A secured and optimized deep recurrent neural network (DRNN) scheme for remote health monitoring system with edge computing
AUTOMATIKA(2023)
摘要
Patients now want a contemporary, advanced healthcare system that is faster and more individualized and that can keep up with their changing needs. An edge computing environment, in conjunction with 5G speeds and contemporary computing techniques, is the solution for the latency and energy efficiency criteria to be satisfied for a real-time collection and analysis of health data. The feature of optimum computing approaches, including encryption, authentication, and classification that are employed on the devices deployed in an edge-computing architecture, has been ignored by previous healthcare systems, which have concentrated on novel fog architecture and sensor kinds. To avoid this problem in this paper, an Optimized Deep Recurrent Neural Network (O-DRNN) model is used with a multitier secured architecture. Initially, the data obtained from the patient are sent to the healthcare server in edge computing and the processed data are stored in the cloud using the Elliptic Curve Key Agreement Scheme (ECKAS) security model. The data is pre-processed and optimal features are selected using the Particle Swarm Optimization (PSO) algorithm. O-DRNN algorithm hyper-parameters are optimized using Bayesian optimization for better diagnosis. The proposed work offers superior outcomes in terms of accuracy and encryption latency while using computational cloud services.
更多查看译文
关键词
deep recurrent neural network,remote health monitoring system,edge computing,drnn
AI 理解论文
溯源树
样例
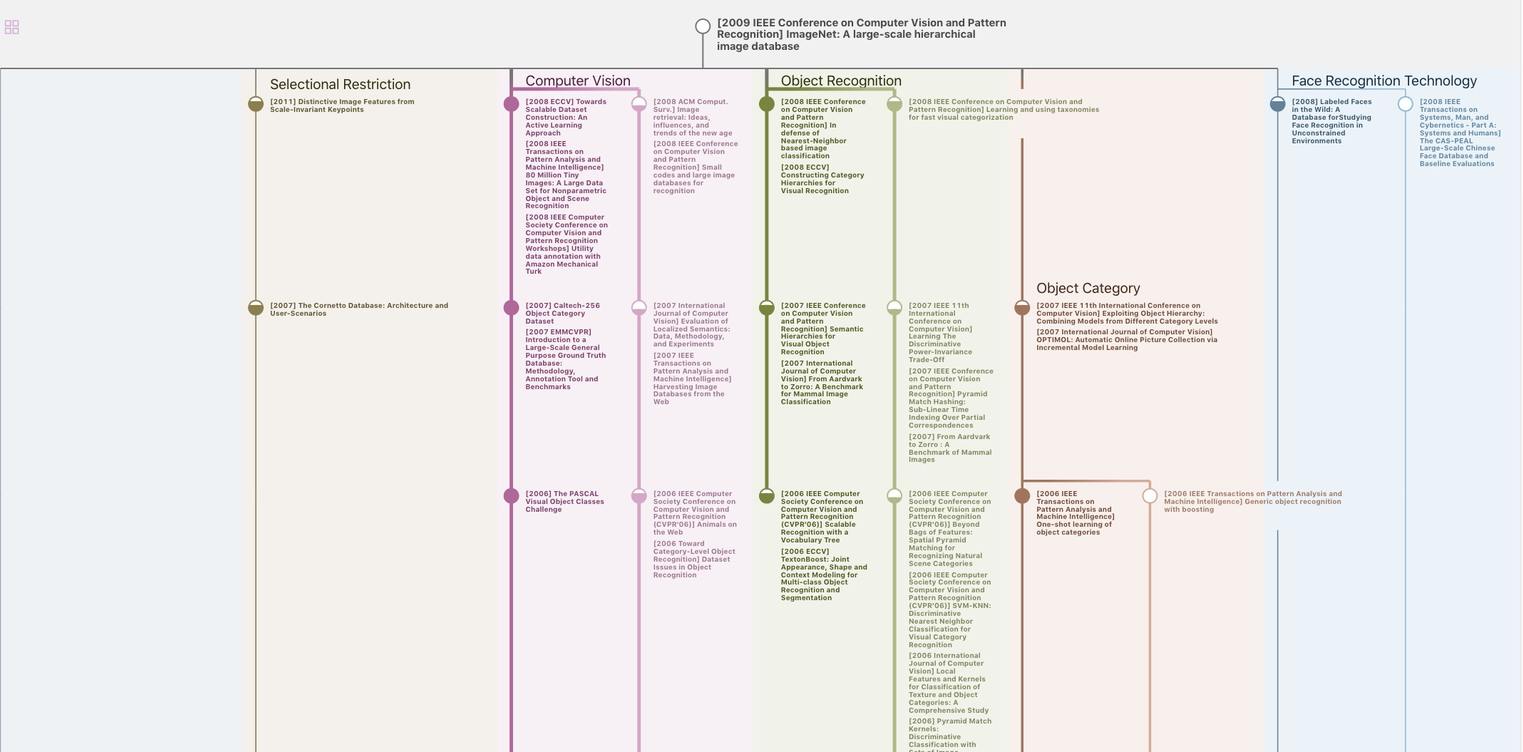
生成溯源树,研究论文发展脉络
Chat Paper
正在生成论文摘要