Rheological characterisation of water atomised tool steel powders developed for laser powder bed fusion by supervised and unsupervised machine learning
POWDER METALLURGY(2023)
摘要
Metal powders developed for additive manufacturing processes need to achieve specific flow characteristics to be considered suitable. However, for the relationship between powder flow and the morphological characteristics of individual particles can be difficult to establish. In this context, artificial intelligence appears to be the perfect tool to clarify the imprecision surrounding this type of interaction. The work summarised in this manuscript first uses a neural network architecture (Mask R-CNN) allowing the segmentation of individual water-atomised tool steel particles in micrographs acquired in scanning electron microscopy. The micrographs of individual particles or their shape descriptors are then processed using and comparing two different strategies, namely linear regression or unsupervised machine learning (ML), to corelate the information collected on individual particles with the rheological properties of powder specimens. The approach developed aims to acquire new knowledge regarding specific particle characteristics that are required to optimise powder flowability for laser powder-bed fusion.
更多查看译文
关键词
Water atomisation, tool steel, laser powder bed fusion, rheological characterisation, Particle morphologies, machine learning, Roughness, Cohesive index
AI 理解论文
溯源树
样例
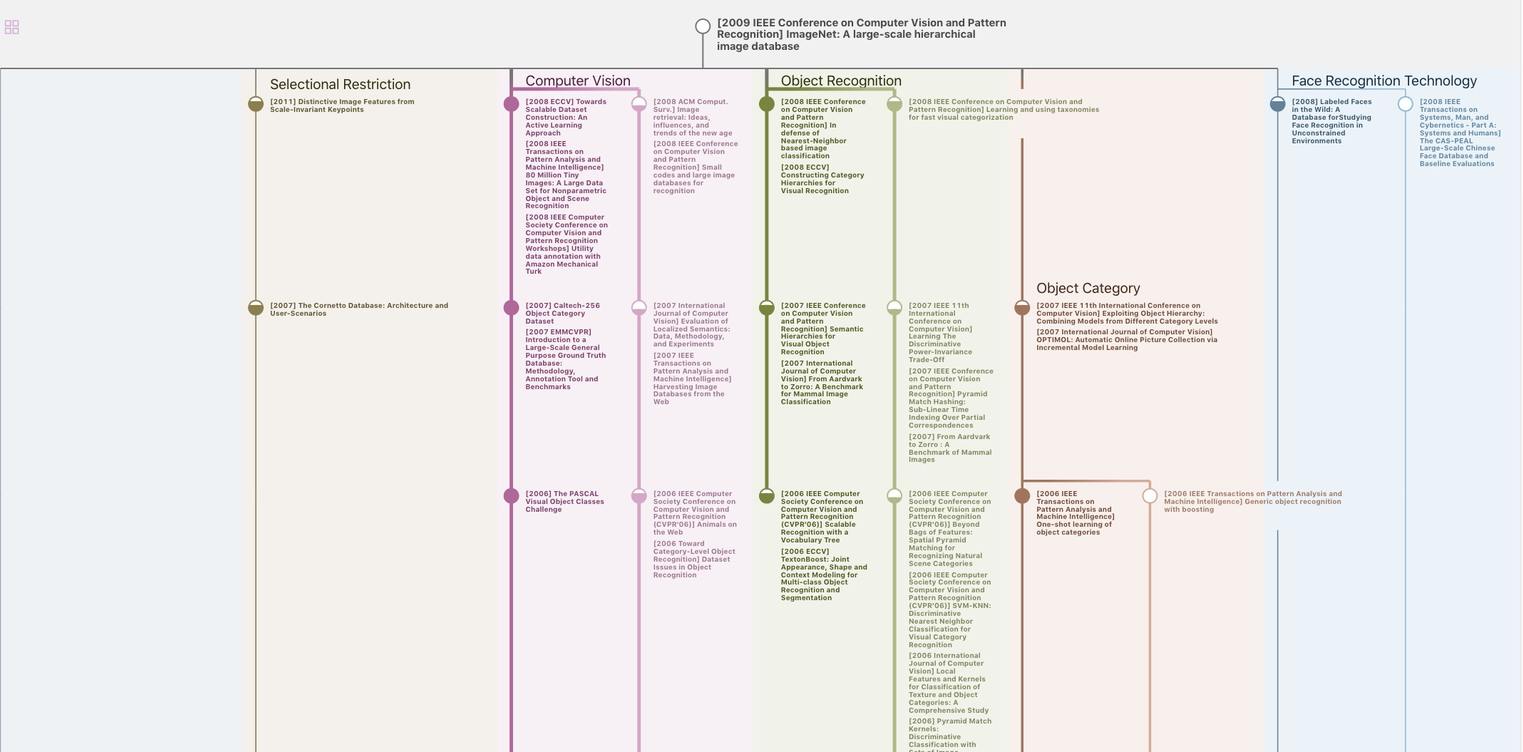
生成溯源树,研究论文发展脉络
Chat Paper
正在生成论文摘要