Short-term load forecasting based on AM-CIF-LSTM method adopting transfer learning
FRONTIERS IN ENERGY RESEARCH(2023)
摘要
Aiming at the unreliability of historical data for short-term load forecasting caused by the sudden change of power grid load under emergencies, a short-term load prediction method adopting transfer learning is studied. The proposed transfer learning method combines the attention mechanism (AM) with the long short-term memory network coupled with input and forgetting gates (CIF-LSTM) to construct the AM-CIF-LSTM short-term load prediction model. First, the variational modal decomposition (VMD) method is used to extract the trend component and certain periodic high-frequency components of the load datasets of the scene to be predicted and similar scenes. Subsequently, the AM-encoder/decoder learning model is established based on the trend component, and the AM learnable parameters are trained and transferred to the AM-CIF-LSTM model. Furthermore, inspired by the idea of classified forecasting, the load trend component and periodic high-frequency components under the required prediction scene are predicted by AM-CIF-LSTM and deep recursive neural network (DRNN), respectively. Finally, the load forecasting results are superimposed to obtain the load forecasting value. The experimental results demonstrate that the proposed method outperformed the existing methods in multiple accuracy indicators and could predict the rapid change trend of load in the case of insufficient data accurately and stably.
更多查看译文
关键词
learning,load,short-term,am-cif-lstm
AI 理解论文
溯源树
样例
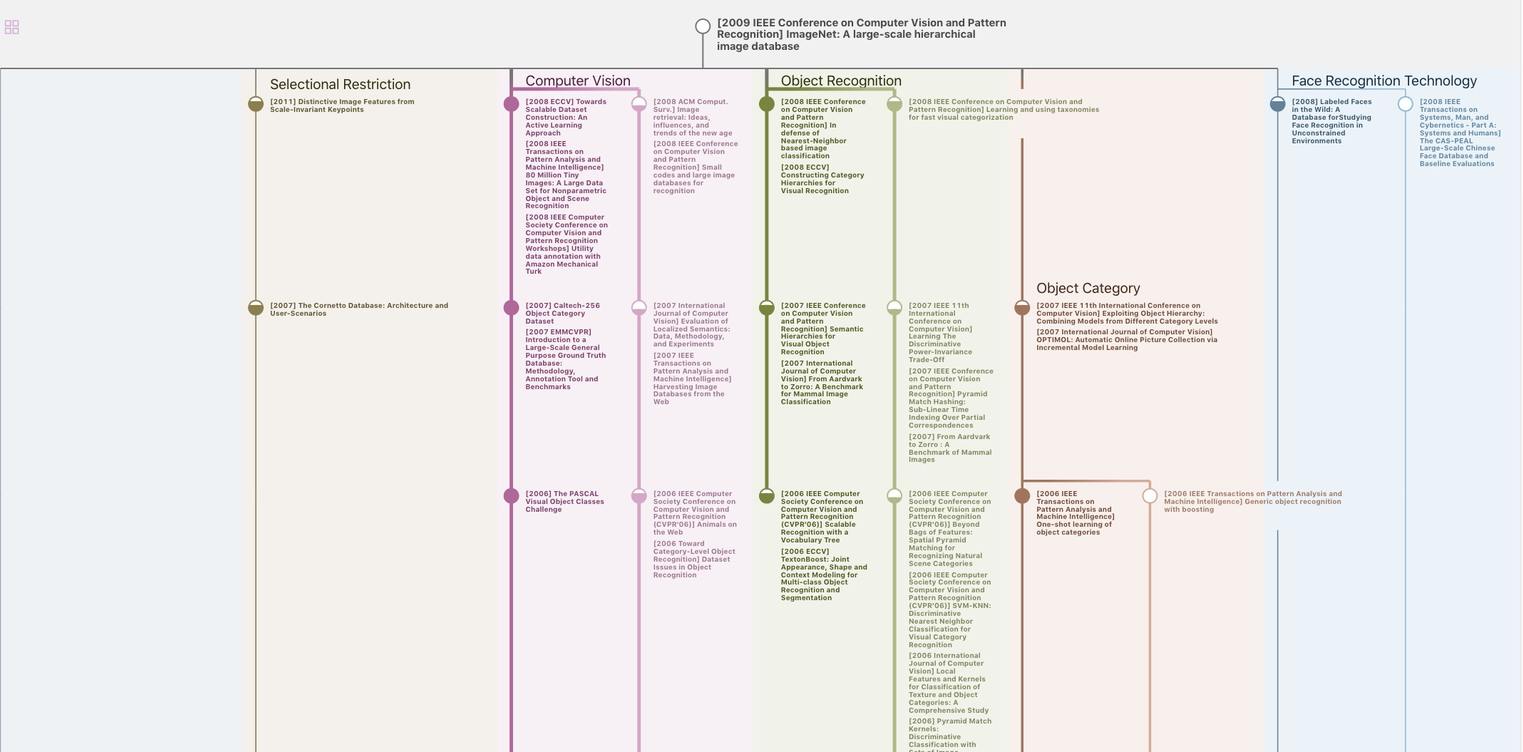
生成溯源树,研究论文发展脉络
Chat Paper
正在生成论文摘要