Task-specific speech enhancement and data augmentation for improved multimodal emotion recognition under noisy conditions
FRONTIERS IN COMPUTER SCIENCE(2023)
摘要
Automatic emotion recognition (AER) systems are burgeoning and systems based on either audio, video, text, or physiological signals have emerged. Multimodal systems, in turn, have shown to improve overall AER accuracy and to also provide some robustness against artifacts and missing data. Collecting multiple signal modalities, however, can be very intrusive, time consuming, and expensive. Recent advances in deep learning based speech-to-text and natural language processing systems, however, have enabled the development of reliable multimodal systems based on speech and text while only requiring the collection of audio data. Audio data, however, is extremely sensitive to environmental disturbances, such as additive noise, thus faces some challenges when deployed "in the wild." To overcome this issue, speech enhancement algorithms have been deployed at the input signal level to improve testing accuracy in noisy conditions. Speech enhancement algorithms can come in different flavors and can be optimized for different tasks (e.g., for human perception vs. machine performance). Data augmentation, in turn, has also been deployed at the model level during training time to improve accuracy in noisy testing conditions. In this paper, we explore the combination of task-specific speech enhancement and data augmentation as a strategy to improve overall multimodal emotion recognition in noisy conditions. We show that AER accuracy under noisy conditions can be improved to levels close to those seen in clean conditions. When compared against a system without speech enhancement or data augmentation, an increase in AER accuracy of 40% was seen in a cross-corpus test, thus showing promising results for "in the wild" AER.
更多查看译文
关键词
multimodal emotion recognition, BERT based text features, modulation spectrum features, data augmentation, speech enhancement, context-awareness
AI 理解论文
溯源树
样例
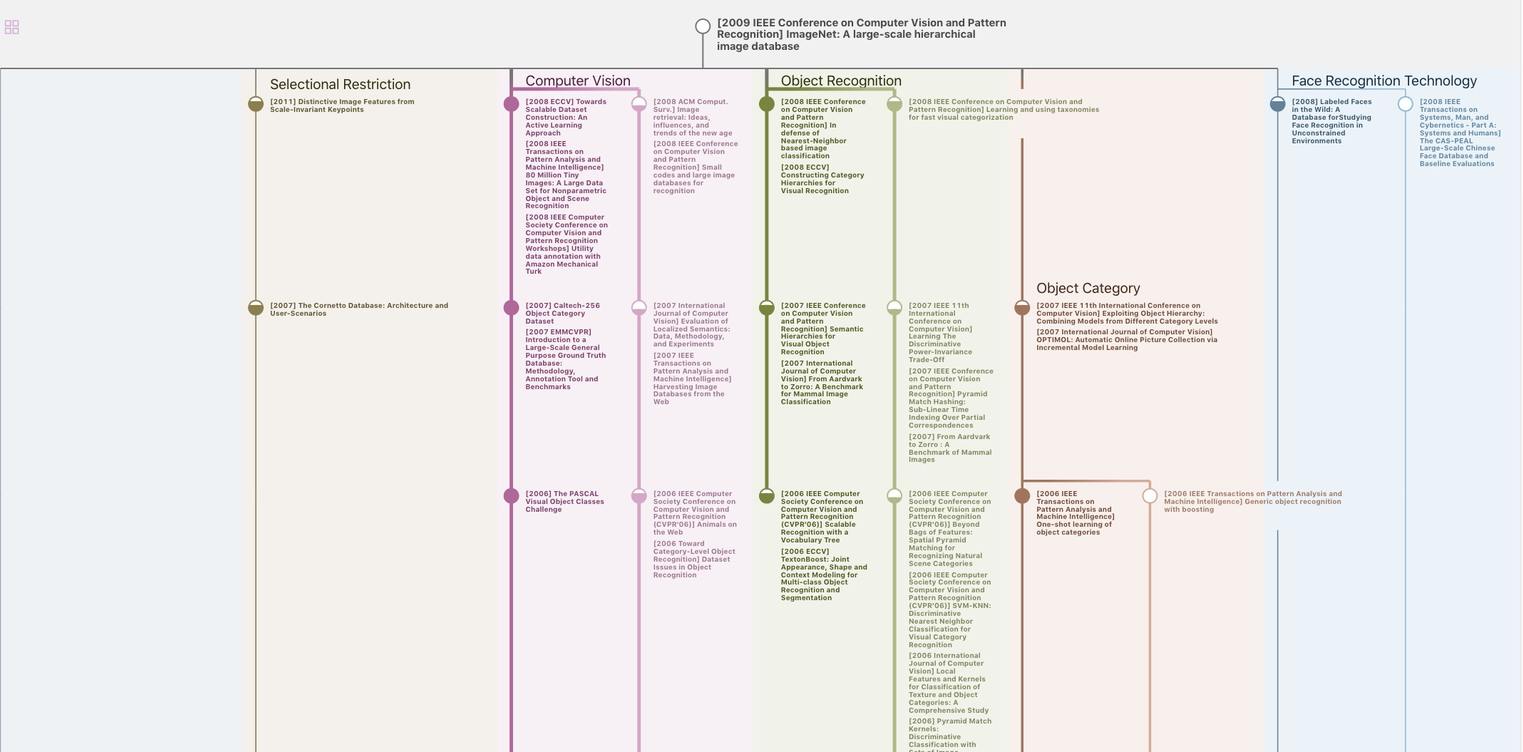
生成溯源树,研究论文发展脉络
Chat Paper
正在生成论文摘要