Fast, Approximate Maximum Likelihood Estimation of Log-Gaussian Cox Processes
JOURNAL OF COMPUTATIONAL AND GRAPHICAL STATISTICS(2023)
摘要
Log-Gaussian Cox processes (LGCPs) offer a framework for regression-style modeling of point patterns that can accommodate spatial latent effects. These latent effects can be used to account for missing predictors or other sources of clustering that could not be explained by a Poisson process. Fitting LGCP models can be challenging because the marginal likelihood does not have a closed form and it involves a high dimensional integral to account for the latent Gaussian field. We propose a novel methodology for fitting LGCP models that addresses these challenges using a combination of variational approximation and reduced rank interpolation. Additionally, we implement automatic differentiation to obtain exact gradient information, for computationally efficient optimization and to consider integral approximation using the Laplace method. We demonstrate the method's performance through both simulations and a real data application, with promising results in terms of computational speed and accuracy compared to that of existing approaches. for this article is available online.
更多查看译文
关键词
Automatic differentiation, Fixed rank kriging, Integrated nested Laplace approximation, Point patterns, Spatial point process, Variational approximation
AI 理解论文
溯源树
样例
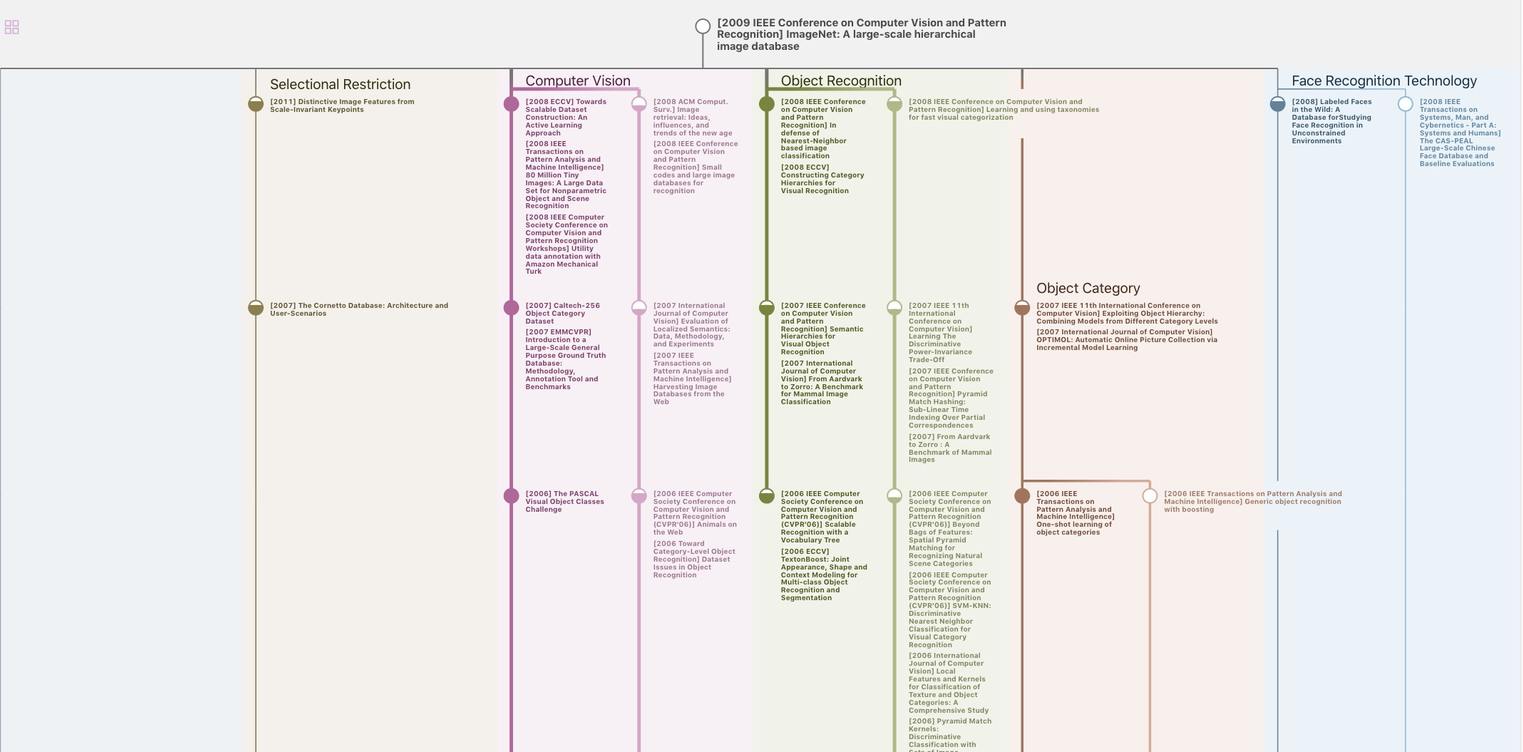
生成溯源树,研究论文发展脉络
Chat Paper
正在生成论文摘要