Real-time self-adaptive Q-learning controller for energy management of conventional autonomous vehicles
EXPERT SYSTEMS WITH APPLICATIONS(2023)
摘要
Reducing emissions and energy consumption of autonomous vehicles is critical in the modern era. This paper presents an intelligent energy management system based on Reinforcement Learning (RL) for conventional autonomous vehicles. Furthermore, in order to improve the efficiency, a new exploration strategy is proposed to replace the traditional decayed epsilon-greedy strategy in the Q-learning algorithm associated with RL. Unlike tradi-tional Q-learning algorithms, the proposed self-adaptive Q-learning (SAQ-learning) can be applied in real-time. The learning capability of the controllers can help the vehicle deal with unknown situations in real-time. Nu-merical simulations show that compared to other controllers, Q-learning and SAQ-learning controllers can generate the desired engine torque based on the vehicle road power demand and control the air/fuel ratio by changing the throttle angle efficiently in real-time. Also, the proposed real-time SAQ-learning is shown to improve the operational time by 23% compared to standard Q-learning. Our simulations reveal the effectiveness of the proposed control system compared to other methods, namely dynamic programming and fuzzy logic methods.
更多查看译文
关键词
energy management,conventional autonomous vehicles,real-time,self-adaptive,q-learning
AI 理解论文
溯源树
样例
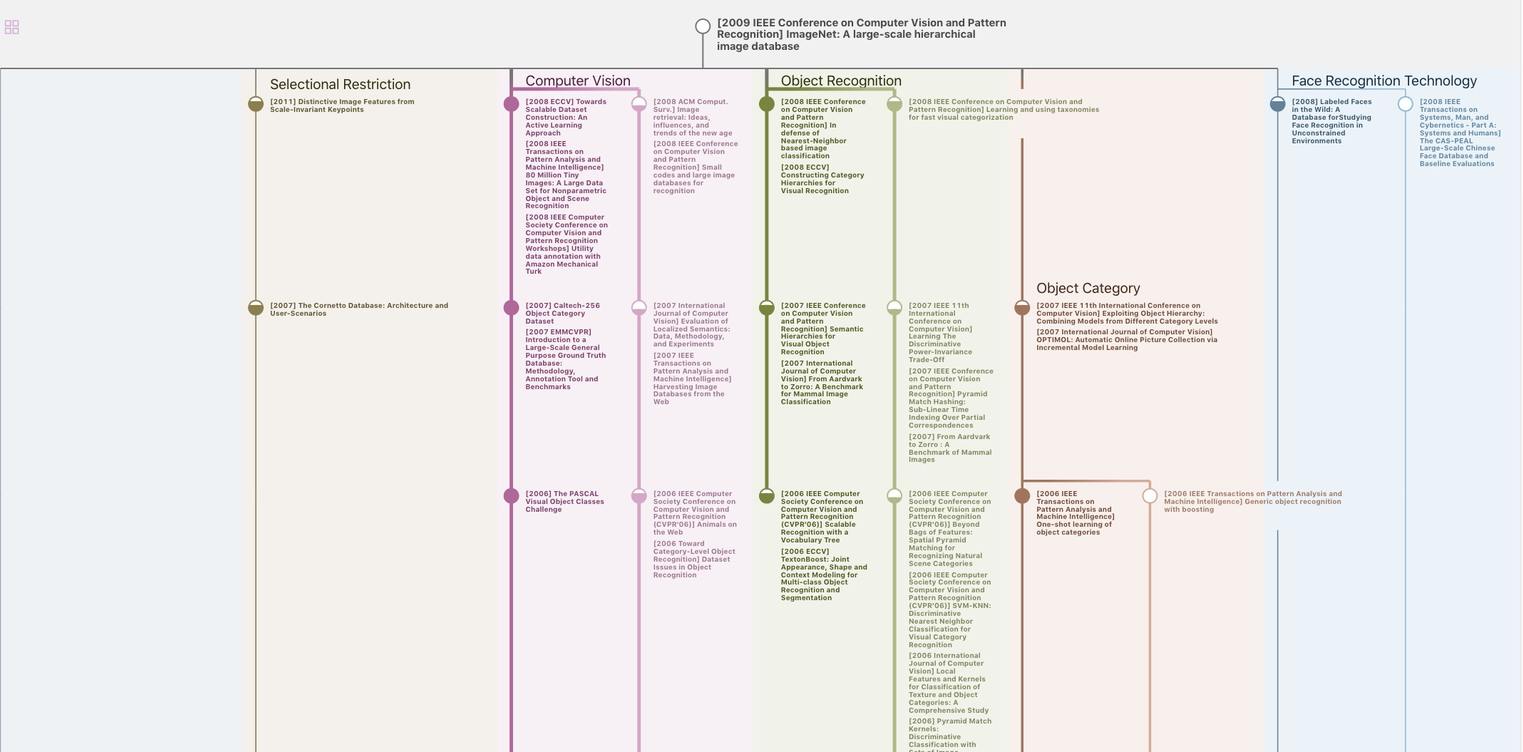
生成溯源树,研究论文发展脉络
Chat Paper
正在生成论文摘要