CP-nets-based user preference learning in automated negotiation through completion and correction
KNOWLEDGE AND INFORMATION SYSTEMS(2023)
Abstract
User preference learning is an important process in automated negotiation, because only when the negotiating agents are able to fully grasp the user preference information can the negotiation strategy play its due role. However, in most automated negotiation systems, user preference is assumed to be complete and correct, which is quite different from the reality. In real life, user preference is often complex and incomplete, which hinders the application of automated negotiation research in practice. To this end, this paper focuses on the learning method of user preference in negotiation. Since CP-nets can intuitively express the interdependence among negotiation issues, which have good interpretability and expansibility, they have become one of most important representations of user preference in automated negotiation. Therefore, we propose a CP-nets-based user preference learning module in negotiation framework, which consists of both passive learning and active learning methods. In passive learning, we propose an algorithm to construct complete CP-nets with incomplete user preference information. In active learning, we innovatively propose the structural query method, which improves the accuracy of preference learning represented by CP-nets with less query cost. The experimental results show that the module is effective for negotiation framework and can help users reach better agreements in negotiation.
MoreTranslated text
Key words
Automated negotiation,CP-nets,Preference learning,Incomplete information
AI Read Science
Must-Reading Tree
Example
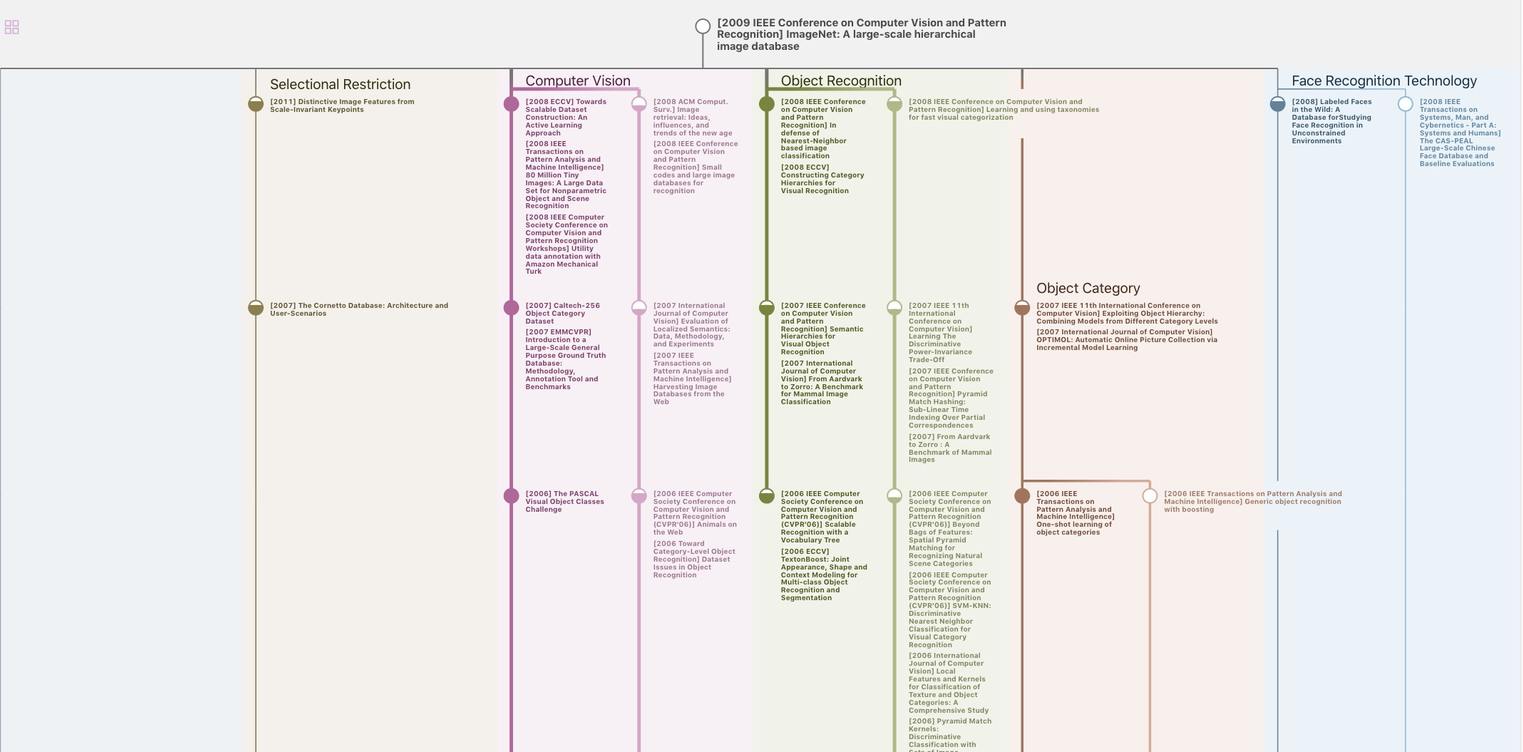
Generate MRT to find the research sequence of this paper
Chat Paper
Summary is being generated by the instructions you defined