Efficient machine learning model to predict dynamic viscosity in phosphoric acid production
RESULTS IN ENGINEERING(2023)
摘要
The rheological behavior of the phosphoric acid slurry, during the production process, strongly depends on its dynamic viscosity. Controlling this property limits P2O5 losses, minimizes energy consumption and ensures optimal flow conditions. Thus, reliable simulation tools predicting the viscosity property are needed for analysis and process optimization. To this end, three machine learning (ML) methods: single-layer artificial neural network (ANN), gradient boosting (GB) and random forest (RF), were tested using 456 data of dynamic viscosity at different solid content, shear rate and temperature, obtained from industry. The performance of these models was evaluated and compared using diverse precision metrics. The GB has shown to be the outperforming model with determination coefficient greater than 99%, and Root Mean Squared Error lower than 0.750, on both training and validation datasets. Based on the importance of the explanatory variables, all models agree on the large effect of solid content on dynamic viscosity, followed by shear rate, then temperature. The GB relative partial dependence diagram made it possible to deduce operating intervals for the solid content of the pulp to be fed to the phosphoric acid reactor, leading to optimal flow of the suspension at the level of the attack and maturation units.
更多查看译文
关键词
phosphoric acid production,dynamic viscosity,machine learning
AI 理解论文
溯源树
样例
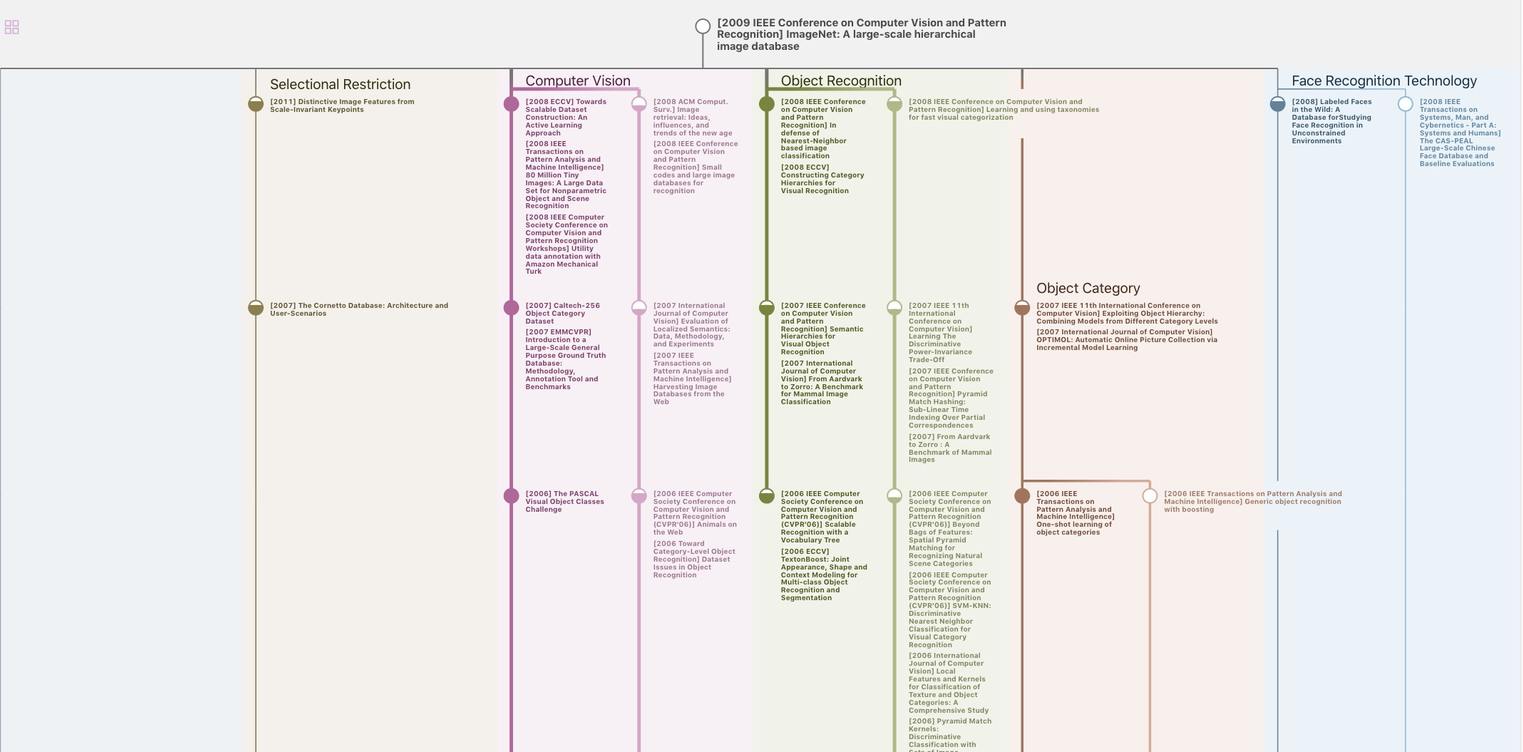
生成溯源树,研究论文发展脉络
Chat Paper
正在生成论文摘要