Digital twin-long short-term memory (LSTM) neural network based real-time temperature prediction and degradation model analysis for lithium-ion battery
JOURNAL OF ENERGY STORAGE(2023)
摘要
Real-time temperature prediction is essential to circumvent thermal safety issues for lithium-ion batteries (LIB). However, its industrial applications are challenging due to operating temperature, voltage range, capacity degradation, and current rate (C-rate) variations. This study proposes a digital twin (DT) technology and long short-term memory (LSTM) neural network-based method for real-time temperature prediction and degradation pattern analysis. The DT model is established based on lumped thermal equivalent circuits to describe the dy-namic thermal behavior of LIB and identify the parameters by calculations. Furthermore, the real-time tem-perature prediction framework considering voltage, current, and operating temperature is further designed following identifying results, which consists of indicators extraction, correlation analysis, LSTM neural network, and DT model four parts. In addition, the incremental capacity analysis (ICA) method is used to analyze LIB degradation patterns. The experimental results indicate that the primary degradation pattern of the experimental sample is loss of lithium inventory (LLI), and the loss of active material (LAM) appears after 600 cycles. Moreover, the maximum error and root mean square error (RMSE) of the temperature prediction framework is 0.31 degrees C and 0.17 degrees C under the constant current (CC) discharging condition, 0.85 degrees C and 0.47 degrees C under the dynamic discharging condition, respectively. The results demonstrate that the proposed real-time temperature prediction framework delivers acceptable accuracy for CC discharging and dynamic discharging conditions, which can complete the requirements of practical applications. This study dramatically reduces the response time of temperature prediction and guides optimizing battery thermal management systems (BTMS).
更多查看译文
关键词
Energy storage,Digital twin,Neural network,Real-time temperature prediction,Degradation mode
AI 理解论文
溯源树
样例
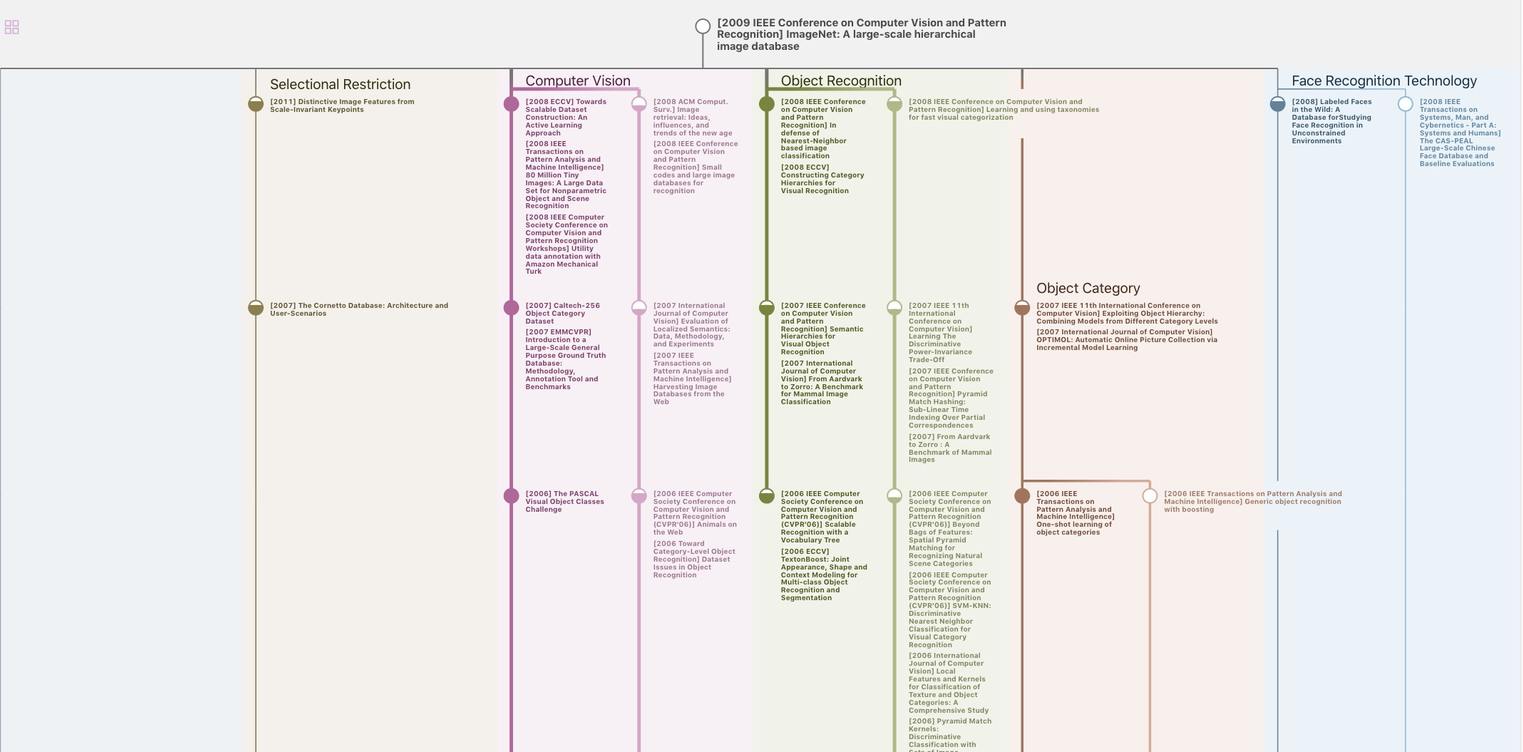
生成溯源树,研究论文发展脉络
Chat Paper
正在生成论文摘要