Optimal planning of Electricity-Hydrogen hybrid energy storage system considering demand response in active distribution network
ENERGY(2023)
摘要
With the increasing penetration of distributed generation (DG) based on renewable energy in active distribution networks (ADN), net load and voltage fluctuation has greatly worsened. Demand response (DR) load and energy storage systems (ESSs) are regarded as significant resources of ADN, owing to their critical role in increasing stability. This study establishes a novel planning bi-level programming model (including an upper-level model and a lower-level model) of the electricity-hydrogen hybrid (EHH)-ESS considering the DR load to enhance ADN stability. The upper-level model determines the optimal time-of-use (ToU) price formulation strategy to minimise the net load fluctuation and maximise user electricity purchasing cost satisfaction and electricity consumption. The lower-level model considers the life cycle cost (LCC) of the EHH-ESS, voltage fluctuation, and net load fluctuation under DR as objectives to optimise the locations, capacities, and charging/discharging power of the EHH-ESS. A multi-objective mayfly algorithm (MOMA) based on improved grey-target decision-making (IGTDM) was developed to optimise the aforementioned models. The validity and feasibility of the proposed models and methods were comprehensively validated based on extended IEEE 33 and IEEE 66 bus systems. Simulation re-sults based on MOMA-IGTDM under the extended IEEE 66 bus system demonstrate that compared with only accessing EHH-ESS, although LCC is increased by 2.79% (from $ 1.0217 e+3/to $ 1.0502e+3/) by considering the DR load and configuring EHH-ESS, the net load fluctuation and voltage fluctuation can be reduced by 20.88% (from 5.7754 MW to 4.5697 MW) and 27.40% (from 0.2606 p.u. to 0.1892 p.u.), respectively. It is verified that the configuration of the EHH-ESS and DR can significantly improve the stability of the ADN.
更多查看译文
关键词
distribution network,demand response,optimal planning
AI 理解论文
溯源树
样例
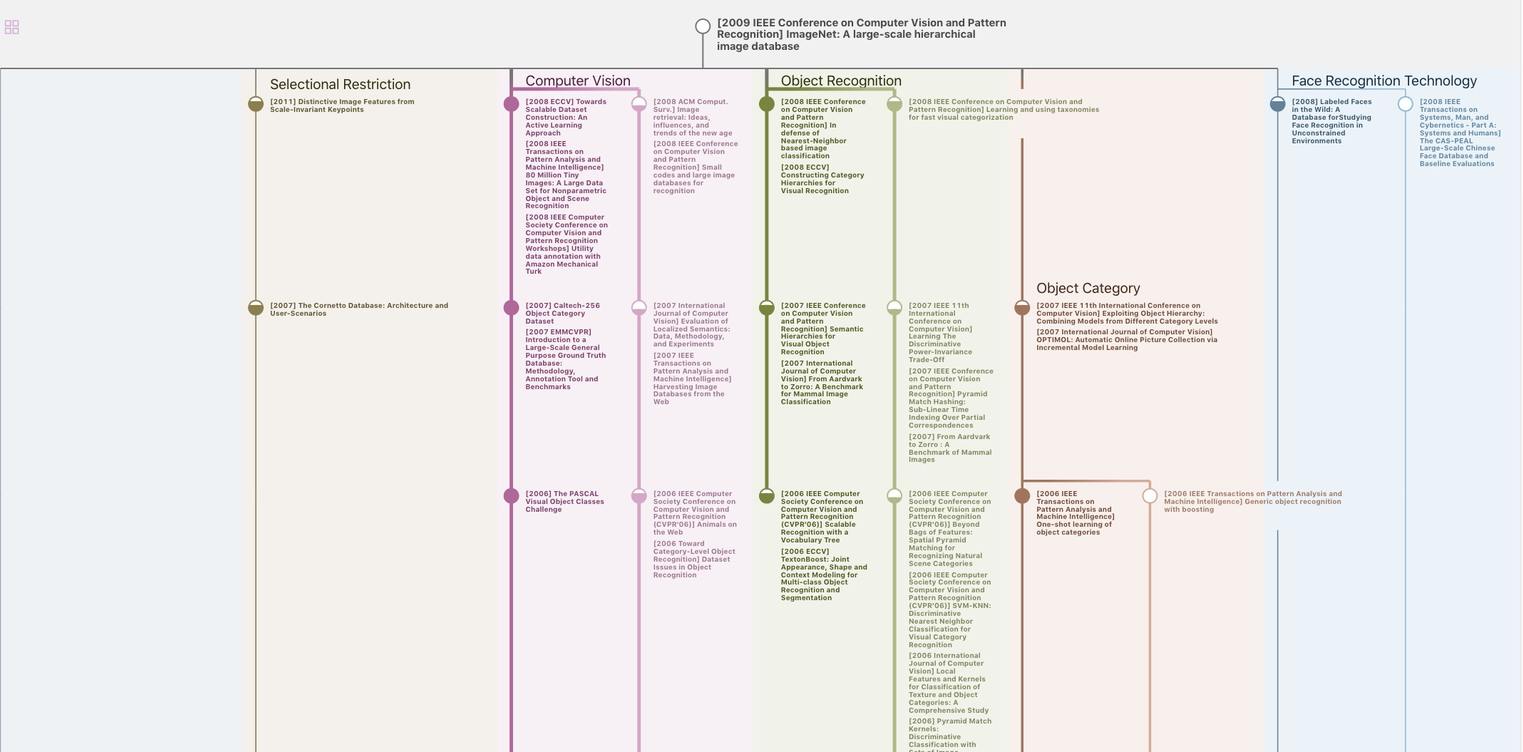
生成溯源树,研究论文发展脉络
Chat Paper
正在生成论文摘要