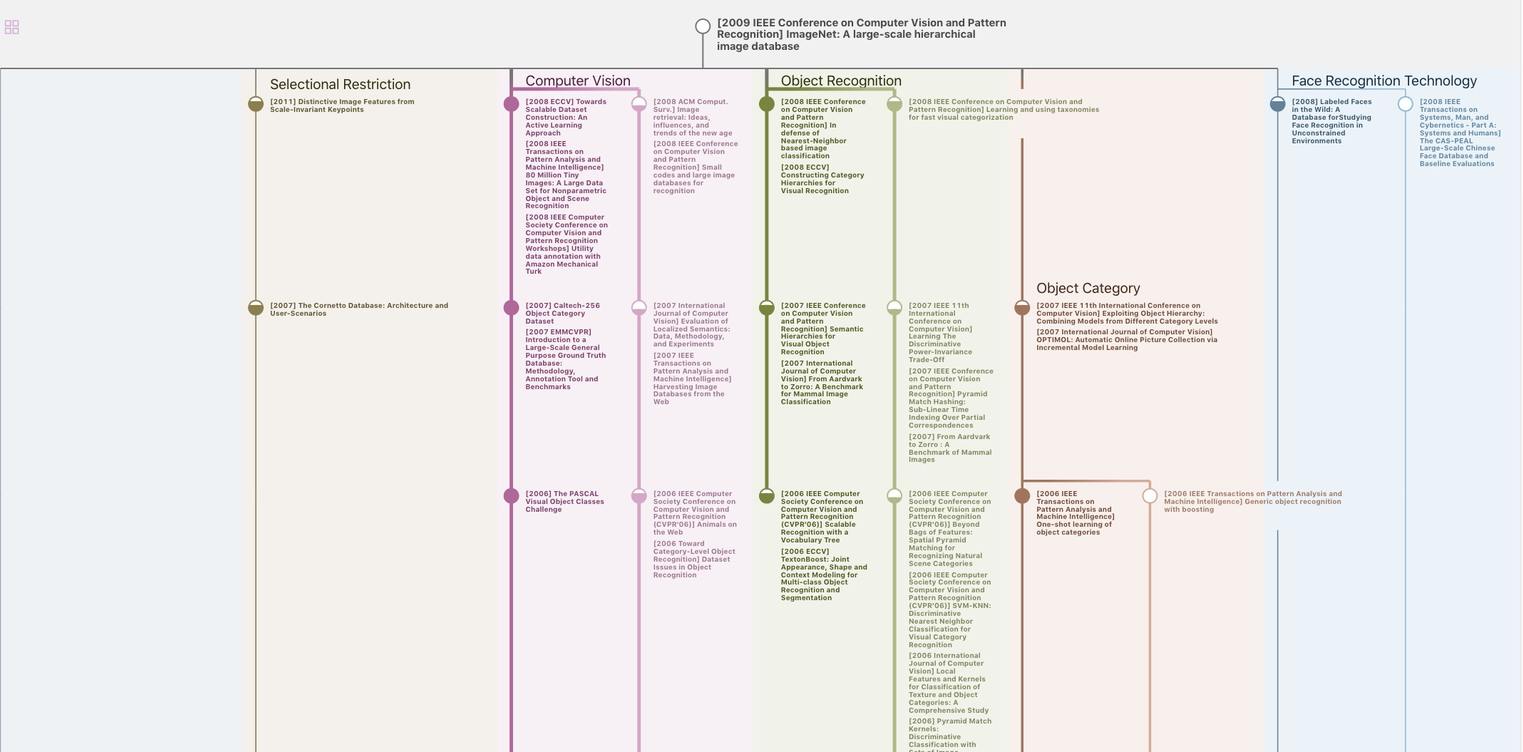
生成溯源树,研究论文发展脉络
Chat Paper
正在生成论文摘要