Adaptive Grid Refinement Method for DOA Estimation via Sparse Bayesian Learning
IEEE JOURNAL OF OCEANIC ENGINEERING(2023)
摘要
In sparse signal recovery methods for direction of arrival (DOA) estimation, a set of uniform angular grid points is usually predefined. Dense grid points will improve the resolution and precision, but increase computational workload distinctly. To improve the efficiency and performance when using coarse initial grid points, an adaptive grid refinement (AGR) sparse Bayesian learning (SBL) method is proposed. The key idea of the proposed method is to adaptively insert new grid points based on the spatial spectrum learned from SBL iterations, as a result, grid points become denser and denser around the potential DOAs. The number of total grid points in the AGR process is much smaller than that of traditional uniform grid points, which enhances the computation efficiency. After the improved on-grid estimation of the AGR process, a post-processing DOA search procedure is implemented to reduce the off-grid DOA error. Furthermore, the proposed method is extended into the wideband case. Simulation results demonstrate that the proposed method has higher computation efficiency and precision than the classical off-grid SBL methods in scenarios of low SNR and limited snapshots. The effectiveness of the proposed method is also validated using the data of the SWellEx-96 ocean acoustic experiment.
更多查看译文
关键词
Direction-of-arrival estimation, Estimation, Bayes methods, Signal to noise ratio, Matching pursuit algorithms, Computational modeling, Wideband, Adaptive grid refinement, direction of arrival estimation, off-grid, sparse Bayesian learning, sparse signal recovery
AI 理解论文
溯源树
样例
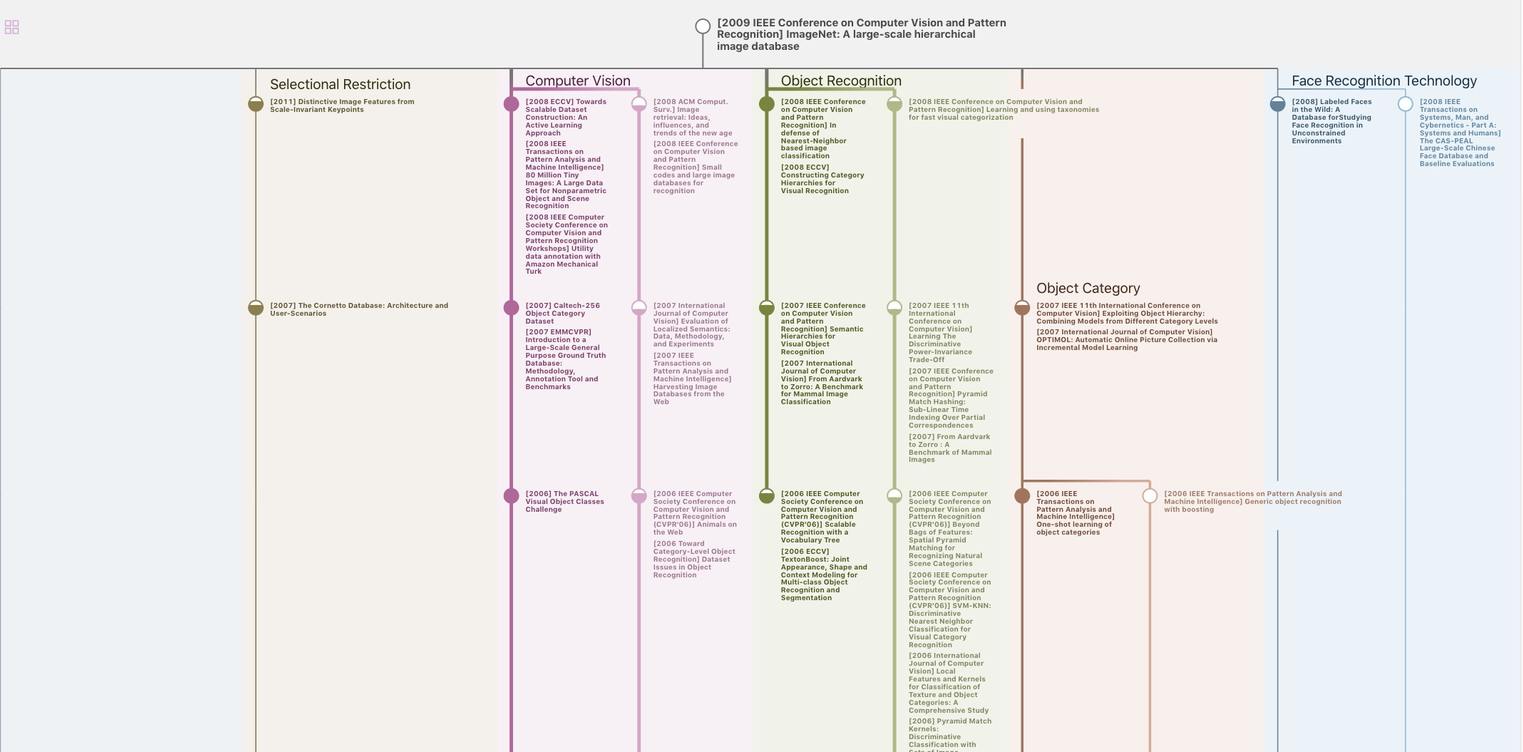
生成溯源树,研究论文发展脉络
Chat Paper
正在生成论文摘要