Toward Implementing Autonomous Adaptive Data Acquisition for Scanning Hyperspectral Imaging of Biological Systems
APPLIED PHYSICS REVIEWS(2023)
AI Read Science
Must-Reading Tree
Example
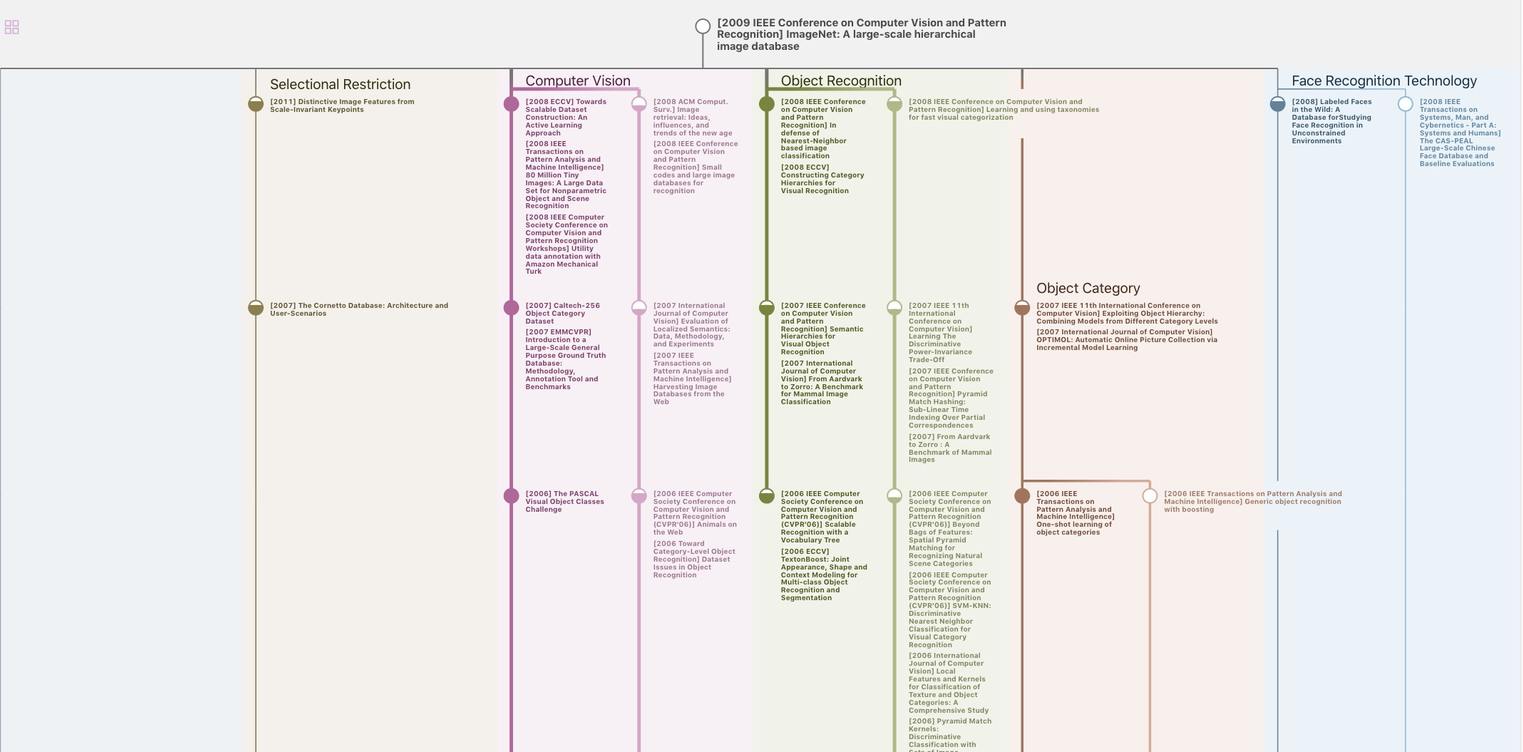
Generate MRT to find the research sequence of this paper
Chat Paper
Summary is being generated by the instructions you defined