Detection of tool wear during machining by designing a novel 12-way 2-shot learning model by applying L2-regularization and image augmentation
The International Journal of Advanced Manufacturing Technology(2023)
摘要
Tool wear monitoring is regarded as an incredibly important aspect of improving the surface integrity of machined components in the manufacturing sector. This research study performed operations using twelve different types of drilling and milling tools. The worn tools ranging from grade-1 to grade-5 were categorized based on tool wear severity by measuring the flank wear land width of each tool. Advanced algorithms were designed based on short-time Fourier transform and continuous wavelet transform to convert time-series force signals’ data into spectrogram and scalogram images, respectively, to increase the number of shots with which the model can work based on the methodology of 2-shot learning. An algorithm for image augmentation was developed to increase the number of images to improve the training and overall performance of the model. L2 regularization along with the optimal hyper-parameters were utilized to avoid overfitting and to improve the model’s efficiency. Hyper-parameters were optimized by using the grid-search methodology. The milling and drilling data was collated into 12 classes which resulted in a 12-way learning model. Therefore, it will work for both milling and drilling operations. The model will determine whether the test tool is normal or worn. And if worn, it will determine the severity level of tool wear ranging from grade-1 to grade-5. The final results have shown that the model has worked efficiently during CNC machining and achieved 87.83% accuracy.
更多查看译文
关键词
Tool wear grade,Drilling,Milling,Accuracy,12-way 2-shot learning
AI 理解论文
溯源树
样例
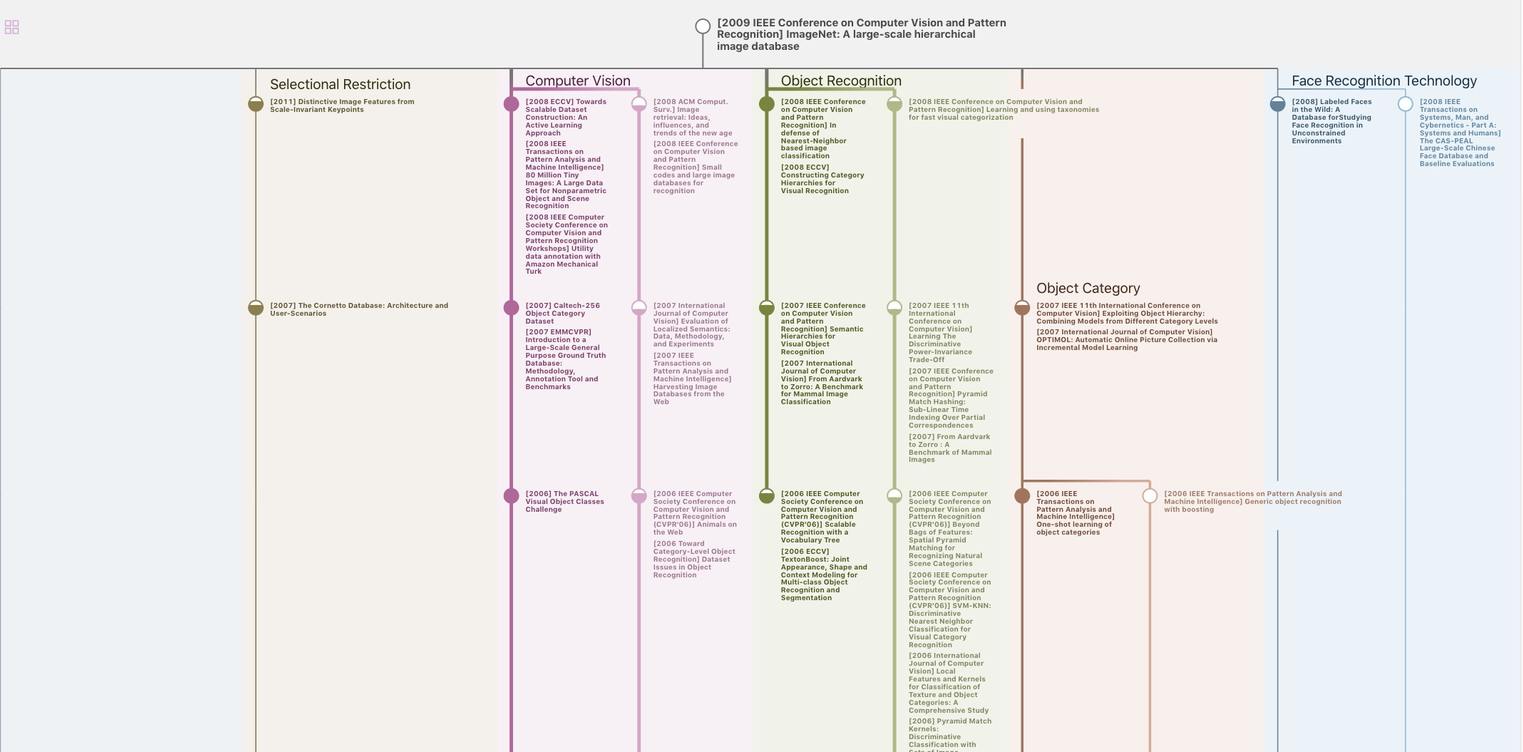
生成溯源树,研究论文发展脉络
Chat Paper
正在生成论文摘要