Inapproximability of matrix p?q norms
SIAM JOURNAL ON COMPUTING(2023)
摘要
We study the problem of computing the p \rightarrow q norm of a matrix A \in Rm\times n, defined as \| A\| p\rightarrow q = maxx\in Rn\setminus \{ 0\} \| x\| p. This problem generalizes the spectral norm of a matrix (p = q = 2) and the Grothendieck problem (p = \infty , q = 1) and has been widely studied in various regimes. When p \geq q, the problem exhibits a dichotomy: constant factor approximation algorithms are known if 2 \in [q, p], and the problem is hard to approximate within almost polynomial factors when 2 \in/ [q, p]. The regime when p < q, known as hypercontractive norms, is particularly significant for various applications but much less well understood. The case with p = 2 and q > 2 was studied by 307--326], who gave subexponential algorithms for a promise version of the problem (which captures small-set expansion) and also proved hardness of approximation results based on the exponential time hypothesis. However, no NP-hardness of approximation is known for these problems for any p < q. We prove the first NP-hardness result (under randomized reductions) for approximating hypercontractive norms. We show that for any 1 < p < q < \infty with 2 \in/ [p, q], \| A\| p\rightarrow q is hard to approximate within 2O((log n)1 - \epsilon ) assuming NP \subseteq \not BPTIME(2(logn)O(1) ). En route to the above result, we also prove almost tight results for the case when p \geq q with 2 \in [q, p].
更多查看译文
关键词
operator norms, continuous optimization, inapproximability
AI 理解论文
溯源树
样例
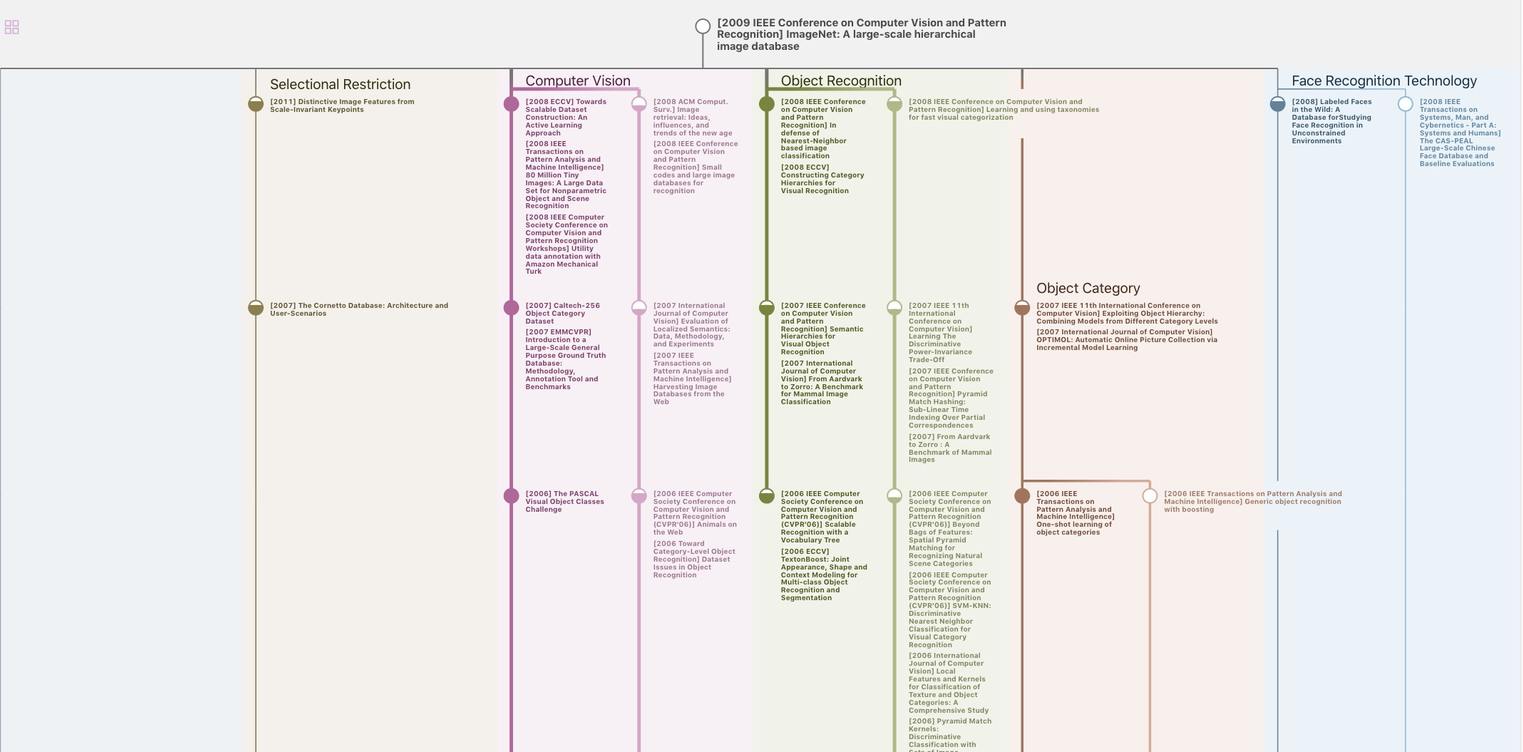
生成溯源树,研究论文发展脉络
Chat Paper
正在生成论文摘要