Social Psychology Inspired Distributed Ledger Technique for Anomaly Detection in Connected Vehicles
IEEE TRANSACTIONS ON INTELLIGENT TRANSPORTATION SYSTEMS(2023)
摘要
Connected Vehicles (CVs), an integral part of the future of intelligent transportation systems, use communication and sensing technologies to communicate among vehicles and infrastructure. However, as vehicles become interconnected, the vulnerability of their components to anomalies and deliberate malicious activity increases. In both cases, it is vital to detect and exclude anomalous data from the decision-making process. While deep learning techniques are gaining popularity for anomaly detection due to their adaptability, they are computationally expensive and require long training times. To overcome this challenge, this paper uses a directed acyclic graph (DAG) based distributed ledger technique and combines it with social psychology principles of ability, integrity, and benevolence to calculate the reputation of vehicles. We introduce the probability of malevolence, a measure of quality, which is a function of the error measurements (between ground truth and reported values) and reputation metrics. We introduce various anomalies such as bias, noise, short, multi-short, drift, multi-drift, stuck-at, and parasite chain attack in the simulated data from the Intelligent Driver Module framework on road topology such as uphill, ring, on-ramp, off-ramp, and road-works to validate the efficacy of the proposed framework in identifying the anomalies. Simulation results show that the malevolence factor serves as an efficient metric for automatically determining the types of anomalies in the CV network.
更多查看译文
关键词
ledger technique,connected vehicles,anomaly detection,social
AI 理解论文
溯源树
样例
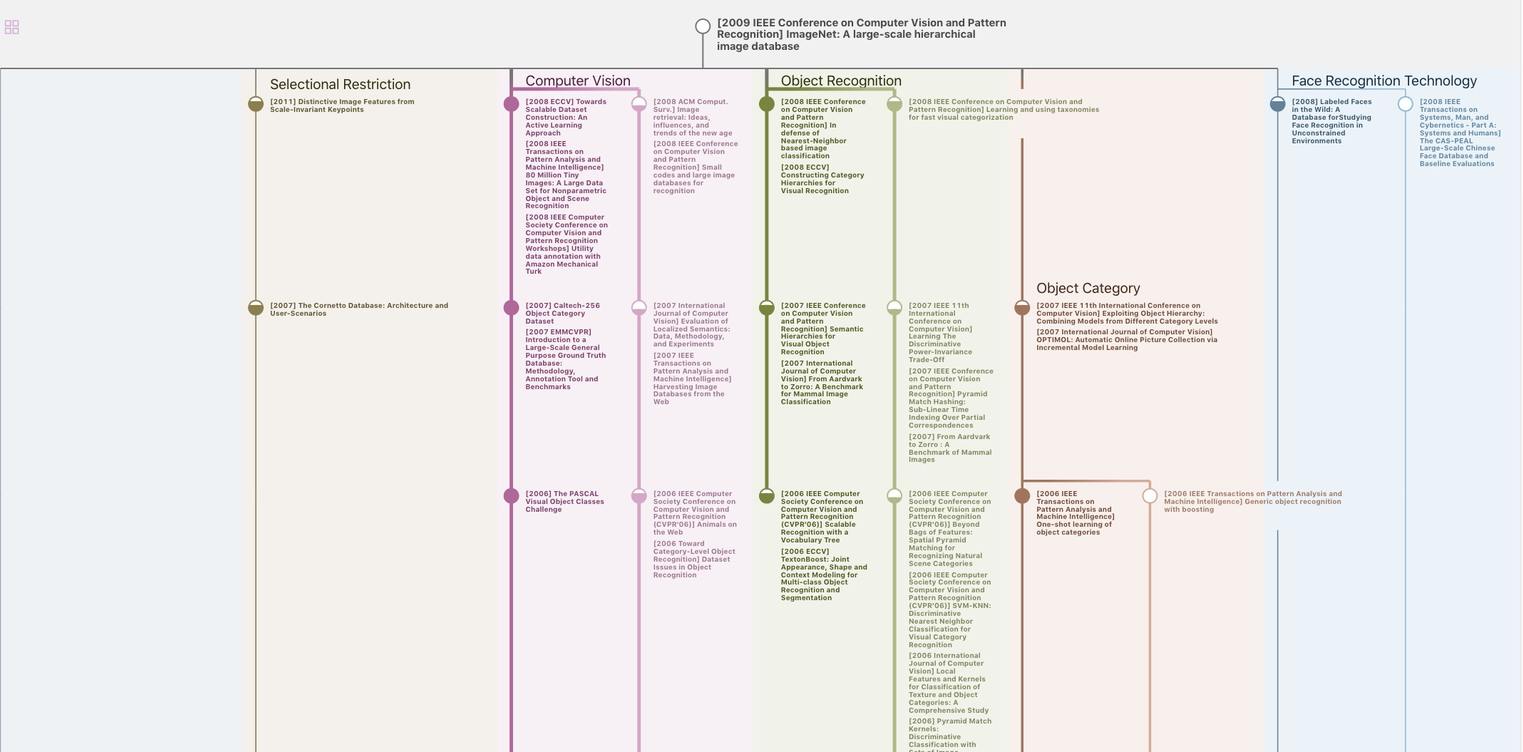
生成溯源树,研究论文发展脉络
Chat Paper
正在生成论文摘要