Model selection for varying coefficient nonparametric transformation model
ECONOMETRICS JOURNAL(2023)
摘要
Based on the smoothed partial rank (SPR) loss function, we propose a group LASSO penalized SPR estimator for the varying coefficient nonparametric transformation models, and derive its estimation and model selection consistencies. It not only selects important variables, but is also able to select between varying and constant coefficients. To deal with the computational challenges in the rank loss function, we develop a group forward and backward stagewise algorithm and establish its convergence property. An empirical application of a Boston housing dataset demonstrates the benefit of the proposed estimators. It allows us to capture the heterogeneous marginal effects of high-dimensional covariates and reduce model misspecification simultaneously that otherwise cannot be accomplished by existing approaches.
更多查看译文
关键词
Nonparametric regression,rank estimator,high dimensional modelling,varying coefficient
AI 理解论文
溯源树
样例
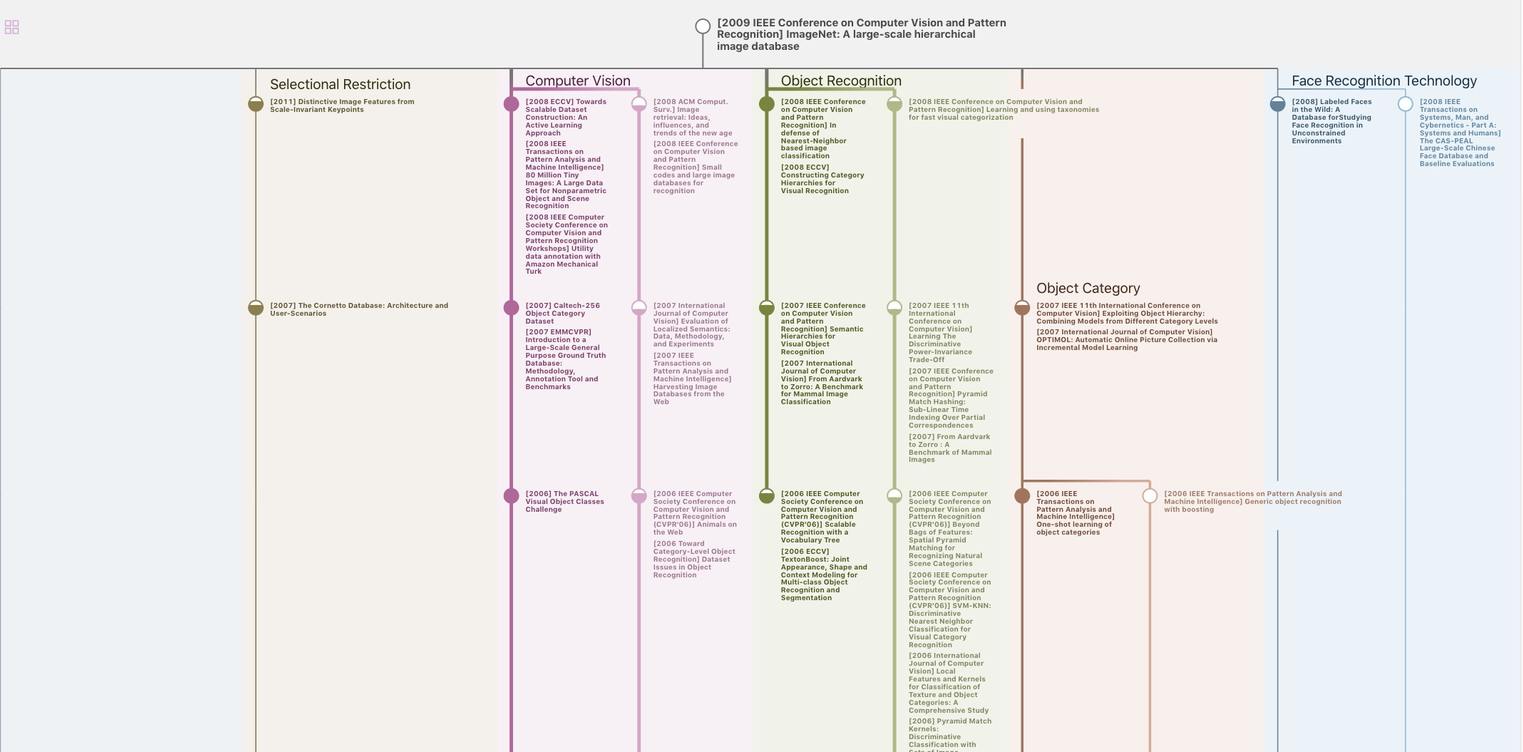
生成溯源树,研究论文发展脉络
Chat Paper
正在生成论文摘要