Dynamic Transition Graph for Estimating the Predictability of Financial and Economical Processes
COMPLEX NETWORKS AND THEIR APPLICATIONS XI, COMPLEX NETWORKS 2022, VOL 1(2023)
摘要
The problem of time series predictability estimation often appears when one deals with the task of forecasting financial and economical processes, especially when the processes are not sustainable and presume critical transitions and/or behaviour changes in the generating complex dynamical system. In these cases it is important to notice the moment when transitions/changes start and to distinguish their direction as soon as possible in order to adjust the forecasting algorithm or, at least, properly evaluate the forecast accuracy. To deal with such effects, we propose a dynamic transition graph-based method for real-time incremental tracing of the changes in the predictability of time series describing financial and economical processes. Our method helps to filter some "noise" time series information and emphasize the significant aspects of the corresponding dynamical system behavior. Besides, we use several graph features such as centrality degree, number and size of loops, connectivity and entropy to evaluate the predictability. We also construct a graph neural network classifier and train it on specific artificial time series datasets to efficiently classify real-world time series by predictability in a real-time incremental tracing manner.
更多查看译文
AI 理解论文
溯源树
样例
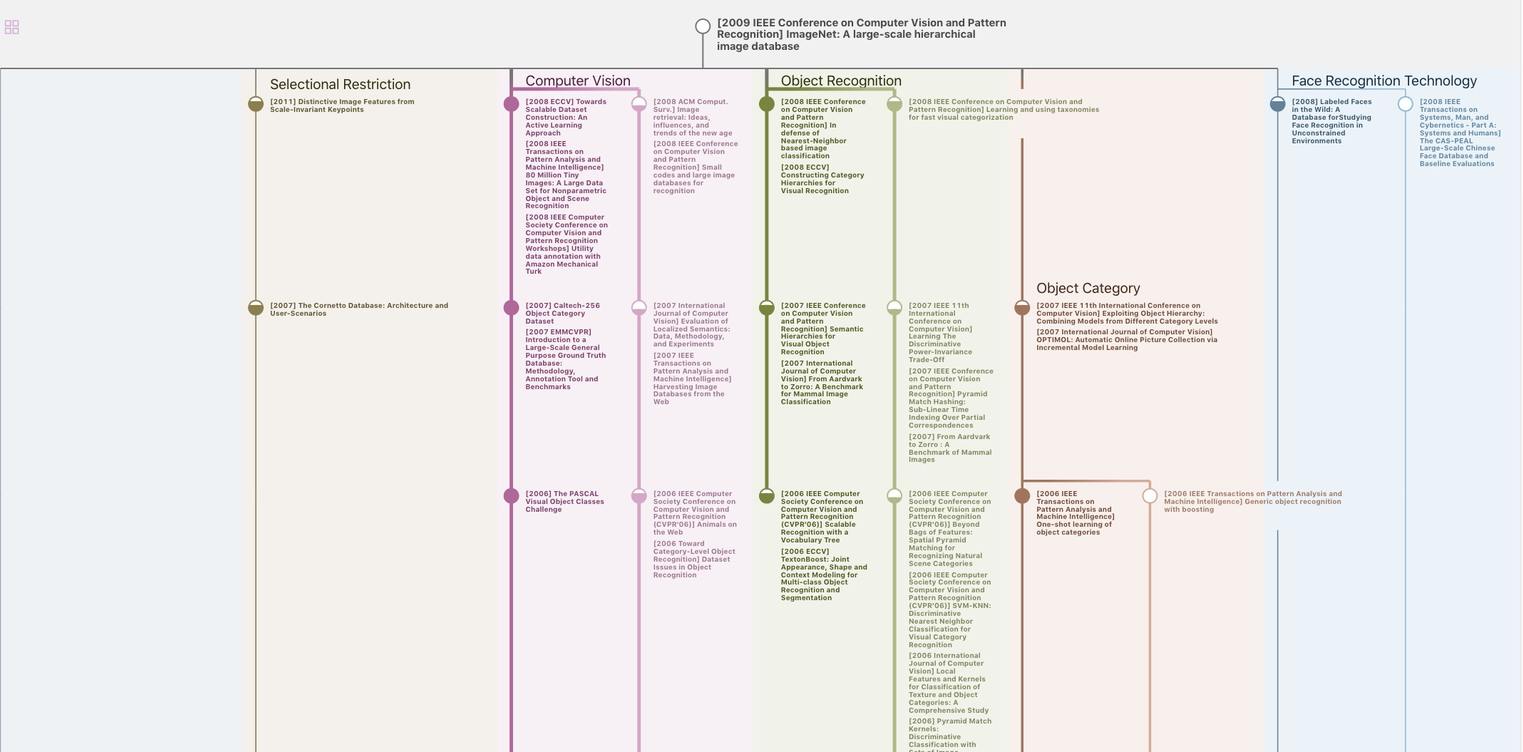
生成溯源树,研究论文发展脉络
Chat Paper
正在生成论文摘要