Learning Equilibria in Asymmetric Auction Games
INFORMS JOURNAL ON COMPUTING(2023)
摘要
Computing Bayesian Nash equilibrium strategies in auction games is a challenging problem that is not well-understood. Such equilibria can be modeled as systems of nonlinear partial differential equations. It was recently shown that neural pseudogradient ascent (NPGA), an implementation of simultaneous gradient ascent via neural networks, converges to a Bayesian Nash equilibrium for a wide variety of symmetric auction games. Whereas symmetric auction models are widespread in the theoretical literature, in most auction markets in the field, one can observe different classes of bidders having different valuation distributions and strategies. Asymmetry of this sort is almost always an issue in real-world multiobject auctions, in which different bidders are interested in different packages of items. Such environments require a different implementation of NPGA with multiple interacting neural networks having multiple outputs for the different allocations in which the bidders are interested. In this paper, we analyze a wide variety of asymmetric auction models. Interestingly, our results show that we closely approximate Bayesian Nash equilibria in all models in which the analytical Bayes-Nash equilibrium is known. Additionally, we analyze new and larger environments for which no analytical solution is known and verify that the solution found approximates equilibrium closely. The results provide a foundation for generic equilibrium solvers that can be used in a wide range of auction games.
更多查看译文
关键词
equilibrium learning, neural networks, Bayes-Nash equilibria
AI 理解论文
溯源树
样例
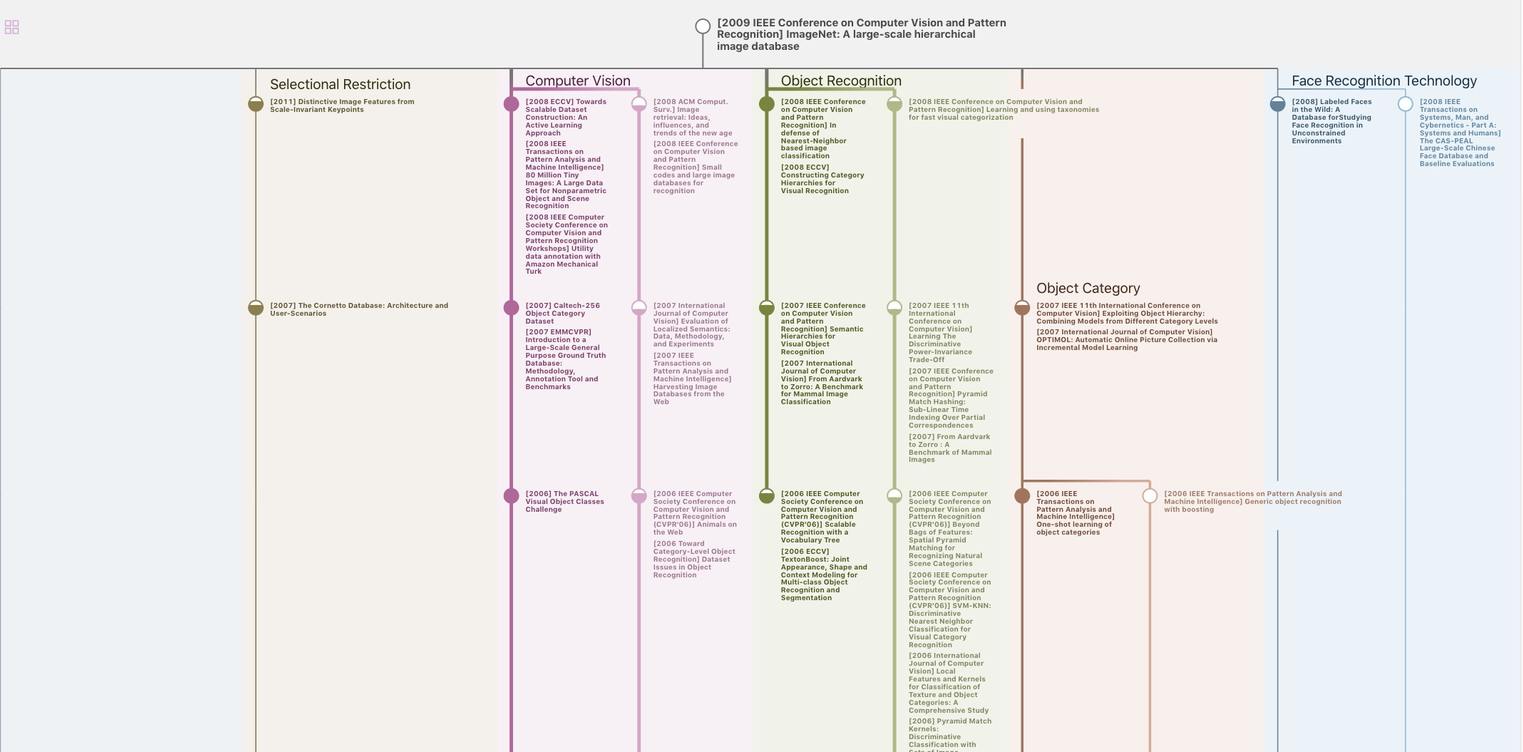
生成溯源树,研究论文发展脉络
Chat Paper
正在生成论文摘要