Real-time robust and precise kernel learning for indoor localization under the internet of things
SIGNAL PROCESSING(2023)
摘要
More and more applications under Internet of Things have strong need for more dedicated localization techniques. As a wireless signal strength measurement standard, received signal strength indicator (RSSI) nowadays is widely utilized as a quantity to build advanced fingerprint indoor localization techniques. However, the mixed noise such as Gaussian noise together with the abrupt noise always causes the devi-ation of the RSSI value and the mismatched fingerprints in the fingerprint-based method, which results in the deterioration of positioning accuracy. In this paper, we propose an online risk-sensitive localiza-tion technique named compositional online kernel indoor localization (COKIL), which further improves the performance and reduces the prediction variance under multi-path effects. Meanwhile, the Student's t kernel is firstly employed in COKIL to fight against RSSI instability, which leads to the great perfor-mance improvement compared with the Gaussian kernel. Moreover, surprise criterion, novelty criterion and kernel orthogonal matching pursuit are embedded into COKIL to reduce the size of the neural net-works. Comparing their performances in experiments, surprise criterion is the optimal sparse method in practice. Finally, a new model-based technique, RSSIq, is proposed to deal with the missing fingerprints, which significantly improves the performance in indoor environment compared to traditional path-loss model.(c) 2023 Elsevier B.V. All rights reserved.
更多查看译文
关键词
Kernel learning,Stochastic optimization,Indoor localization,Internet of things
AI 理解论文
溯源树
样例
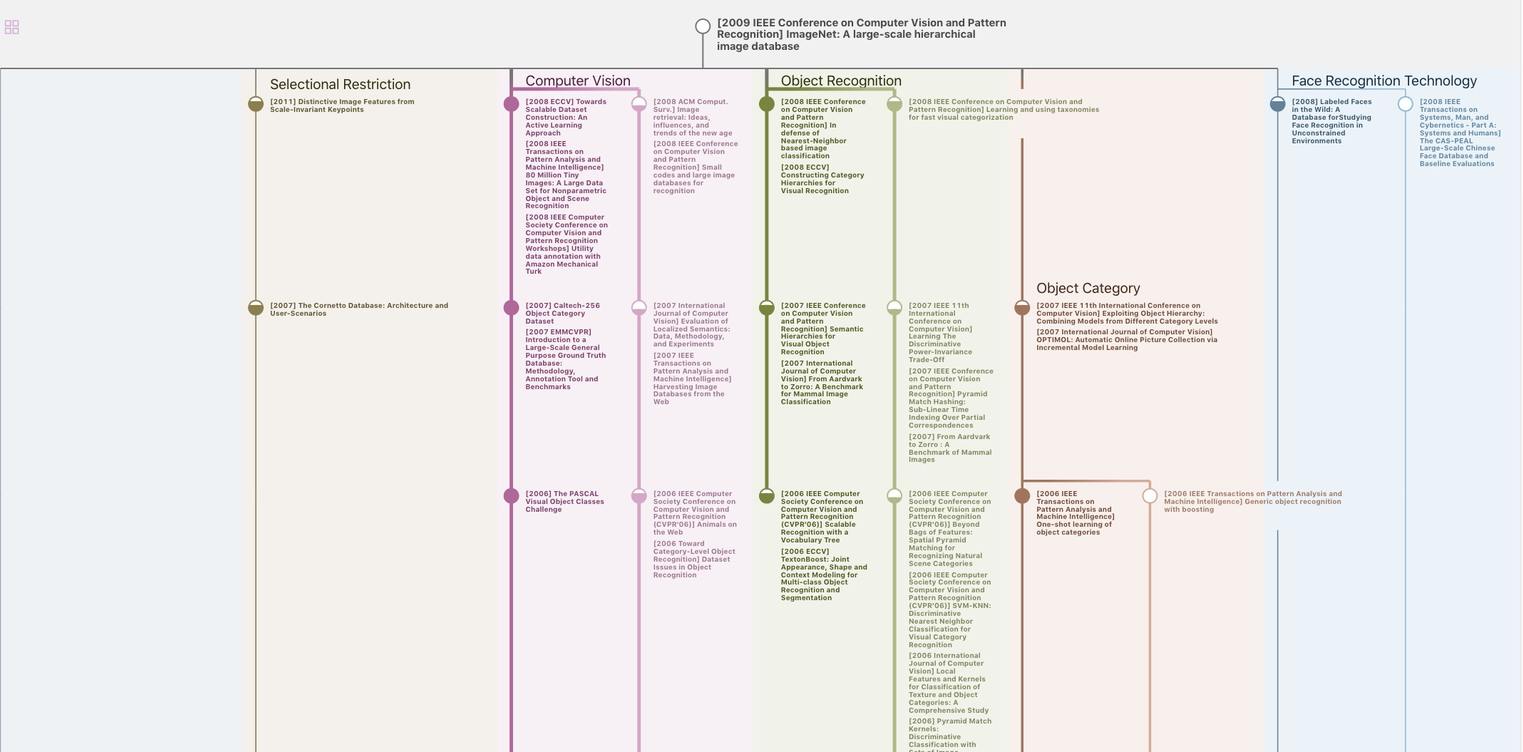
生成溯源树,研究论文发展脉络
Chat Paper
正在生成论文摘要