Pre-Activating Semantic Information for Image Aesthetic Assessment
AATCC JOURNAL OF RESEARCH(2023)
摘要
Automatic image aesthetic evaluation is an attractive and challenging visual task. Recently, methods based on convolutional neural networks have achieved remarkable performance. However, semantic information, an intuitive prerequisite for evaluating image aesthetics, has not received enough attention regarding its importance in previous methods. How to efficiently extract semantic information and make better use of it to assist the aesthetic evaluation task remains unsolved. In this article, we propose to utilize the self-supervised model Auto-Encoder to extract semantic information in the form of multi-task learning. Then, a fusing module is prepended at the bottleneck layer to explicitly combine semantic information with aesthetic information in a pre-activated manner. Specifically, we implement a customized pooling operation to pool the semantic features extracted by Auto-Encoder and apply a weak constraint between the pooled semantic features and aesthetic information to realize the combination. The following regressor can complete aesthetic evaluation based on the semantic-aesthetic combined features. In addition, to enable our model to adapt to arbitrary aspect ratios of images, another pooling strategy called spatial pyramid pooling is adopted to obtain the image features of a fixed length. Our method achieves competitive performance on the public image aesthetic evaluation benchmark. Especially on the most commonly used metric Spearman rank-order correlation coefficient, the proposed model achieved the best performance compared with some state-of-the-art methods. Extensive ablation studies and visualization experiments were conducted to demonstrate the effectiveness of our method.
更多查看译文
关键词
image aesthetic assessment,semantic,information,pre-activating
AI 理解论文
溯源树
样例
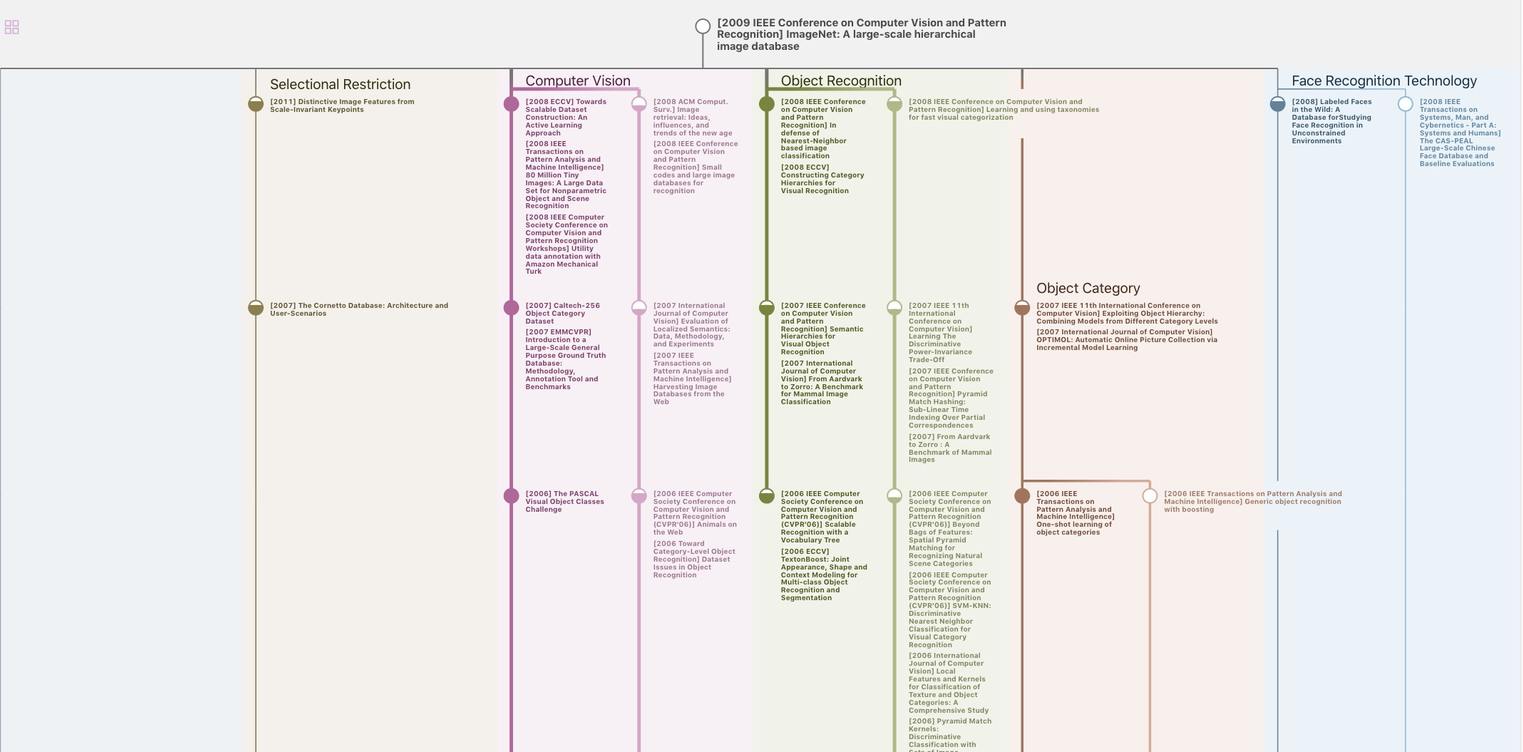
生成溯源树,研究论文发展脉络
Chat Paper
正在生成论文摘要