Comparative Study for Daily Streamflow Simulation with Different Machine Learning Methods
WATER(2023)
摘要
Rainfall-runoff modeling has been of great importance for flood control and water resource management. However, the selection of hydrological models is challenging to obtain superior simulation performance especially with the rapid development of machine learning techniques. Three models under different categories of machine learning methods, including support vector regression (SVR), extreme gradient boosting (XGBoost), and the long-short term memory neural network (LSTM), were assessed for simulating daily runoff over a mountainous river catchment. The performances with different input scenarios were compared. Additionally, the joint multifractal spectra (JMS) method was implemented to evaluate the simulation performances during wet and dry seasons. The results show that: (1) LSTM always obtained a higher accuracy than XGBoost and SVR; (2) the impacts of the input variables were different for different machine learning methods, such as antecedent streamflow for XGBoost and rainfall for LSTM; (3) XGBoost showed a relatively high performance during dry seasons, and the classification of wet and dry seasons improved the simulation performance, especially for LSTM during dry seasons; (4) the JMS analysis indicated the advantages of a hybrid model combined with LSTM trained with wet-season data and XGBoost trained with dry-season data.
更多查看译文
关键词
LSTM,XGBoost,different input scenarios,joint multifractal spectra
AI 理解论文
溯源树
样例
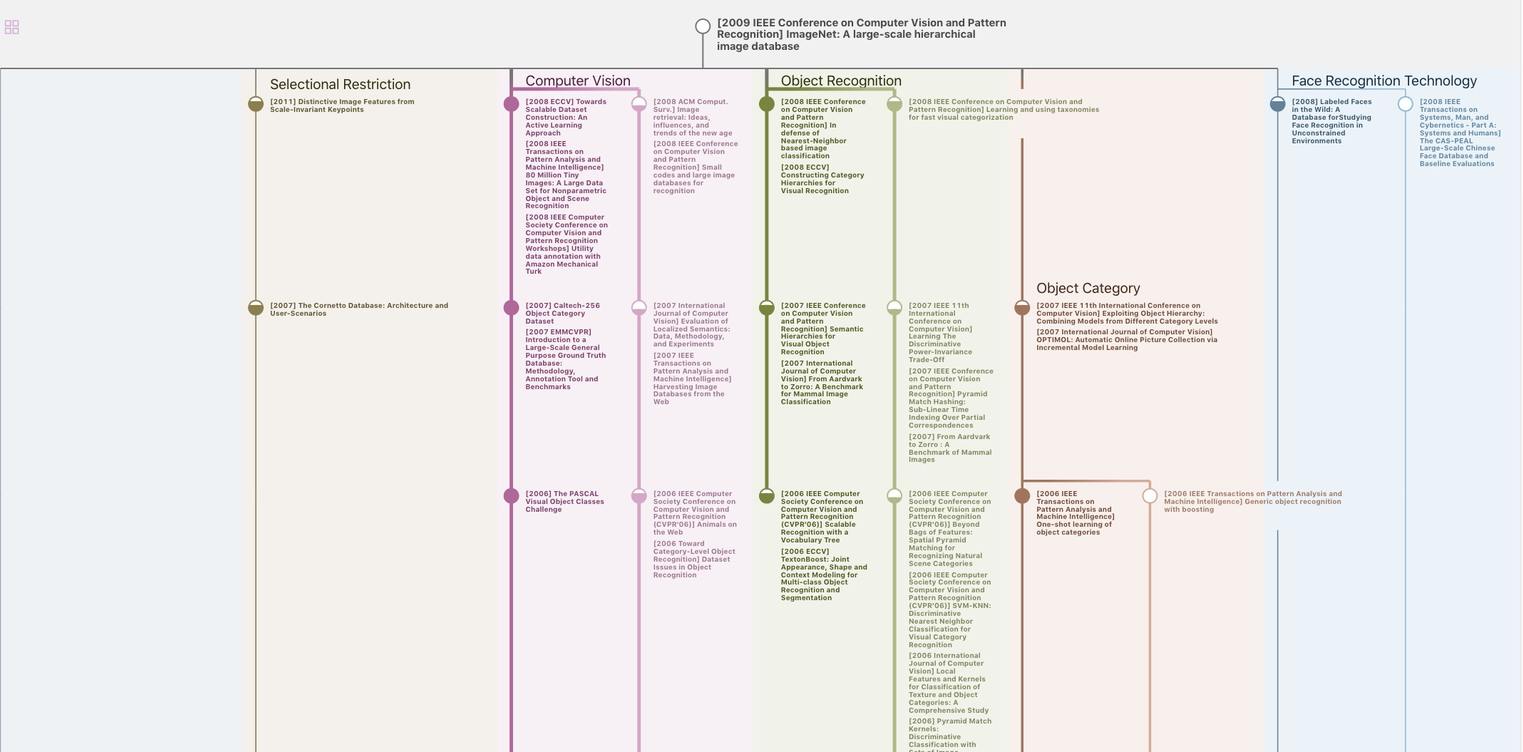
生成溯源树,研究论文发展脉络
Chat Paper
正在生成论文摘要