Investigation of Vowel Generation Method in Low-resource Pathological Voice Database
ENGINEERING LETTERS(2023)
Key words
pathological voice,data augmentation,improved WaveNet,SWT-WGANGP
AI Read Science
Must-Reading Tree
Example
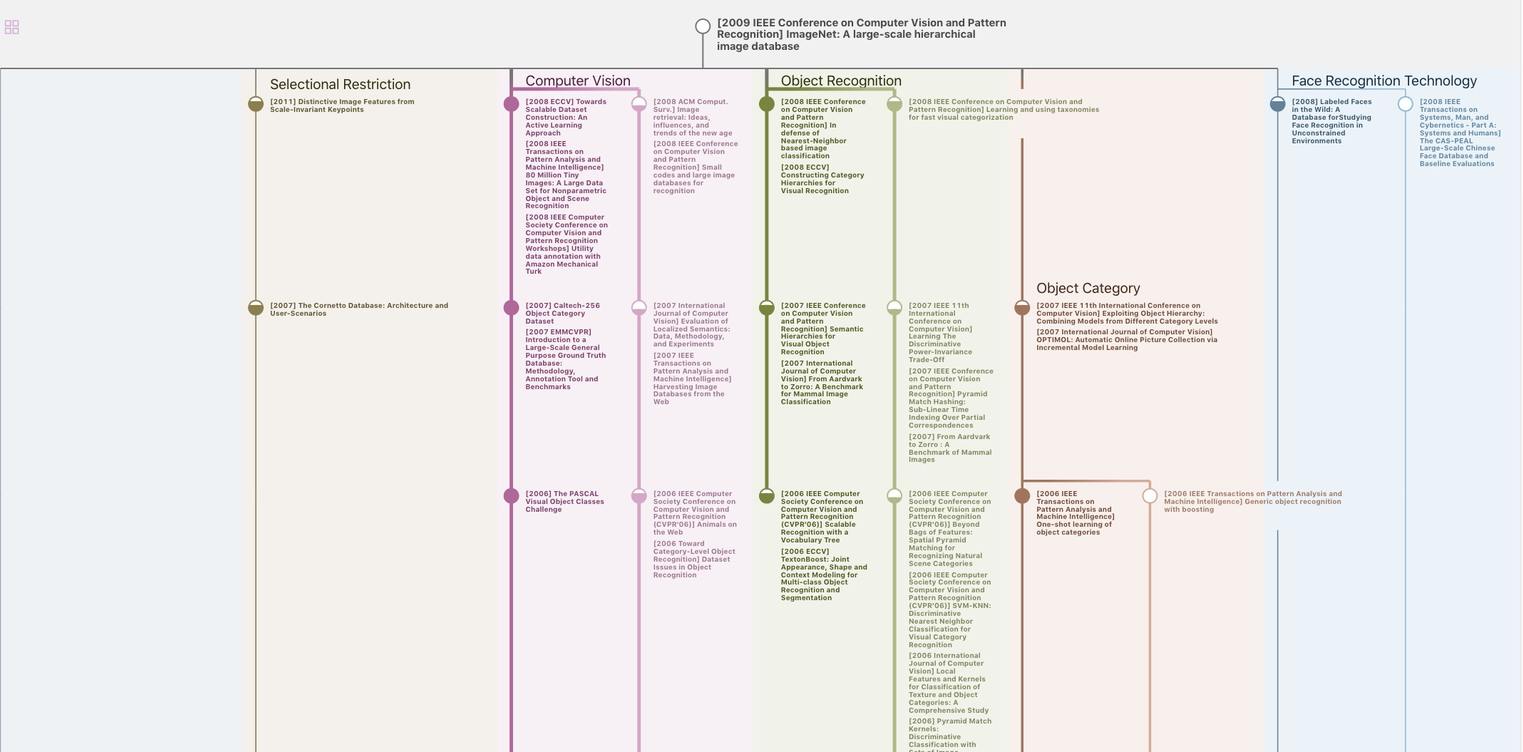
Generate MRT to find the research sequence of this paper
Chat Paper
Summary is being generated by the instructions you defined