Machine learning assisted photothermal conversion efficiency prediction of anticancer photothermal agents
CHEMICAL ENGINEERING SCIENCE(2023)
摘要
Photothermal therapy (PTT) is a minimally invasive and promisingly effective strategy for thermal abla-tion of tumors. There is an urgent need for the development of ideal organic photothermal agents (PTAs) with high photothermal conversion efficiency (PCE). Machine learning (ML)-assisted predictions of PCE could offer an efficient way for early screening of PTAs. Herein, 44 organic PTAs were collected from the literature as a dataset to establish a best-performed regression model by comparing different ML methods, in which R2, Pears, and RMSE were 0.761, 0.913, and 0.058, respectively. Then, the reliability of the model was further verified by predicting two newly designed PTAs. The double bond of tetraphe-nylethylene (TPE) was found to be an important substructure to enhance PCE by the Shapley additive explanations method. The results show that ML can provide a valuable tool for predicting PCE of PTAs, thus promoting the development of photothermal therapy for cancer.(c) 2023 Elsevier Ltd. All rights reserved.
更多查看译文
关键词
Photothermal therapy,QSPR,Machine learning,Model interpretation,Feature extraction
AI 理解论文
溯源树
样例
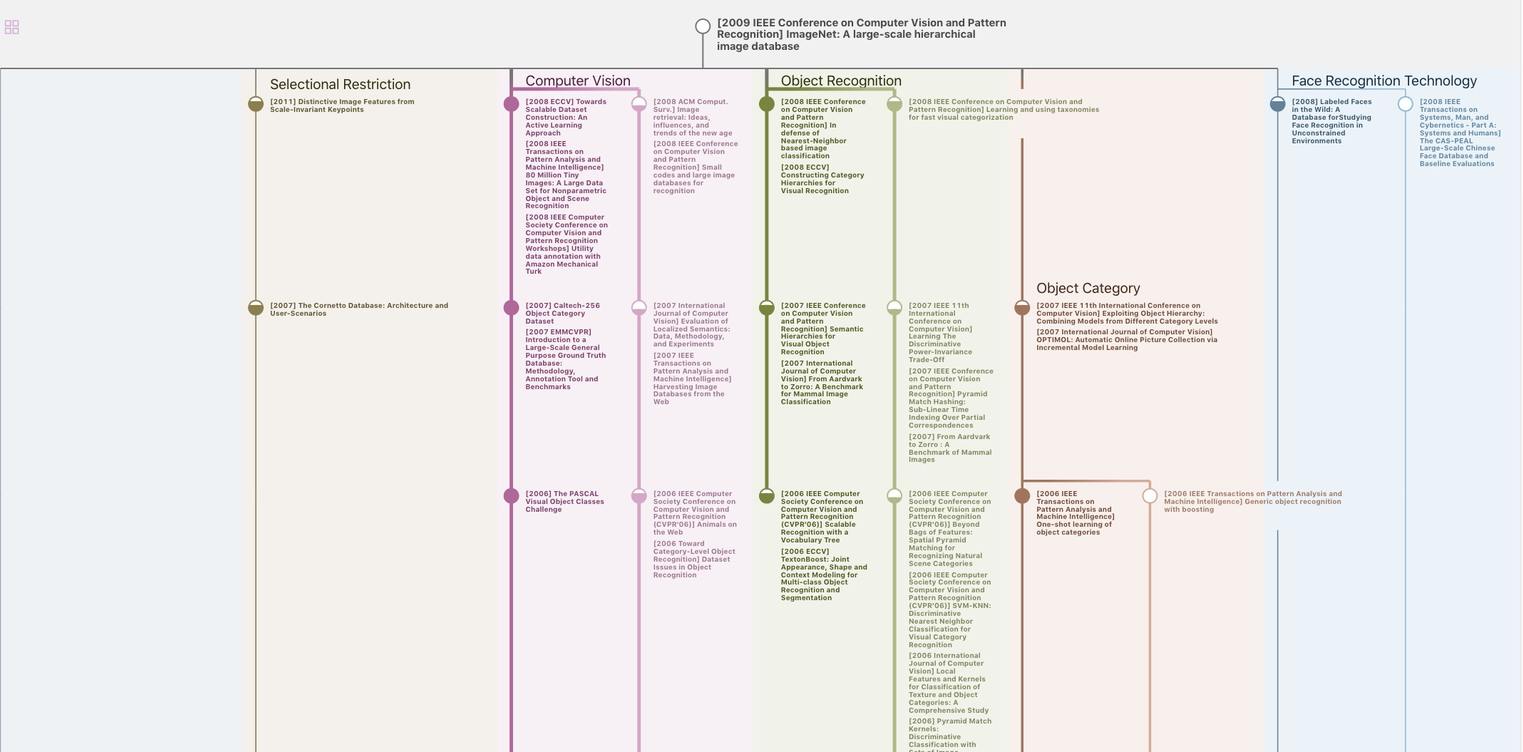
生成溯源树,研究论文发展脉络
Chat Paper
正在生成论文摘要